Paving the way for enriched metadata of linguistic linked data
Abstract
The need for reusable, interoperable, and interlinked linguistic resources in Natural Language Processing downstream tasks has been proved by the increasing efforts to develop standards and metadata suitable to represent several layers of information. Nevertheless, despite these efforts, the achievement of full compatibility for metadata in linguistic resource production is still far from being reached. Access to resources observing these standards is hindered either by (i) lack of or incomplete information, (ii) inconsistent ways of coding their metadata, and (iii) lack of maintenance. In this paper, we offer a quantitative and qualitative analysis of descriptive metadata and resources availability of two main metadata repositories: LOD Cloud and Annohub. Furthermore, we introduce a metadata enrichment, which aims at improving resource information, and a metadata alignment to META-SHARE ontology, suitable for easing the accessibility and interoperability of such resources.
1.Introduction
The need for reusable, interoperable and interlinked linguistic resources (LRs) in Natural Language Processing (NLP) downstream tasks has been proved by the increasing efforts to develop standardized representations and metadata schemes suitable to represent several layers of information. Nevertheless, despite these efforts, the achievement of a full compatibility for metadata in linguistic resource production is still far from being reached [11].
To overcome this limitation and to support a metadata harmonization process, several initiatives (e.g., LRE map11 [12], European Language Grid22 [48], CLARIN33 [35], Nexus Linguarum44 [22], Prêt-à-LLOD55 [23], Elexis66 [40]) have been proposed to promote community-based documentation and definitions of existing resources and standards. However, many challenges about resource discovery, reuse and integration are still present, due to the fact that issues of interoperability between different types of resources persist [14].
Lately, ontology-based approaches have become a wide-spread method for modelling linguistic data, mainly on the Semantic Web [5], as proven by the activities of the W3C Ontology-Lexicon community group77 to develop OntoLex-Lemon88, a shared vocabulary to represent lexical data and their linguistic information. As a consequence, several linguistic resources have been developed, in compliance with Linked Data (LD) principles99 and the number of datasets published as LD resources has increased at quite a fast pace (see Section 3).
Under the LD paradigm, data should comply to the aforementioned principles to ensure an easy discoverability, as well as an easy way to query information within the data [6,34]. The adoption of LD best practices assures that the structure and the semantics of the data are made explicit, which is also the main goal of the Semantic Web.
This goal of ensuring data transparency, reproducibility, and reusability is shared with the FAIR four foundational principles, namely findability, accessibility, interoperability, and reusability, that support producers and consumers to maximize the added-value of their data, algorithms, tools, etc., since all components of the research process must be available [59]. Nevertheless, descriptive metadata useful for retrieving and accessing such LD resources are still far away from being fully informative and interoperable, as they are not always up-to-date, shared and harmonized among providers and among repositories. Indeed, metadata used for describing an LD resource may be different, depending on the description schema applied and on the information provided by owners/creators as well as by repository maintainers. The heterogeneous nature of data sources may cause inconsistent as well as misinterpreted and incomplete metadata information [4].
Moreover, resources can become unavailable over time, as their landing pages or endpoints may change or be not accessible anymore. For example, within one of the main metadata repositories, i.e., LOD Cloud,1010 SentiWS,1111 a German-language resource for sentiment analysis, opinion mining, is not available, neither as the dump nor as the endpoint, even though the information that points to the dump1212 and to the SPARQL endpoint1313 does exist.1414
Thus, even though LD datasets are considered to be a gold mine as they can ease the access and interlink with other valuable interoperable resources, their usage is still limited, as finding useful datasets without prior knowledge is getting more complicated. In fact, in order to decide if the dataset is useful or not, one should have access to its descriptive metadata, where information about the content, such as its domain, access point, data dump or SPARQL endpoint, release and update dates, license information, etc., should be available. However, metadata do not always provide all this information, but they become fundamental for a first skimming. Dataset usage becomes even more challenging when the dataset does not come with metadata information at all, or when such information is partially missing. Access to reliable metadata is important for different use cases as they provide a landscape view, help with the dataset and ontology integration, and help with the data analysis.
Starting from these observations, we present a quantitative and qualitative analysis of the descriptive metadata and the resources availability from two main metadata repositories: Linked Open Data (LOD) Cloud and the Annotation Hub (Annohub).1515 Furthermore, we introduce a metadata enrichment effort, which aims at improving resource information, and a metadata alignment to a descriptive schema, namely META-SHARE ontology [29], in compliance with a minimal level of description suitable for easing the accessibility and interoperability of such resources (see Section 4).
With respect to the state-of-the-art, we make the following contributions:
– provide an analysis of the current status of linguistics resources: the domain that the resource belongs to, its language, type and license. Such analysis provides a general overview of the status of LOD and Annohub datasets.
– propose metadata alignment to META-SHARE ontology to harmonize the information within different repositories, and release the resulting RDF file;
– propose metadata enrichment for the existing information;
– evaluate the accessibility/availability of existing linguistic LD resources.
The remainder of this paper is organized as follows: Section 2 describes related work with reference to four lines of research, i.e., linguistic data cataloguing, quality evaluation, data enrichment, and metadata modeling. Following this, Section 3 presents the two main sources for LD and Section 4 introduces the methodology applied for both the metadata alignment and enrichment tasks. Section 5, provides resource and metadata analysis from two main perspectives, domains and languages covered, with the purpose of highlighting the effort of enriching the metadata. In Section 6, special attention is paid to linguistic resources, to the status of various languages in these repositories (i.e., low- or high-resourced), to their availability for interested parties, as well as to the type of license under which they are released. Finally, Section 7 presents conclusions and future work.
2.Background
In the recent years, many efforts have been made in order to link together the ever growing number of resources available on the Web. The literature on the topic of linking together linguistic resources, in particular, mainly focuses on the following lines of research: linguistic data cataloguing, quality evaluation, data enrichment and metadata modeling.
Linguistic Data Cataloguing: Cataloguing domain-specific LD generally calls for huge efforts, as usually the field for domain in datasets’ description is used ambiguously [44,53]. An example of such an effort is described in the creation of the AgroPortal repository1616 [38]. In particular, with regard to linguistic resources, in Chiarcos et al. [14] the authors apply LOD principles to linguistic data, with the objective of making such data queryable, interoperable and easy to share and expand through the Web.
The result is the Linguistic Linked Open Data Cloud1717 (LLOD Cloud), which makes use of many different vocabularies in its infrastructure, e.g LexInfo [16] and Lexvo [19], among others.
As an attempt to tackle two of the main shortfalls of the LLOD Cloud (i.e., the variations of language encoding standards and the lack of common metadata schemas for LD), Abromeit et al. [1] proposes Annohub, a dataset composed of languages and annotation schemes already used in language resources. In particular, the schemes are supported by and linked to the thesaurus of the Bibliography of Linguistic Literature1818 (BLL). Furthermore, this resource makes use of commonly adopted RDF vocabularies, such as DCAT,1919 Dublin Core,2020 DCMI Metadata Terms2121 and PROV.2222
Still, a high degree of heterogeneity in terms of representation formats is present among linguistic resources, as it is highlighted by Bosque et al. [8] in their reviewing of models and ontologies for language resources. In the present work, we attempt at tackling the heterogeneity and inconsistencies present in LRs metadata through a process of metadata alignment/mapping and further metadata enrichment (see Section 4).
Quality Evaluation: The evaluation of quality issues related to cataloguing LD has represented an important topic of discussion in recent years. This was, for example, one of the points highlighted during the creation of LODStats [26]. Research by Hasnain et al. [33] shows that, when working with data from the LOD Cloud through CKAN Datahub,2323 a tool helping to manage and publish collections of data, several issues may arise, especially in relation to ease of access to data. According to the authors, ownership related information was missing 41% of the cases, while 64% of the general metadata (e.g., size and url_type values) and 80% of the provenance information contained missing values.
Furthermore, many datasets contain unreachable/undefined URLs and inconsistent values in data fields.
Debattista et al. [21] performs a quality evaluation of several datasets from the LOD Cloud as a way to ease the search and processing of LD. The authors use the Dataset Quality Vocabulary [20] as a semantic quality metadata graph to make it possible for users to search, filter and rank datasets according to some quality criteria. The metrics used in this work, described by Zaveri et al. [60], deal with the assessment of data quality with regards to the following categories, each with its own set of quality dimensions:
– Accessibility Dimensions (availability, licensing, interlinking, security and performance);
– Intrinsic Dimensions (syntactic validity, semantic accuracy, consistency, conciseness and completeness);
– Contextual Dimensions (relevancy, trustworthiness, understandability and timeliness);
– Representational Dimensions (representational-conciseness, interoperability, interpretability and versatility).
The non-conformance to LOD guidelines is proven to be a problem in data quality as this is particularly common, especially in regards to certain pieces of information (e.g. Licensing and Human-Readable Metadata) [36]. For example, it is important to keep information updated about the status of accessibility of the resources. In this regard, SPARQLES2424 [54] focuses on the accessibility of SPARQL Endpoints registered in Datahub. As of March 2019, according to the collected data, the majority of endpoints falls into the lowest category for availability (342 out of 557), and the 64.72% of the endpoints have no metadata description.
Data Enrichment: One further topic of discussion tackles the need to solve the sparsity of information in LOD resources through data enrichment. Ding and Finin [24] show that, despite the ever growing size of the Semantic Web, the majority of properties used to describe data in natural language such as rdfs:comment, rdfs:label, dc:title, etc., are never used in the data. Similarly, the results of the cataloguing of Life Science Linked Open Data (LSLOD) [33] show that, for most datasets, there is little to no reuse of both ontologies and URIs. They use unpublished schemes resulting in high semantic heterogeneity [39]. These issues clearly pose serious problems in the attempts to align resources, which, even when dealing with a single domain, needs to be solved through an enrichment of the data.
Paulheim [47] describes three main axes to classify data refinement when dealing with concepts:
– completion (i.e., adding missing knowledge) vs error detection (i.e., the identification of wrong information);
– target of refinement;
– internal approach (i.e., using just the knowledge at hand) vs. external approach (i.e., making use of external human knowledge).
Despite the attempts, none of the approaches was able to correct and complete knowledge at the same time. In particular, what is highlighted is the absence of approaches that are able to find and correct errors at the same time. Furthermore, it is shown that most approaches focus on only one target (e.g. relations, literals, etc.). As mentioned already, in this work we try to overcome some of the above issues and solve inconsistencies in the description of resources and enrich metadata description for missing information: in particular we make use of both an automatic and a manual process of Metadata Enrichment to find and fix inconsistencies and missing values in the metadata (see Section 4).
Metadata Modeling: The efforts of cataloguing different datasets show the need for a uniform metadata model to improve the interoperability of data and facilitate retrieval processes and reuses of resources [17]. Many efforts have been made in recent years with regards to both general metadata, e.g. Vocabulary Of Interlinked Datasets (voiD) [3], Vocabulary of a Friend (VOAF),2525 Data Catalog Vocabulary (DCAT)2626 and DataID [9], and for more model- and domain-specific cataloguing, e.g. the Semantic Web Applications in Neuromedicine Ontology (SWAN)2727 by the Semantic Web Health Care and Life Sciences (HCLS) Interest Group;2828 the Linguistic Metadata (LIME) [28] for OntoLex,2929 the Meta-Share.owl ontology3030 [57], a linked open data version of the XML-based META-SHARE [29].
Other efforts to map META-SHARE to RDF have been carried out by the W3C Linked Data for language Technologies (LD4LT) community group,3131 as described by McCrae et al. [43] and Cimiano [18].
The basic needs answered by this model are:
– to identify and model all types of LRs and the relations occurring between them;
– to apply for a common terminology;
– to use minimal schemas that nevertheless allow for exhaustive descriptions;
– to guarantee interoperability between LRs, tools and repositories.
– expressiveness of LR typology in order to cover any type of resource;
– extensibility of the schemes through their modularity;
– semantic clarity of each element of the schema, which is thoroughly described;
– flexibility through the definition of a two tier schema which allows different levels of description;
– interoperability through the mappings to popular schemes (mainly Dublin Core).
In this model, the classification of LRs is also helped by the use of metadata elements (e.g., the value for lingualityType can be used as a means to distinguish between mono-, bi- and multilingual resources). The schema3232 for the META-SHARE model can describe LRs across several dimensions (see Section 4).
3.Repositories
As previously stated, our survey, conducted within the framework of Nexus Linguarum CA 18209,3333 is based on the information about resources available from two sources: the LOD Cloud and Annohub.3434 Neither of them is a place for storing resources, as they are both repositories of the metadata that describe such resources. Each repository has its own characteristics, making them suitable for contributing to our analysis of the state-of-the-art in LOD accessibility, interoperability and re-use, and to the development of a new resource based on a uniform metadata model. The main reason for choosing these repositories lies in the type of information they encompass. The LOD Cloud collects metadata for several resources on different domains, while Annohub contains only metadata about annotated linguistic resources from reliable sources.
3.1.LOD Cloud
The LOD Cloud is a diagram that offers an up-to-date image of the freely available linked datasets in various domains, maintained by the Insight Centre for Data Analytics.3535 The diagram uses different colours to render the datasets pertaining to nine domains (see Table 2), including a pool of cross-domain datasets. For every dataset in the cloud, the topic is either assigned by verifying its content or by accessing the metadata assigned by the publisher [53]. With 12 datasets in 2007, the LOD Cloud has grown constantly since then. In 2014, the Web was crawled by the LD Spider framework [49], which followed dataset interlinks.
The crawler seeds originate from three sources: (i) datasets from the LOD Cloud in datahub.io datasets catalog, as well as other datasets marked with Linked Data related tags within the same catalog; (ii) a sample from the Billion Triple Challenge 2012 dataset;3636 and (iii) datasets advertised since 2011 in the mailing list of public-lodw3.org. Since 2014, more frequent releases of the LOD Cloud have taken place. The cloud contains 1,440 datasets as of 20-05-2020.
As mentioned before, the LLOD cloud [14] was established in 2011 as a means “to measure and visualize the adoption of linked and open data within the linguistics community” [41]. It is the result of an effort by the Open Linguistics Working Group3737 and contains two kinds of resources: linguistic resources in a strict sense (e.g., dictionaries, wordnets, annotated corpora such as treebanks) and other linguistically-relevant resources (e.g., thesauri from tourism or life sciences, such as EARTh – the Environmental Applications Reference Thesaurus [2] or AGROVOC [13]); various downstream tasks can make use of them in data processing.
The diagram representing the LLOD Cloud is generated from the metadata in LingHub3838 [42], which is an indexing and search service that does not store metadata or resources, but provides harmonization of the metadata in different formats.
Although envisaged to reflect the linguistically-relevant resources available as linked data and with an open license, this is not fully observed at the moment (see also Section 6.2). McCrae et al. [41] write about a validation step when including resources in the LLOD Cloud. A new resource is included if: (i) its metadata contain a link to a resource already in the LLOD Cloud, and (ii) the resource is available for download.
The evolution of the number of resources in the cloud, presented by Chiarcos et al. [15], reveals a 19.3% increase every year since its establishment. The version of the LLOD Cloud considered for this survey contains 136 resources3939.
3.2.Annohub
Annohub [1] refers to both a software and a repository: the former queries various sources (including, e.g., LingHub, CLARIN and individual resource providers) of metadata of linguistic resources, while the latter stores the collected metadata. Annohub also comes with tools for resource type, language and annotation model detection from the resource content and represents all generated metadata as RDF.
Listing 1.
Query used to retrieve data from Annohub
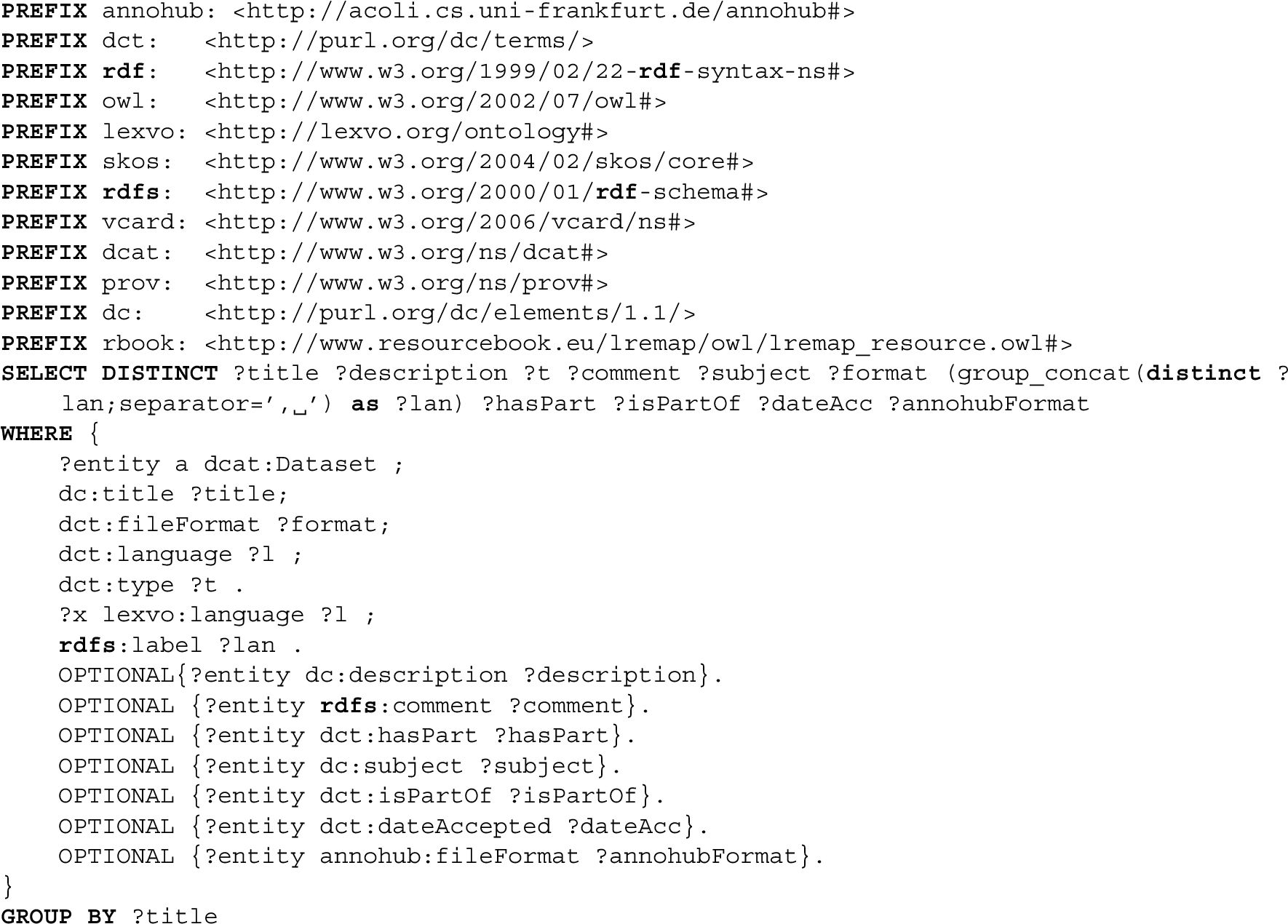
At the time of writing, the latest version of Annohub was from March 2020.4040 This version contains 604 resources with associated metadata. For the purposes of this work, data about these resources was retrieved using the query shown in Listing 1, then automatically enriched with information about the language and the adopted annotation model, manually validated, corrected and completed with missing information. We should add that, for the purpose of this paper, we count Universal Dependencies treebanks only once, irrespective of the fact that they are listed twice in the dump file, because they are available in two formats (i.e., RDF4141 and CONLL-U4242). After this, the remaining number of resources considered is 530.
4.Methodology
Information stored in the aforementioned metadata repositories has been used to analyse the existing resources and their metadata (see Section 5) and to develop a new enriched version of metadata information for LLD using META-SHARE ontology. For this purpose, we firstly gather resource information from both repositories, then fix inconsistencies and typos, align this information to the META-SHARE scheme and, finally, enrich the extracted information, both manually and automatically, to develop META-SHARE Enriched LLD (MELLD), a new metadata resource (see Fig. 1).
Fig. 1.
Methodology workflow.
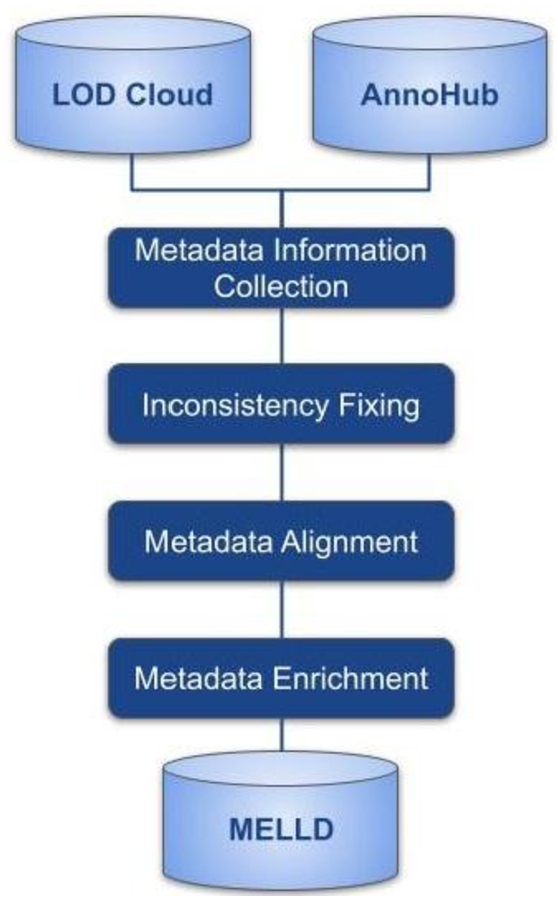
The LOD Cloud and AnnoHub have been developed for different aims and by means of different approaches, and so, they apply two different metadata schemes to collect information. This means that we would have different information also for the overlapping resources, i.e., according to our analysis there exist 69 overlapping datasets (see Section 5).
In fact, the LOD Cloud and Annohub collect different types of metadata with different levels of granularity.
Besides the differences noted in the metadata schemes, these two repositories apply two different approaches to the collection of resource information. Metadata information from the LOD Cloud is provided by the different resource providers/developers, while metadata information from Annohub are automatically generated from CLARIN, LingHub [42] resource metadata, or other reliable resource providers [1] so that they can be consistent and coherent.
Due to the fact that the LOD Cloud uses a bottom-up approach to collect the provided information, some metadata information may be missing (e.g., for some of the Universal Dependencies4343 [46] treebanks, such as for the Indonesian and the Czech ones, the language is not specified), or inconsistent, e.g., different names are used to refer to the same content. For instance, to indicate the language for resources on modern Greek, either Modern Greek (1453-) or simply Greek is used.
Furthermore, there also exist some inconsistencies within the LOD metadata themselves, e.g., linguistic and linguistics are used interchangeably to refer to resources belonging to the linguistic domain. The presence of this kind of inconsistencies would make it difficult for the potential user to retrieve all the resources from the respective domain when searching only for one of the two forms, in this case most probably the noun, i.e. linguistics, and would limit resource interlinking as well.
To harmonize and enrich the existing metadata, we adopt a two-step procedure, as follows:
1. Metadata alignment;
2. Metadata enrichment.
Then, we fix the inconsistencies among the values for several fields, e.g., domain, language, license type, and proceed to aligning manually such information to META-SHARE classes and properties, identified as core information with regards to usability and accessibility principles and considered useful for quality evaluation of both metadata and resources themselves. Finally, enrichment has been performed both automatically and manually with the aim of providing consistent values for the new metadata resource.
4.1.Metadata alignment
The alignment of the existing metadata information to the set of META-SHARE properties and classes has been performed manually starting from the analysis of the differences between the two metadata schemes (Listings 2 and 3).
Listing 2.
Example from LOD Cloud dumped data
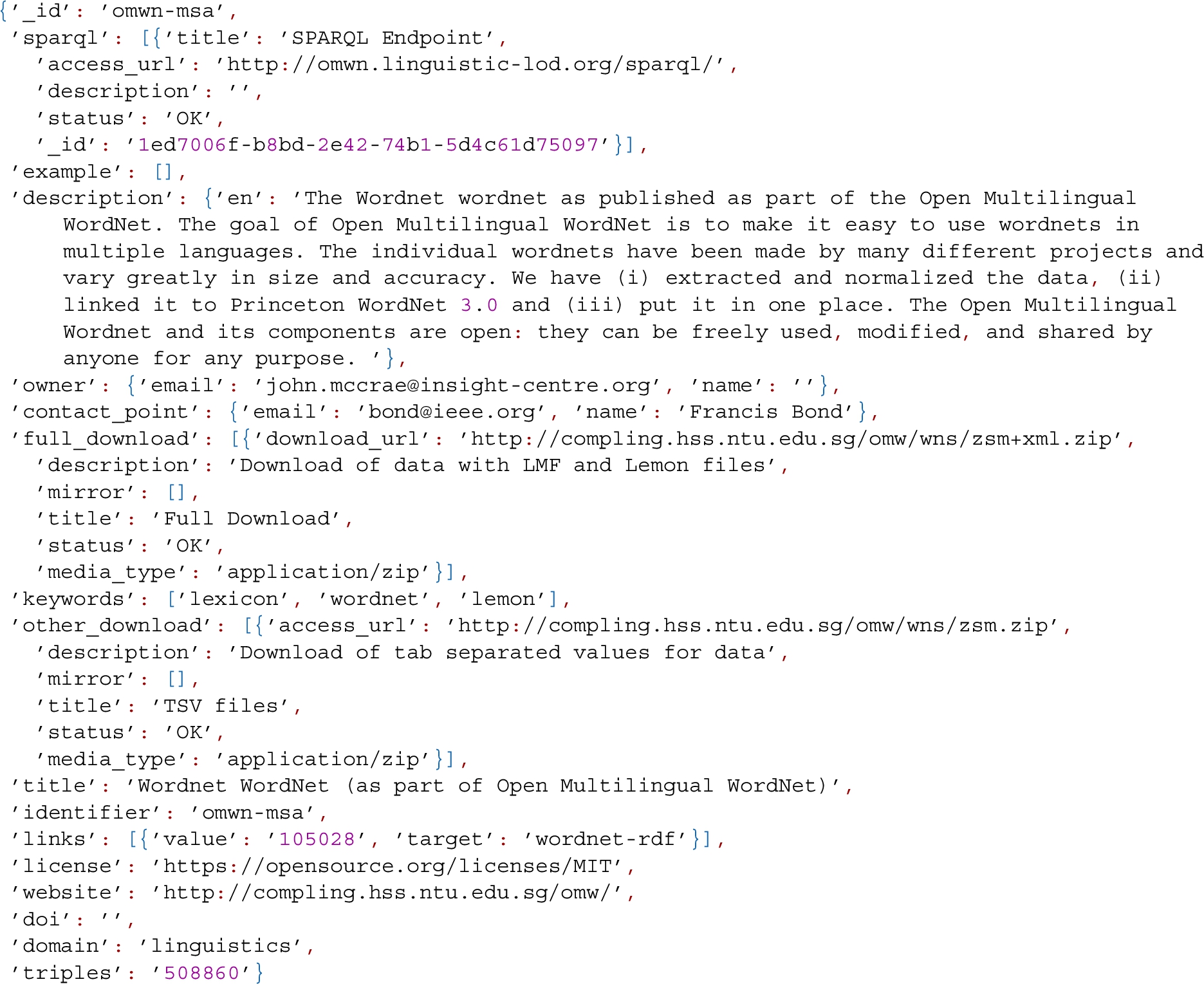
Listing 3.
Example from Annohub dumped data
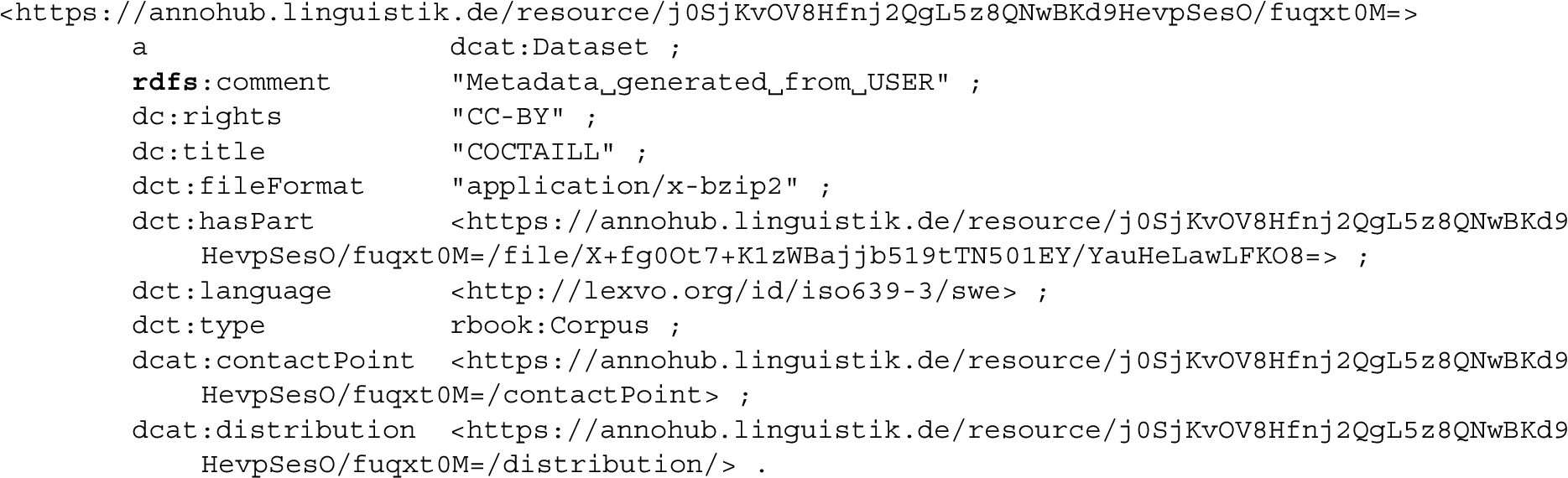
For instance, while the LOD Cloud has a field to indicate the domain and another one to specify keywords about the resources, the Annohub repository takes over the description/subject field from the original metadata provider, field which contains different information, e.g., models, annotation types. As far as the LOD Cloud metadata schema is concerned, a number of fields about available endpoints and downloads (e.g., SPARQL status, SPARQL link, Full download) and about provider/contact information (e.g., Owner, Contact point, Contact point email) are provided. On the other hand, Annohub metadata offer the possibility to include more fine-grained description about types, formats, languages and copyright information. Furthermore, while the LOD Cloud describes information about language using languages’ names, in Annohub this information is represented by a Lexvo URI [19].
In compliance with the LD principles for resource metadata, we select a set of properties and classes among the ones proposed by the META-SHARE ontology,4444 grouped according to the criterion they satisfy, as follows:4545
– Classification:
∗ metadataRecordIdentifier a string (e.g., PID, DOI, internal to an organization, etc.) used to uniquely identify a metadata record;
∗ resourceName to introduce a human-readable name or title by which the resource is known;
∗ resourceCreator links a resource to the person, group or organisation that has created the resource;
∗ language A particular linguistic system used in a specific region or by a social group;
∗ categoryLabel to introduce a human readable name (label) by which a classification category (e.g. text type, text genre, domain, etc.) is known;
∗ lcrSubclass to classify lexical/conceptual resources into types (used for descriptive reasons);
∗ size with reference to the number of triples. This value might be used for the indication of the size of the dataset so that a user might know in advance the amount of data (s)he has to deal with or prepare the right tools that might manage this amount of data;
∗ sizeUnit defining what size unit is used to describe the size property.
– Usability:
∗ landingPage links to a web page that provides additional information about a language resource (e.g., its contents, acknowledgements, link to the access location, etc.);
∗ licence to allow linking to a licence with a specific condition/term of use imposed for accessing a language resource. META-SHARE considers this as an optional element and only to be considered to provide brief human readable information on the fact that the language resource is provided under a specific set of conditions.
∗ dataFormat to indicate the format(s) of a data resource;
∗ description to report a short free-text account that provides information about the resource (e.g., function, contents, technical information, etc.);
∗ contact to report the data of the person/organization/group that can be contacted for information about a resource.
– Accessibility:
∗ downloadLocation A URL to the resource download page;
∗ accessLocation A URL to the SPARQL endpoint;
– Quality:
∗ annotationSchema to refer to the vocabulary/standard/best practice to which a resource is compliant with. It allows to link to the standard/model used for the creation of the resource;
∗ accessLocation/comment, downloadLocation/comment
∗ distributionForm to specify how the resource is distributed or how it can be accessed.
Furthermore, the classification of LRs is helped by the use of metadata elements (e.g., the value for lingualityType can be used as a means to distinguish between mono-, bi- and multilingual resources). To merge the existing metadata, we apply a manual alignment procedure between the information available in both repositories and the new metadata schema based on META-SHARE properties and classes (Table 1).
Table 1
Metadata alignment and enrichment. * indicates that the information from these fields has been enriched automatically and manually
META-SHARE | LOD Cloud | Annohub |
Classification | ||
metadataRecordIdentifier | identifier | N/A |
resourceName | title | title |
resourceCreator | owner* | creator |
language & lingualityType | keywords* | language(s) |
domain | domain | subject* |
lcrSubclass | name/keywords/description* | type |
size & sizeUnit | triples | N/A |
Usability | ||
landingPage | website | N/A |
licence | license | rights |
dataFormat | keywords* | description/subject* |
contact/email | contact_point_email | contact_point_hasEmail* |
contact/eName | contact_point_name | contact_point_name |
Accessibility | ||
downloadLocation | full_download*, other_download | accessURL* |
accessLocation | sparql_link | N/A |
Quality | ||
annotationSchema | keywords/description* | description* |
distributionLocation/comment | N/A | N/A |
accessLocation/comment | N/A | N/A |
distributionForm | sparql_status | N/A |
In addition to META-SHARE, we make use of properties from other ontologies to help with the final alignment and enrichment process. In particular:
– the property source from the Dublin Core Metadata Terms4646 is used to specify whether the resource comes from Annohub or LOD Cloud;
– the property sameAs from the OWL Web Ontology4747 is used to link together two resources that have both an URI from Annohub and one from LOD Cloud;
– the property hasIdentifier from DataCite ontology4848 is used to specify the ORCID for contacts.
Languages, agents and URLs are defined as separated resources in the final version of MELLD. In particular, languages are represented by a Lexvo URI, which is automatically built by appending the ISO 639-3 language code to the http://www.lexvo.org/id/iso639-3/ URL. For instance, the URI for the Italian language is http://www.lexvo.org/id/iso639-3/ita. A rdfs:label property is used to define a human-readable label for each specific language.
Agents (people and organizations) are defined using a custom-made URI, with further information being name, email,4949 and ORCID code, when possible.
Finally, URLs for accessLocation, downloadLocation and landingPage are represented as separate resources as well, with a comment property giving information on whether the website/SPARQL endpoint is accessible and working as intended.
4.2.Metadata enrichment
Finally, we proceed with a metadata enrichment phase, which has been achieved both automatically and manually. As most of the harvested information is already available in Annohub, the automatic enrichment was applied to the resources of the LOD cloud only, whereas the manual enrichment was applied to both LOD cloud and Annohub.
The automatic extraction procedure focuses on fields that encompass different types of information: language, domain, type, the values of creator and contact names, labels, and keywords.
For instance, from the names of the UD treebanks, e.g., Universal Dependencies Treebank Arabic, we easily extract information about their language and infer that, being a treebank, they are of type corpora. Such information might be inferred even for those resources lacking this information in the metadata, as in the case of the one for Indonesian and the one for Czech within the LOD Cloud (see Section 3 and Section 6). In addition to this, in some cases, when available, the resources’ content has also been considered, especially its textual properties. In particular, this automatic process has been carried out using a simple Python script5050 in order to recognize a list of keywords within the resource titles and descriptive texts, e.g., comment field. The list of keywords that the script recognizes is made up of: languages’ names, languages’ ISO codes (both extracted using the iso639 library).5151 Indeed, in several cases, the description and comment fields present more fine-graded information related to a resource, thus the enrichment of additional metadata results quite straightforward. For instance, considering the following example of a resource description from Annohub, we infer information about the annotation types and schemes (reported in bold face):
Universal Dependencies is a project that seeks to develop cross-linguistically consistent treebank annotation for many languages, with the goal of facilitating multilingual parser development, cross-lingual learning, and parsing research from a language typology perspective. The annotation scheme is based on (universal) Stanford dependencies (de Marneffe et al., 2006, 2008, 2014), Google universal part-of-speech tags (Petrov et al., 2012), and the Interset interlingua for morphosyntactic tagsets (Zeman, 2008). This dataset refers to UD’s Ancient_Greek treebank.
Information about ORCID was also automatically retrieved by querying creator names (or contact names in case the former were missing) on the ORCID API library for Python.5252
Then, a manual enrichment has been performed by three experts who filled in the information still missing after the automatic enrichment, so that language and domain values have been assigned to all the resources, including non-linguistic ones, while type has been assigned to linguistic resources only (see Section 6).
Moreover, a process of manual enrichment was further executed to retrieve missing information regarding resource accessibility and quality (Table 1).
On the basis of such a process of alignment and enrichment (see Section 5 and Section 6 for a comparison between original and enriched information), we propose a new coherent and consistent RDF-based metadata resource, aligned with a set of META-SHARE properties and classes, which encompasses enriched meta-data from both repositories.5353
5.Metadata overview
The LOD Cloud includes 1,447 unique datasets, with 13 of them repeating at least twice for a total of 1,461 entries in the dump.5454 But, as we mentioned already, its metadata is incomplete: it does not cover resource language and not all datasets have a domain explicitly assigned.
On the other hand, Annohub covers 530 resources, all with an assigned language (be it one or more, depending on the resource content) and all from the domain of linguistics. A total of 69 resources is present in both LOD Cloud and Annohub, which results in 1,908 distinct datasets considered.
Our analysis firstly focused on the domains and languages covered by the linked resources. Here, we look at the covered domains and the number of datasets for each, considering only the metadata from the LOD cloud and from Annohub, then using our enriched metadata (Enriched). Following the same methodology, we make a first analysis of the languages covered by the considered datasets.
5.1.Domain
Table 2 enumerates the domains covered by the LOD Cloud and the number of datasets per domain, in the original LOD metadata and in our enriched version. Whenever this field was empty, we tried to fill it in automatically or, when this was not possible, manually (see Section 4.2). Spahiu et al. [53] discuss the attempts to automatically classify datasets in the LOD into one or more domains. Even though sometimes datasets are considered as borderline between two ore more domains, in this paper we assume that each dataset belongs to a single domain. Even if, in some cases, this decision was not easy (e.g., linguistic resources, like corpora or thesauri, for a specific domain, such as GeoWordNet5555 [30]), we leave further refinements of this classification, including a review of the available domains, for future work.
Table 2
Number of datasets for each domain in the LOD Cloud metadata, Annohub and in the enriched metadata
Domain | LOD Cloud | Annohub | MELLD | |
Life Sciences | 344 | N/A | 343 | (−1) |
Government | 203 | N/A | 223 | (+20) |
Publications | 152 | N/A | 135 | (−17) |
Linguistics | 133 | 530 | 666 | (+72)* |
Cross Domain | 70 | N/A | 85 | (+15) |
User Generated | 70 | N/A | 67 | (−3) |
Social Networking | 54 | N/A | 54 | (=) |
Geography | 50 | N/A | 53 | (+3) |
Media | 39 | N/A | 38 | (−1) |
Metadata | N/A | N/A | 14 | (+14) |
Education | N/A | N/A | 6 | (+6) |
Art | N/A | N/A | 4 | (+4) |
Tourism | N/A | N/A | 2 | (+2) |
Other | N/A | N/A | 7 | (+7) |
Total w/ domain | 1,115 | 530 | 1,697 | (+121)* |
Total w/o domain | 332 | N/A | 211 | (−121)* |
Total | 1447 | 530 | 1908∗ |
LOD datasets span through nine domains with the most represented being Life Sciences. This covers the knowledge-rich biomedical domain, which has adopted Linked Data technologies, e.g., for representing medical ontologies, such as the Human Disease Ontology [50], or biology ontologies, such as the Plant Ontology [37]. In fact, a great contribution comes from the BioPortal5656 repository [58], where 245 datasets in the LOD Cloud are also indexed.
The second most represented domain is Government and the third is Publications. The former covers mainly Linked Data published by federal or local governments, including several statistical datasets [49]. Examples in this category include the data.gov.uk [51] and opendatacommunities.org datasets. The latter holds library datasets, information about scientific publications and conferences, reading lists from universities, and citation database. Prominent datasets in this category include German National Library5757 dataset, the L3S DBLP dataset5858 and the Open Library5959 dataset.
As far as this latter domain, Publications, is concerned, it is the only one for which the number of assigned resources has decreased in the enriched version, as a result of the fact that for a pool of resources we assigned a more appropriate domain, as well as of the fact that we considered that publications is hardly a domain [44]. Some examples of resources for which the domain field was changed from Publications to another value are: Project Gutenberg,6060 which was assigned the domain label cross_domain, already existing in the LOD Cloud; Linked Data from the Open University,6161 for which we assigned a new domain, namely education; data-szepmuveszeti-hu,6262 assigned to the new domain art; Santillana Guide Dataset,6363 assigned to the new domain tourism; or datos.bne.es, assigned to the new domain metadata.
In Table 2 we included the nine LOD Cloud domains and the newly assigned domains.
The linguistic domain comes only in fourth place and will be further analysed in Section 6. The number of datasets to which this domain was assigned is presented with an asterisk (*) because, as above, there are 69 datasets in both LOD Cloud and Annohub. So, this number cannot be obtained simply by subtracting the total of linguistic datasets in both repositories to the number of datasets belonging to this domain in the enriched data.
5.2.Language
In this section we focus on the language metadata field of all resources, irrespective of the domain they belong to, while the next section contains the discussion of this field only for the resources in the linguistic domain. One of the main issues, as mentioned already, is how repositories represent information about language. In Annohub, in fact, languages are represented by a Lexvo URI, while the LOD Cloud describes languages by names. In this work, we opt for the latter in order to harmonize the metadata at hand, for two main reasons: first, names are easier for humans to read than URIs; secondly, they are easier to retrieve automatically by the means described in Section 4 in case the information is not explicitly present.
Furthermore, we also noticed inconsistency among various resources. On one hand, there are cases when the language(s) of a resource is/are clearly mentioned in the metadata, while for others this information is missing. On the other hand, in the case of resources containing data in several languages, two ways of registering this are manifest: either the languages are enumerated (e.g., English, German, French, Spanish for JEL Classification6464) and they can sometimes be even in a high number (e.g., 262 languages are enumerated for lemon-UBY OmegaWiki English6565); or, instead, the label “multilingual” is used, but this is opaque as to the number of languages represented within the respective resource (see e.g., the resources BabelNet [45], Semantic Quran [52] or Open WordNet (as part of Open Multilingual WordNet) [7]).
Another type of inconsistency happens for some languages which can be referred to by means of different labels: e.g., the case of Modern Greek explained in Section 4. Another example is that of Norwegian, for which, in the case of some resources we know the variety (Bokmål or Nynorsk) as it is made explicit in the resource name (e.g., Apertium Dictionary Danish-Norwegian.Bokmål6666), but in the case of other resources only Norwegian is mentioned and it remains unclear if both varieties of the language are represented or only one (e.g. Freedict RDF dictionary Finnish-Norwegian6767). With the help of a native speaker, the Greek resources are now assigned the right information: either Ancient Greek (to 1453) or Modern Greek (1453-).
For the resources lacking information about the language, effort has been invested in filling this in and, as Tables 3 and 4 show, the metadata of many resources benefited from it. More precisely, while in the original metadata, only the 530 resources in Annohub had a language assigned, in the enriched metadata, we assign the language to 731 more resources, making a total of 1,261 resources with language (66%), out of which 666 are from the linguistic domain (100% for this domain). Table 3 shows the top-10 most represented languages in datasets indexed by the LOD Cloud and Annohub. For some multilingual resources we find a list of all the covered languages, either in the resource description or in a paper describing the resource. All the languages were added to the language field, split by commas, in a similar way to resources that already had several languages enumerated. Otherwise, if the resource was presented as multilingual and we did not find a list of the languages, we simply assigned it the label multilingual: this is the case for 4.4% of the resources. We found a total of 2,768 distinct values for the language field. As expected, English is the most represented language, by a far margin. Yet, out of the English resources, almost three quarters belong to non-linguistic domains, which becomes clearer if we compare these figures with Table 4, focused on the linguistics domain. This does not happen for most of the other languages and is explained by the fact that, in the manual assignment of languages, we considered the values of resource textual properties, namely names, labels, comments, or descriptions. It turns out that many datasets use English for labelling and commenting on their contents.
Table 3
Ten most represented languages and number of datasets covering each. Due to the multilingual resources, the total number of resources is different from the sum of resources per language
Language | Annohub | MELLD |
English | 99 | 577 |
Swedish | 278 | 288 |
Spanish | 50 | 105 |
German | 56 | 86 |
French | 56 | 80 |
Italian | 45 | 80 |
Czech | 28 | 69 |
Portuguese | 41 | 52 |
Polish | 44 | 50 |
Dutch | 38 | 48 |
Total w/ language | 530 | 1,261 (+731) |
Table 4
Languages covered by 30 or more datasets in the linguistic domain and their quantity in Annohub and in the enriched metadata
Language | Annohub | MELLD |
Swedish | 277 | 283 |
English | 99 | 146 |
Spanish | 50 | 66 |
German | 56 | 66 |
French | 56 | 66 |
Italian | 45 | 54 |
Portuguese | 41 | 48 |
Polish | 44 | 47 |
Dutch | 38 | 43 |
Catalan | 34 | 43 |
Finnish | 38 | 42 |
Russian | 36 | 38 |
Japanese | 34 | 38 |
Bulgarian | 30 | 33 |
Esperanto | 28 | 33 |
Modern Greek | 29 | 36 |
Turkish | 32 | 33 |
Latin | 31 | 31 |
Romanian | 27 | 31 |
Galician | 26 | 31 |
Czech | 28 | 30 |
Danish | 28 | 30 |
Total w/ language | 530 | 666 (+72)* |
6.Focusing on the linguistic domain
This section presents the status of the metadata resources in the linguistic domain. The important aspects are: (i) the language for which they were created, as this offers insights into the efforts made for ensuring a language presence in the electronic medium, on the one hand, and in the LD landscape, on the other hand, although we do not assume a direct correlation between these two aspects; (ii) the type of information they contain and the way in which this is annotated (when applicable), so in one word, the type of the resource, (iii) the licence with which they are released to the community, and (iv) the actual availability of the resource for those interested, which is scrutinized here from two perspectives: the possibility to download their data dump and/or to query them through a SPARQL endpoint.
6.1.Linguistic LD languages
When considering only the linguistic domain, the number of distinct languages is 2,766. Comparing it with the number of languages for which linked resources are indexed by the two repositories (see Section 5.2), we notice that there are only two languages, better, values in this field, for which LD resources exist but they either lack a domain or it is not linguistics. After inspecting these situations, we noted that they were: Bantu, which is actually a family of languages; and Swahili, for which there exist linguistics resources, but either marked as Swahili (individual language) or Swahili (macro language). Since we do not know where the latter would fit, we left it as Swahili only.
Table 4 shows the languages for which there are over 30 resources in the linguistic domain. It may come as a surprise that, considering only the linguistic domain, Swedish is the highest-resourced language, but this is justified by the fact that the second major source of resources for the Annohub repository is Sprakbanken.6868
When comparing the ranks of languages in Table 3 and in Table 4 we notice that, in general, most of the LD resources are in the linguistic domain, with English6969 (see also the discussion about multilingual resources in Section 5) and Czech7070 being exceptions.7171
Apart from Swedish and English, no other language has more than 100 linguistic datasets. Six languages have more than 50 linguistic datasets (Spanish, German, French and Italian). Interestingly enough, there are 382 languages with at least 10 linguistic datasets.
Table 5
Licenses that apply to more than one linguistic datasets and respective number of datasets
Type | LOD Cloud | Annohub | MELLD |
CC-BY | 36 | 241 | 289 (+12) |
GPL | 0 | 191 | 214 (+23) |
CC-BY-NC | 4 | 0 | 45 (+41) |
CC-BY-SA | 25 | 25 | 70 (+20) |
WordNet | 9 | 0 | 13 (+4) |
ODC-BY | 5 | 0 | 5 (=) |
CC-zero | 4 | 0 | 5 (+1) |
SUC | 0 | 0 | 2 (+2) |
Others | 8 | 3 | 16 (+5) |
N/A | 42 | 70 | 7 (−105) |
Total | 133 | 530 | 666 (+72)* |
6.2.License
The different types of licenses of the linguistic resources are presented in Table 5. We notice that they are all released with open access. A limitation is only imposed by the CC-BY-NC license that does not permit commercial use of the resources. However, it is used only for a rather small percent of datasets (7%).
Furthermore, despite the importance of specifying the type of license in order to improve shareability, a total of 41 linguistic resources in the original LOD Cloud and 70 resources in Annohub presented no values for the license of the data. The values for these licenses were manually enriched by using the information provided in the resources’ metadata. This way, values have been found for 104 datasets, with only 7 datasets without enough information on the website/documentation provided.
6.3.lcrSubclass
Despite using the lcrSubclass property from META-SHARE, we have ignored its values and borrowed the types in the LLOD cloud diagram, namely:
– corpora (defined as “collections of language data”),
– lexical-conceptual resources (“focus on the general meaning of words and the structure of semantic concepts”)
∗ lexicons and dictionaries,
∗ terminologies, thesauri and knowledge bases,
– metadata (“resources providing information about language and language resource”) [41].
∗ linguistic resource metadata (“linguistic resource metadata repositories, including bibliographical data”),
∗ linguistic data categories (“e.g., grammatical categories or language identifiers”),
∗ typological databases (“collections of features and inventories of individual languages, e.g., from linguistic typology”) [41].
– other resources.
All types written in italics in this classification are used in the LLOD Cloud for classifying resources7272 and, in order to ensure consistency, we used the same set for assigning a type to those resources lacking one. We recall that, in the enriched metadata, types were assigned to every single dataset of the domain linguistics.
Table 6 shows the counts of linguistic resources per type, in Annohub and in the enriched metadata. It is clear that most resources of this domain fall either in the type of corpora or of lexicons & dictionaries. 65 out of the 315 corpora are actually treebanks, almost all (except one) released within Universal Dependencies. When working with corpora, their levels of annotation represent important information, useful for, e.g., choosing one resource over another. In Annohub, the annotation model of the resources has been automatically inserted into the description.
Also, among the Lexicons and Dictionaries types, there are 42 wordnets, while ontologies are counted as Terminologies, Thesauri and Knowledge Bases type. Given the proven importance of such resources for NLP tasks, it could be important for a user to easily find and distinguish them from resources of the same type. At the moment, their name is the only way to distinguish them within the type they are assigned to. As it happens for the domains, in the future, types could benefit from a review.
Table 6
Number of linguistic datasets for each type, in Annohub and in the enriched metadata
Type | Annohub | MELLD |
Corpora | 312 | 315 (+3) |
Lexicons & Dictionaries | 218 | 303 (+85) |
Terminologies, Thesauri & Knowledge Bases | 0 | 30 (+30) |
Linguistic Data Categories | 0 | 12 (+12) |
Linguistic Resource Metadata | 0 | 4 (+4) |
Typological Databases | 0 | 2 (+2) |
Total | 530 | 666 (+136) |
The number of datasets for each language gives an overview on how languages are represented. A finer-grained perspective is given by looking at the types of resource available for each language. For this analysis, we focus on the 24 official languages of the European Union and present, in Table 7, not only the total number of linguistic datasets, but also the number of resources of the three most common types. For each of the previous, we show the total of resources including multilingual (All), counting once for each covered language, and also considering just monolingual datasets – i.e., dedicated exclusively to the target language (Mono). The latter is relevant because the coverage of different languages in some multilingual resources is significantly different. Moreover, they rarely focus on issues specific to one or a minority of languages.
Table 7
Linguistic resource types for each official EU language. ‘All’ includes multilingual resources and ‘Mono’ only resources exclusively dedicated to the target language
Language | All Linguistics | Corpora | Lexicons & Dictionaries | Terminologies Thesauri & KBs | ||||
All | Mono | All | Mono | All | Mono | All | Mono | |
Bulgarian | 33 | 2 | 3 | 1 | 30 | 1 | 0 | 0 |
Croatian | 28 | 2 | 3 | 1 | 25 | 1 | 0 | 0 |
Czech | 30 | 3 | 5 | 3 | 25 | 0 | 0 | 0 |
Danish | 30 | 2 | 1 | 1 | 28 | 1 | 1 | 0 |
Dutch | 43 | 3 | 5 | 2 | 36 | 1 | 2 | 0 |
English | 146 | 38 | 18 | 9 | 108 | 15 | 13 | 6 |
Estonian | 25 | 1 | 2 | 1 | 23 | 0 | 0 | 0 |
Finnish | 42 | 4 | 2 | 2 | 38 | 1 | 2 | 1 |
French | 66 | 4 | 7 | 2 | 55 | 2 | 4 | 0 |
German | 66 | 8 | 7 | 4 | 54 | 3 | 5 | 1 |
Modern Greek | 35 | 4 | 2 | 1 | 32 | 3 | 1 | 0 |
Hungarian | 26 | 1 | 2 | 1 | 24 | 0 | 0 | 0 |
Irish | 27 | 1 | 1 | 1 | 26 | 0 | 0 | 0 |
Italian | 54 | 5 | 4 | 2 | 49 | 3 | 1 | 0 |
Latvian | 24 | 1 | 1 | 1 | 23 | 0 | 0 | 0 |
Lithuanian | 26 | 0 | 1 | 0 | 25 | 0 | 0 | 0 |
Maltese | 22 | 0 | 0 | 0 | 22 | 0 | 0 | 0 |
Polish | 47 | 2 | 3 | 1 | 44 | 1 | 0 | 0 |
Portuguese | 48 | 4 | 4 | 3 | 43 | 1 | 1 | 0 |
Romanian | 31 | 2 | 3 | 1 | 27 | 1 | 1 | 0 |
Slovak | 27 | 2 | 3 | 1 | 24 | 1 | 0 | 0 |
Slovenian | 28 | 2 | 3 | 2 | 25 | 0 | 0 | 0 |
Spanish | 66 | 5 | 6 | 4 | 57 | 0 | 3 | 0 |
Swedish | 283 | 216 | 230 | 211 | 48 | 1 | 2 | 0 |
We note an imbalanced distribution of the three types, first explained by the fact that there are fewer datasets of the type Terminologies, Thesauri & Knowledge Bases, and when we look at monolingual resources, only English (6), Finnish (YSA – General Finnish Thesaurus7373) and German (Thesaurus Datenwissen7474) have one resource of this type. Yet, even though the total number of Corpora and Lexicons & Dictionaries is close (315 and 303, see Table 6), all languages but Swedish have significantly more datasets of the latter than of the former type. One reason for this is the fact that there are much more multilingual Lexicons & Dictionaries, including bilingual (e.g., Apertium) and multilingual dictionaries (e.g., DBnary RDF editions of Wiktionary, some of which covering hundreds of languages). We recall that these multilingual resources are counted once for each language represented. When looking at monolingual datasets, we also note that there is no corpus exclusively dedicated to Lithuanian and Maltese, and no lexicons or dictionaries for nine languages (Czech, Estonian, Hungarian, Irish, Latvian, Lithuanian, Maltese, Slovenian, Spanish).
Apart from the EU languages, other well-represented languages in the enriched metadata, with at least 30 linguistic datasets, include two languages spoken in Spain, namely Catalan (43 datasets, two monolingual) and Galician (31, 4), as well as Russian (38, 2), Japanese (38, 4), Turkish (33, 2), Esperanto (33, 1, though not a single corpus) and Latin (31, 3).
6.4.Resource accessibility
In this section we provide information about the accessibility of the dump, SPARQL endpoint, access via resolvable URIs and the ontology for the linguistics LOD datasets. Datasets from Annohub are considered to be available as their availability was checked in Spring 2019.
One of the main concerns about the availability and accessibility of LOD datasets is the fact that they become unavailable in time, which is considered as the main threat to the success of the Semantic Web [55]. Even though LOD Cloud reports resource unavailability by means of an alert signal, this information is not always correct. We find and download datasets for which LOD cloud assigned the alert and vice versa. For this reason, we checked the availability of all linguistic datasets manually. The availability for linguistic LOD datasets was inspected in August 2021.
There exist three ways to consume LLOD data: (i) download their data dump, (ii) query them by their SPARQL endpoint, and (iii) HTTP resolution of the resources URI present in the dataset. The SPARQL language is the standard query language proposed by the W3C7575 to query a collection of RDF triples [32]. Triple stores and RDF processing frameworks, such as Virtuoso [25], Jena [31], Eclipse RDF4J7676 or RDFLib7777 usually offer a SPARQL interface. Users are able to query the triples on the Web because of the SPARQL protocol [27]: clients submit SPARQL queries through a specific HTTP interface and the server executes these queries and responds with the results. Each client may submit unique and highly specific queries. This has as consequence requests timeout because of server overload. In such cases, HTTP caching mechanisms are ineffective as they can only optimize repeated identical queries. The architecture of SPARQL protocol demands the server to respond to highly complex requests, thus reliable public SPARQL endpoints are an exceptionally difficult challenge [56]. Such challenges contribute to the low availability of public SPARQL endpoints [10].
Another way to consume data is to access and download its dump. Data dump is a single-file that represent a part of or the entire dataset. It can contain some triples (e.g., reuters-128-nif-ner-corpus7878) up to billion triples (e.g., dbpedia-abstract-corpus7979). Because metadata about the data are often missing, consumers need to download the dump and make some exploratory queries. In such cases, consumers set up their own private SPARQL endpoint to host the data. However, as this resolves some issues, it has several drawbacks [56]: (i) setting up a SPARQL endpoint requires (possibly expensive) infrastructural support, (ii) involves (often manual) set-up and maintenance, (iii) the data are not up-to-date, and (iv) the entire dataset should be loaded in the server, even though just a part of it is needed.
The other well-known alternative to consume Linguistics Linked Data is through HTTP request to resources URI. The mechanism here requires dereferencing of URIs that describe entities in a dataset. Servers publish documents (“subjects page”) with triples about specific entities, while the client makes a request. The URI of an entity only points to the single document on the server that hosts the domain of that URI. Such documents contain also triples that mention URIs of other entities, which can be dereferenced in turn. This mechanism is a fundamental one, which allows to easily jump from one dataset to the other and access its data. It allows the creation of the LOD Cloud that represents interconnected datasets.
Table 8
Linguistic resource dump, SPARQL endpoint and ontology availability and accessibility
Original | Updated | |||
Accessibility | downloadLocation (of dump) | URL | 72 | 136 (+64) |
N/A | 64 | 0 (−64) | ||
Total | 136 | 136 (=) | ||
accessLocation (of SPARQL) | URL | N/A | 71 | |
unknow | N/A | 65 | ||
Total | N/A | 136 | ||
Access via resolvable URI | URL | 11 | 11 | |
N/A | 125 | 125 | ||
Total | 136 | 136 | ||
externalResource | URL | N/A | 40 | |
N/A | N/A | 96 | ||
Total | N/A | 136 | ||
Quality | accessibleThroughQuery (endpoint availability) | yes | 41 | 31 (−10) |
no | 3 | 40 (+37) | ||
N/A | 92 | 65 (−27) | ||
Total | 136 | 136(=) | ||
distributionLocation/comment (dump availability) | yes | N/A | 100 | |
no | N/A | 36 | ||
Total | N/A | 136 |
Table 8 summarizes the accessibility and availability (which we consider under the quality criteria) information about linguistic datasets (updated column). We include in this table also the statistics about the accessibility and availability of LLOD considering only the information in their metadata (original column). The accessibility information is provided for the dump (downloadLocation), SPARQL endpoint (accessLocation) and ontology (externalResource). From the original metadata we were able to find the information (URL) for 72 datasets, while 64 do not provide any information. We checked and updated the information about the URL to the resource download for all 136 datasets. LLOD metadata do not provide any information about the access to the SPARQL endpoint or about the accessibility and availability of the ontology. However, we were able to find the URL of the SPARQL endpoint for 71 datasets, while there is no information for 65 datasets. Regarding the accessibility of the ontology, we find such information for 40 datasets while we are missing it for 96.
The third way to consume Linked Data is through the access of resolvable URIs. This information is quite difficult to collect as (i) it is not available in the metadata, thus (ii) users should navigate to the homepage of the dataset and explore all the available pages, and (iii) often, such pages redirect to other sites (usually not in English) making it difficult to understand their content and to navigate properly. However, we were able to check the homepage of all the linguistic dataset and we find resolvable URIs for only 11 datasets.
The fact that datasets provide a link to the dump, endpoint of ontology does not mean that such information is actually available. Indeed, from 71 datasets that provide a link to the SPARQL endpoint, only for 31 this link is actually working. Only on three datasets (EMN, saldom-rdf and saldo-rdf) we were able to run only some specific example queries. The modelling of such datasets is out of the scope of this paper, thus we consider their SPARQL endpoint as available.
LOD metadata also contains the status of the SPARQL endpoint for each dataset with values such as “not available”, “available” or “empty”. Not available refers to the fact that the information about the endpoint is present but the endpoint is not available; available refers to the presence of the information about the endpoint in the metadata and the endpoint is actually available, and, finally, empty refers to the absence of such information. Within the metadata, 41 datasets provide the information about the SPARQL status as available, 3 as not available, while for most of them (92) this information is completely missing.
Only 22% of linguistic LOD datasets have a downloadable dump and an available SPARQL endpoint. The dump, SPARQL endpoint and ontology, is available only for 4 datasets (DBnary, PreMOn, getty-aat, rkb-explorer-wordnet).
Even though the LOD Cloud is considered a gold mine, its value is threatened by the unavailability of resources over time. As we can see from Table 8, only 70% of the linguistic datasets are available for download and only 30% of them are accessible through the SPARQL endpoint.
7.Conclusion and future work
In this paper, we present a preliminary investigation on linguistic LD resources and the metadata information used to represent them within the LOD Cloud and Annohub, together with MELLD, a new catalog of enriched information on LLD.
With reference to the assessment of existing metadata, as first results, we notice that LOD datasets span through nine domains with the most represented being Life Sciences, while the linguistic domain comes only in fourth place. With reference to languages, we noticed several inconsistencies among various resources, e.g., in the way such information is registered and in the use of different or inconsistent labels. When considering only the linguistic domain, the number of distinct languages is very high.
Further analysing LD in the linguistic domain, we observe that most resources fall either in the type of corpora or of lexicons & dictionaries and that they usually present an open license.
Finally, with reference to the accessibility of the data, the dump is available only for 70% of linguistics LOD datasets. With regard to the SPARQL accessibility, only 30% have a working endpoint.
As consequence of this recognition, in order to satisfy the accessibility and usability principles for LD resources, we propose MELLD, a new coherent and consistent metadata resource, aligned with a set of META-SHARE properties and classes, which encompasses enriched metadata from both repositories. Such an alignment could help quality assessment of resources and metadata, e.g., providing information about working accesses to those resources.
Future work includes a further metadata enrichment, with reference to the vocabularies and models applied in the development of such resources, together with a review of domains and types used to classify them. Moreover, the re-evaluation of the domain field is still in progress, and we expect to obtain more diverse, specific and reliable results.
Being aware that the manual enrichment is time-consuming, as for the metadata consistency check and the accessibility evaluation, we plan to implement a low-cost way to automatically achieve this task in order to guarantee also the maintenance of our catalog. One option is to further exploit the data already present in the database to fill in missing values.
In fact, other fields in the original metadata repositories might be used as a source of additional information for data enrichment. The process of automatization of these tasks would also help with the creation and implementation of a tool to convert a non-conforming resource description to one conforming to the META-SHARE model. This tool would give users the possibility to share their own resources regardless of their consistency with a metadata model, thus greatly improving intereoperability between linguistic datasets without the need for manual data refinement.
We also consider to support the distributed and collaborative creation and extension of LLOD by providing best practices to easily extend existing linguistic resources and publish their extensions as LD.
Another way to ensure the use of metadata in compliance with available standards could be creating a mechanisms for a validation of the information provided together with a resource.
Finally, we envision an analysis on the availability and the general status of metadata about other LOD datasets, in order to have a clearer picture and evaluate the potential of the LOD Cloud.
Notes
14 It is worth mentioning that the LOD Cloud reports resource unavailability by means of an alert signal. However, we found examples where, despite the alert, we could download the dataset, as well as the opposite.
32 The full documentation for Language Resources in the META-SHARE model can be found at http://www.meta-net.eu/meta-share/META-SHARE%20%20documentationUserManual.pdf.
34 It is worth stressing that Annohub includes metadata on language resources in different formats such as RDF, XML and CONLL, while LOD Cloud presents only metadata in RDF.
39 In the original metadata there were only 133 resources with linguistics domain assigned in the metadata, but as we assigned other three resources to the linguistics domain during the metadata enrichment phase, the total number of datasets raised to 136.
40 This version has since been archived at https://annohub.linguistik.de/archive/2020-03-30/.
44 http://www.meta-share.org/ontologies/meta-share/meta-share-ontology.owl/documentation/index-en.html
45 For the sake of this paper, we analyse deeply only some of the META-SHARE classes and properties in our enriched metadata resource. See Section 5 and Section 6.
49 It is worth stressing that some entries from the LOD Cloud present a unique email for resources with multiple authors. We leave the fix to this issue to future work.
50 While the code is not made publicly available for the purpose of this paper, it can be provided by directly contacting the authors.
51 https://pypi.org/project/iso-639/ and a series of manually collected keywords indicating domains and resource types (e.g., “corpora”, “terminology”, “lexicon”, and similar). By means of a set of rules based on regular expressions, we assign the retrieved keywords to their specific META-SHARE fields and infer some additional information (e.g., the domain “linguistics” can be inferred from the presence of the keyword “dependencies” in another field).
54 Please note that this json file contains 1,461 datasets while on the LOD website it is said that this version has 1,255 datasets https://lod-cloud.net/versions/2020-05-20/lod-data.json.
69 The largest set of English resources (i.e., 274) belong to the Life Sciences domain, and linguistics comes second.
70 There are 32 resources from the Government domain for Czech.
71 Spanish is another language for which 37% of the resources are not in the linguistic domain.
72 Such information is not present, however, in the LOD Cloud metadata and that is why this repository is not reflected by Table 6.
Acknowledgements
This work has been carried out within the COST Action CA 18209 European network for Web-centred linguistic data science (Nexus Linguarum).
Maria Pia di Buono has been supported by Programma Operativo Nazionale Ricerca e Innovazione 2014-2020 – Fondo Sociale Europeo, Azione I.2 “Attrazione e Mobilità Internazionale dei Ricercatori” Avviso D.D. n 407 del 27/02/2018.
Blerina Spahiu has been supported by FOODNET project (http://www.food-net.it/).
Hugo Gonçalo Oliveira was supported by national funds through FCT, within the scope of the project CISUC (UID/CEC/00326/2020) and by European Social Fund, through the Regional Operational Program Centro 2020.
The authors thank Penny Labropoulou for her help with the enrichment of the Greek resources with language information, and to Frank Abromeit for his help with all the information we needed about Annohub. We are also grateful for the valuable feedback we got from the reviewers, which contributed to improving the paper.
References
[1] | F. Abromeit, C. Fäth and L. Glaser, Annohub – annotation metadata for linked data applications, in: Proceedings of the 7th Workshop on Linked Data in Linguistics (LDL-2020) @LREC2020, (2020) , pp. 36–44. |
[2] | R. Albertoni, M. De Martino, S. Di Franco, V. De Santis and P. Plini, EARTh: An environmental application reference thesaurus in the linked open data cloud, Semantic Web 5: (2) ((2014) ), 165–171. doi:10.3233/SW-130122. |
[3] | K. Alexander, L. Talis Cyganiak, M. Hausenblas and J. Zhao, Describing linked datasets-on the design and usage of voiD, the ‘Vocabulary of interlinked datasets’, Linked Data on the Web Workshop (LDOW 09), in conjunction with 18th International World Wide Web Conference (WWW 09) 538, 2010. |
[4] | A. Assaf, A. Senart and R. Troncy, Roomba: Automatic validation, correction and generation of dataset metadata, in: Proceedings of the 24th International Conference on World Wide Web, (2015) , pp. 159–162. doi:10.1145/2740908.2742827. |
[5] | T. Berners-Lee, J. Hendler and O. Lassila, The semantic web, Scientific American 285: (5) ((2001) ), 34–43. |
[6] | C. Bizer, T. Heath and T. Berners-Lee, Linked data: The story so far, in: Semantic Services, Interoperability and Web Applications: Emerging Concepts, IGI Global, (2011) , pp. 205–227. doi:10.4018/jswis.2009081901. |
[7] | F. Bond and R. Foster, Linking and extending an open multilingual wordnet, in: Proceedings of the 51st Annual Meeting of the Association for Computational Linguistics (Volume 1: Long Papers), (2013) , pp. 1352–1362. |
[8] | J. Bosque-Gil, J. Gracia, E. Montiel-Ponsoda and A. Gómez-Pérez, Models to represent linguistic linked data, Natural Language Engineering 24: (6) ((2018) ), 811–859. doi:10.1017/S1351324918000347. |
[9] | M. Brümmer, C. Neto, I. Ermilov, M. Freudenberg, D. Kontokostas and S. Hellmann, Data ID: Towards semantically rich metadata for complex datasets, ACM International Conference Proceeding Series 2014: ((2014) ), 84–91. ISBN 978-1-4503-2927-9. doi:10.1145/2660517.2660538. |
[10] | C. Buil-Aranda, A. Hogan, J. Umbrich and P.-Y. Vandenbussche, SPARQL web-querying infrastructure: Ready for action? in: International Semantic Web Conference, Springer, (2013) , pp. 277–293. doi:10.1007/978-3-642-41338-4_18. |
[11] | N. Calzolari, N. Bel, K. Choukri, J. Mariani, M. Monachini, J. Odijk, S. Piperidis, V. Quochi and C. Soria, Final FLaReNet deliverable: Language resources for the future – the future of language resources, The Strategic Language Resource Agenda. FLaReNet project, 2011. |
[12] | N. Calzolari, R. Del Gratta, G. Francopoulo, J. Mariani, F. Rubino, I. Russo and C. Soria, The LRE map. Harmonising community descriptions of resources, in: Proceedings of the Eighth International Conference on Language Resources and Evaluation (LREC’12), European Language Resources Association (ELRA), Istanbul, Turkey, (2012) , pp. 1084–1089, http://www.lrec-conf.org/proceedings/lrec2012/pdf/769_Paper.pdf. |
[13] | C. Caracciolo, A. Stellato, A. Morshed, G. Johannsen, S. Rajbahndari, Y. Jaques and J. Keizer, The AGROVOC linked dataset, Semantic Web 4: (3) ((2013) ), 341–348. doi:10.3233/SW-130106. |
[14] | C. Chiarcos, P. Cimiano, T. Declerck and J.P. McCrae, Linguistic linked open data (llod). Introduction and overview, in: Proceedings of the 2nd Workshop on Linked Data in Linguistics (LDL-2013): Representing and Linking Lexicons, Terminologies and Other Language Data, (2013) , pp. i–xi. |
[15] | C. Chiarcos, B. Klimek, C. Fäth, T. Declerck and J.P. McCrae, On the linguistic linked open data infrastructure, in: Proceedings of the 1st International Workshop on Language Technology Platforms (IWLTP 2020), (2020) , pp. 8–15. |
[16] | P. Cimiano, P. Buitelaar, J. McCrae and M. Sintek, LexInfo: A declarative model for the lexicon-ontology interface, Journal of Web Semantics 9: (1) ((2011) ), 29–51, https://www.sciencedirect.com/science/article/pii/S1570826810000892. doi:10.1016/j.websem.2010.11.001. |
[17] | P. Cimiano, C. Chiarcos, J.P. McCrae and J. Gracia, Linguistic Linked Data – Representation, Generation and Applications, Springer, (2020) . doi:10.1007/978-3-030-30225-2. |
[18] | P. Cimiano, C. Chiarcos, J.P. McCrae and J. Gracia, Modelling metadata of language resources, in: Linguistic Linked Data, Springer, (2020) , pp. 123–135. doi:10.1007/978-3-030-30225-2_7. |
[19] | G. de Melo, Lexvo.org: Language-related information for the linguistic linked data cloud, Semantic Web 6: (4) ((2015) ), 393–400. doi:10.3233/SW-150171. |
[20] | J. Debattista, C. Lange and S. Auer, Representing dataset quality metadata using multi-dimensional views, in: Proceedings of the 10th International Conference on Semantic Systems, (2014) , pp. 92–99. doi:10.1145/2660517.2660525. |
[21] | J. Debattista, C. Lange, S. Auer and D. Cortis, Evaluating the quality of the LOD cloud: An empirical investigation, Semantic Web 9: (6) ((2018) ), 859–901. doi:10.3233/SW-180306. |
[22] | T. Declerck, J. Gracia and J.P. McCrae, COST action “European network for web-centred linguistic data science” (NexusLinguarum), Procesamiento del Lenguaje Natural 65: ((2020) ), 93–96. |
[23] | T. Declerck, J.P. McCrae, M. Hartung, J. Gracia, C. Chiarcos, E. Montiel-Ponsoda, P. Cimiano, A. Revenko, R. Saurí, D. Lee, S. Racioppa, J. Abdul Nasir, M. Orlikowsk, M. Lanau-Coronas, C. Fäth, M. Rico, M.F. Elahi, M. Khvalchik, M. Gonzalez and K. Cooney, Recent developments for the linguistic linked open data infrastructure, in: Proceedings of the 12th Language Resources and Evaluation Conference, European Language Resources Association, Marseille, France, (2020) , pp. 5660–5667, https://aclanthology.org/2020.lrec-1.695. ISBN 979-10-95546-34-4. |
[24] | L. Ding and T. Finin, Characterizing the semantic web on the web, 2006, pp. 242–257. ISBN 978-3-540-49029-6. doi:10.1007/11926078_18. |
[25] | O. Erling and I. Mikhailov, Virtuoso: RDF support in a native RDBMS, in: Semantic Web Information Management, Springer, (2010) , pp. 501–519. doi:10.1007/978-3-642-04329-1_21. |
[26] | I. Ermilov, J. Lehmann, M. Martin and S. Auer, LODStats: The data web census dataset, in: Proceedings of 15th International Semantic Web Conference – Resources Track (ISWC’2016), (2016) , http://svn.aksw.org/papers/2016/ISWC_LODStats_Resource_Description/public.pdf. doi:10.1007/978-3-319-46547-0_5. |
[27] | L. Feigenbaum, G.T. Williams, K.G. Clark and E. Torres, SPARQL 1.1 protocol, Recommendation, W3C (Mar. 2013), URL http://www.w3.org/TR/sparql11-protocol. |
[28] | M. Fiorelli, A. Stellato, J. McCrae, P. Cimiano and P. Maria Teresa, LIME: The metadata module for OntoLex, 2015, pp. 321–336. ISBN 978-3-319-18817-1. doi:10.1007/978-3-319-18818-8_20. |
[29] | M. Gavrilidou, P. Labropoulou, E. Desipri, S. Piperidis, H. Papageorgiou, M. Monachini, F. Frontini, T. Declerck, G. Francopoulo, V. Arranz, V. Mapelli and A. Ilsp, The META-SHARE metadata schema for the description of language resources, 2012, pp. 1090–1097. doi:10.13140/2.1.4302.4644. |
[30] | F. Giunchiglia, V. Maltese, F. Farazi and B. Dutta, GeoWordNet: A resource for geo-spatial applications, in: Extended Semantic Web Conference, Springer, (2010) , pp. 121–136. doi:10.1007/978-3-642-13486-9_9. |
[31] | M. Grobe, Rdf, jena, sparql and the ‘semantic web’, in: Proceedings of the 37th Annual ACM SIGUCCS Fall Conference: Communication and Collaboration, (2009) , pp. 131–138. doi:10.1145/1629501.1629525. |
[32] | S. Harris and A. Seaborne, SPARQL 1.1 query language, W3C recommendation 21 March 2013, URL: http://www.w3.org/TR/sparql11-query/, 2013. |
[33] | A. Hasnain, R. Fox, S. Decker and H.F. Deus, Cataloguing and linking life sciences LOD Cloud, in: 1st International Workshop on Ontology Engineering in a Datadriven World Collocated with EKAW12, (2012) , pp. 114–130. |
[34] | T. Heath and C. Bizer, Linked Data: Evolving the Web into a Global Data Space, Morgan & Claypool, (2011) . doi:10.2200/S00334ED1V01Y201102WBE001. |
[35] | E. Hinrichs and S. Krauwer, The CLARIN research infrastructure: Resources and tools for eHumanities scholars, in: Proceedings of the Ninth International Conference on Language Resources and Evaluation (LREC’14), European Language Resources Association (ELRA), Reykjavik, Iceland, (2014) , pp. 1525–1531, http://www.lrec-conf.org/proceedings/lrec2014/pdf/415_Paper.pdf. |
[36] | A. Hogan, J. Umbrich, A. Harth, R. Cyganiak, A. Polleres and S. Decker, An empirical survey of linked data conformance, Journal of Web Semantics 14: ((2012) ), 14–44, Special Issue on Dealing with the Messiness of the Web of Data, http://www.sciencedirect.com/science/article/pii/S1570826812000352. doi:10.1016/j.websem.2012.02.001. |
[37] | P. Jaiswal, S. Avraham, K. Ilic, E.A. Kellogg, S. McCouch, A. Pujar, L. Reiser, S.Y. Rhee, M.M. Sachs, M. Schaeffer et al., Plant Ontology (PO): A controlled vocabulary of plant structures and growth stages, Comparative and functional genomics 6: (7–8) ((2005) ), 388–397. doi:10.1002/cfg.496. |
[38] | C. Jonquet, A. Toulet, B. Dutta and V. Emonet, Harnessing the power of unified metadata in an ontology repository: The case of AgroPortal, Journal on Data Semantics 7: ((2018) ), 191–221. doi:10.1007/s13740-018-0091-5. |
[39] | M.R. Kamdar and M.A. Musen, An empirical meta-analysis of the life sciences linked open data on the web, Scientific Data 8: (1) ((2021) ), 1–21. doi:10.1038/s41597-021-00797-y. |
[40] | S. Krek, I. Kosem, J.P. McCrae, R. Navigli, B.S. Pedersen, C. Tiberius and T. Wissik, European lexicographic infrastructure (elexis), in: Proceedings of the XVIII EURALEX International Congress on Lexicography in Global Contexts, (2018) , pp. 881–892. |
[41] | J.P. McCrae, C. Chiarcos, F. Bond, P. Cimiano, T. Declerck, G. de Melo, J. Gracia, S. Hellmann, B. Klimek, S. Moran, P. Osenova, A. Pareja-Lora and J. Pool, The open linguistics working group: Developing the linguistic linked open data cloud, in: Proceedings of the Tenth International Conference on Language Resources and Evaluation (LREC’16), European Language Resources Association (ELRA), Portorož, Slovenia, (2016) , pp. 2435–2441, https://aclanthology.org/L16-1386. |
[42] | J.P. McCrae and P. Cimiano, Linghub: A linked data based portal supporting the discovery of language resources, SEMANTiCS (Posters & Demos) 1481: ((2015) ), 88–91. |
[43] | J.P. McCrae, P. Labropoulou, J. Gracia, M. Villegas, V. Rodríguez-Doncel and P. Cimiano, One ontology to bind them all: The META-SHARE OWL ontology for the interoperability of linguistic datasets on the web, in: The Semantic Web: ESWC 2015 Satellite Events, F. Gandon, C. Guéret, S. Villata, J. Breslin, C. Faron-Zucker and A. Zimmermann, eds, Springer International Publishing, Cham, (2015) , pp. 271–282. ISBN 978-3-319-25639-9. doi:10.1007/978-3-319-25639-9_42. |
[44] | R. Meusel, B. Spahiu, C. Bizer and H. Paulheim, Towards automatic topical classification of LOD datasets, in: Proceedings of the Workshop on Linked Data on the Web (LDOW2015), Vol. 1409: , (2015) . |
[45] | R. Navigli and S.P. Ponzetto, BabelNet: The automatic construction, evaluation and application of a wide-coverage multilingual semantic network, Artificial Intelligence 193: ((2012) ), 217–250. doi:10.1016/j.artint.2012.07.001. |
[46] | J. Nivre, M.-C. De Marneffe, F. Ginter, Y. Goldberg, J. Hajic, C.D. Manning, R. McDonald, S. Petrov, S. Pyysalo, N. Silveira et al., Universal dependencies v1: A multilingual treebank collection, in: Proceedings of the Tenth International Conference on Language Resources and Evaluation (LREC’16), (2016) , pp. 1659–1666. |
[47] | H. Paulheim, Knowledge graph refinement: A survey of approaches and evaluation methods, Semantic Web 8: ((2017) ), 489–508. doi:10.3233/SW-160218. |
[48] | G. Rehm, S. Piperidis, K. Bontcheva, J. Hajic, V. Arranz, A. Vasiljevs, G. Backfried, J.M. Gomez-Perez, U. Germann, R. Calizzano, N. Feldhus, S. Hegele, F. Kintzel, K. Marheinecke, J. Moreno-Schneider, D. Galanis, P. Labropoulou, M. Deligiannis, K. Gkirtzou, A. Kolovou, D. Gkoumas, L. Voukoutis, I. Roberts, J. Hamrlova, D. Varis, L. Kacena, K. Choukri, V. Mapelli, M. Rigault, J. Melnika, M. Janosik, K. Prinz, A. Garcia-Silva, C. Berrio, O. Klejch and S. Renals, European language grid: A joint platform for the European language technology community, in: Proceedings of the 16th Conference of the European Chapter of the Association for Computational Linguistics: System Demonstrations, (2021) , pp. 221–230. |
[49] | M. Schmachtenberg, C. Bizer and H. Paulheim, Adoption of the linked data best practices in different topical domains, in: International Semantic Web Conference, Springer, (2014) , pp. 245–260. doi:10.1007/978-3-319-11964-9_16. |
[50] | L.M. Schriml, E. Mitraka, J. Munro, B. Tauber, M. Schor, L. Nickle, V. Felix, L. Jeng, C. Bearer, R. Lichenstein et al., Human disease ontology 2018 update: Classification, content and workflow expansion, Nucleic Acids Research 47: (D1) ((2019) ), D955–D962. doi:10.1093/nar/gky1032. |
[51] | J. Sheridan and J. Tennison, Linking UK government data, in: Proceedings of the WWW 2010 Workshop on Linked Data on the Web (LDOW2010), (2010) . |
[52] | M.A. Sherif and A.-C. Ngonga Ngomo, Semantic quran, Semantic Web 6: (4) ((2015) ), 339–345. doi:10.3233/SW-140137. |
[53] | B. Spahiu, A. Maurino and R. Meusel, Topic profiling benchmarks in the linked open data cloud: Issues and lessons learned, Semantic Web 10: (2) ((2019) ), 329–348. doi:10.3233/SW-180323. |
[54] | P. Vandenbussche, J. Umbrich, L. Matteis, A. Hogan and C.B. Aranda, SPARQLES: Monitoring public SPARQL endpoints, Semantic Web 8: ((2017) ), 1049–1065. doi:10.3233/SW-170254. |
[55] | R. Verborgh, O. Hartig, B. De Meester, G. Haesendonck, L. De Vocht, M. Vander Sande, R. Cyganiak, P. Colpaert, E. Mannens and R. Van de Walle, Querying datasets on the web with high availability, in: International Semantic Web Conference, Springer, (2014) , pp. 180–196. doi:10.1007/978-3-319-11964-9_12. |
[56] | R. Verborgh, M. Vander Sande, P. Colpaert, S. Coppens, E. Mannens and R. Van de Walle, Web-scale querying through linked data fragments, in: Linked Data on the Web (LDOW2014), Citeseer, (2014) . |
[57] | M. Villegas, M. Melero and N. Bel, Metadata as linked open data: Mapping disparate XML metadata registries into one RDF/OWL registry, in: Proceedings of the Ninth International Conference on Language Resources and Evaluation (LREC’14), (2014) , pp. 393–400. |
[58] | P.L. Whetzel, N.F. Noy, N.H. Shah, P.R. Alexander, C. Nyulas, T. Tudorache and M.A. Musen, BioPortal: Enhanced functionality via new web services from the National Center for Biomedical Ontology to access and use ontologies in software applications, Nucleic Acids Res. 39: ((2011) ). doi:10.1093/nar/gkr469. |
[59] | M.D. Wilkinson, M. Dumontier, I.J. Aalbersberg, G. Appleton, M. Axton, A. Baak, N. Blomberg, J.-W. Boiten, L.B. da Silva Santos, P.E. Bourne et al., The FAIR guiding principles for scientific data management and stewardship, Scientific Data 3: (1) ((2016) ), 1–9. doi:10.1038/sdata.2016.18. |
[60] | A. Zaveri, A. Rula, A. Maurino, R. Pietrobon, J. Lehmann and S. Auer, Quality assessment for linked data: A survey, Semantic Web 7: ((2015) ), 63–93. doi:10.3233/SW-150175. |