Abstract
In case of multicollinearity, PRESS statistics has been proposed to be used in the selection of the ridge biasing parameter of the ridge estimator which is introduced as an alternative to BLUE. This newly proposed PRESS statistic for the ridge estimator, \(\textit{CPRESS}_{k}\), depends on the conditional ridge residual and can be computed once at a time by fitting the linear mixed model with all the observations. We also define \(R^2_{RidPred}\) statistic to evaluate the predictive ability of the ridge fit. Since the PRESS statistic for the BLUE is a special \(\textit{CPRESS}_{k}\) statistic, we indirectly also give closed form solution of the PRESS statistic for the BLUE. Then, we compared the predictive performance of the linear mixed model via the statistics \( \textit{CPRESS}_{k}\), \(GCV_{k}\) and \(C_{p}\) by considering a real data analysis and a simulation study where the optimal ridge biaisng parameter is obtained by minimizing each statistic. The study shows that the ridge predictors improve the predictive performance of a linear mixed model over BLUE in the presence of multicollinearity and each statistic gives a different optimum ridge biasing value and they show the best predictive performance at their optimum ridge biasing values. In addition, the simulation study has shown that the intensity of variance and multicollinearity is effective in determining the optimum ridge biasing value and this optimum ridge biasing value is effective on the superiority of the predictive performance of ridge estimator over BLUE.






Similar content being viewed by others
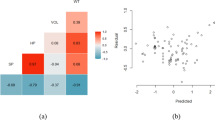
References
Abraham AA (2008) Model selection methods in the linear mixed model for longitudinal data [PhD thesis]. Department of Biostatistics, University of North Carolina
Açar TS, Özkale MR (2016) Cross validation of ridge regression estimator in autocorrelated linear regression models. J Statist Comput Simul 86:2429–2440
Allen DM (1974) The relationship between variable selection and data agumentation and a method for prediction. Technometrics 16:125–127
Belsley DA, Kuh E, Welsch RE (1980) Regression Diagnostics. Wiley, New York
Christensen R, Pearson LM, Johnson W (1992) Case deletion diagnostics for mixed models. Technometrics 34:38–45
Edwards LJ, Muller KE, Wolfinger RD, Qaqish BJ, Schabenberger O (2008) An \(R^{2}\) statistic for fixed effects in the linear mixed model. Stat Med 27:6137–6157
Elden L (1984) A note on the computation of the generalized cross-validation function for ill-conditioned least squares problems. BIT 24:462–472
Eliot M (2011) Tree-based methods and a mixed ridge estimator for analyzing longitudinal data with correlated predictors [Ph. D. Thesis]. Department of Biostatistics, University of Massachusetts Amherst
Harville DA (1977) Maximum likelihood approaches to variance component estimation and to related problems. J Amer Statist Assoc 72:320–338
Hemmerle WJ, Hartley HO (1973) Computing maximum likelihood estimates for the mixed AOV model using the W transformation. Technometrics 15:819–831
Henderson CR, Kempthorne O, Searle SR, Von Krosig CN (1959) Estimation of environmental and genetic trends from records subject to culling. Biometrics 15:192–218
Hodges JS, Sargent DJ (2001) Counting degrees of freedom in hierarchical and other richly-parameterised models. Biometrika 88:367–379
Hoerl AE, Kennard RW (1970) Ridge regression: biased estimation for nonorthogonal problems. Technometrics 12:55–67
Krzanowski WJ (1987) Selection of variables to preserve multivariate data structure, using principal components. J R Statist Soc C 36:22–33
Kuran Ö, Özkale MR (2016) Gilmour’s approach to mixed and stochastic restricted ridge predictions in linear mixed models. Linear Algeb Appl 508:22–47
Kuran Ö, Özkale MR (2021) Marginal ridge conceptual predictive model selection criterion in linear mixed models. Comm Statist Simul Comput 50:581–607
Kuran Ö, Özkale MR (2019) Model selection via conditional conceptual predictive statistic under ridge regression in linear mixed models. J Statist Comput Simul 89:155–187
Liu H, Weiss RE, Jennrich RI, Wenger NS (1999) PRESS model selection in repeated measures data. Comput Statist Data Anal 30:169–184
Liu XQ, Jiang HY (2012) Optimal generalized ridge estimator under the generalized cross-validation criterion in linear regression. Linear Algeb Appl 436:1238–1245
Liu XQ, Hu P (2013) General ridge predictors in a mixed linear model. J Theor Appl Statist 47:363–378
Mallows CL (1973) Some comments on Cp. Technometrics 15:661–675
McCulloch CE, Searle SR (2001) Generalized, linear, and mixed models. Wiley, New York
McDonald MC, Galarneau DI (1975) A Monte Carlo evaluation of some ridge-type estimators. J Amer Statist Assoc 70:407–416
Montgomery DC, Friedman DJ (1993) Prediction using regression models with multicollinear predictor variables. IIE Trans 25:73–85
Montgomery DC, Peck EA, Vining GG (2012) Introduction to linear regression analysis, 5th edn. Wiley, New York
Newhouse JP, Oman SD (1971) An evaluation of ridge estimators. Rand Corporation R-716-PR:1-28
Orelien JG, Edwards LJ (2008) Fixed effect variable selection in linear mixed models using \(R^{2}\) statistics. Comput Stat Data Anal 52:1896–1907
Özkale MR (2015) Predictive performance of linear regression models. Statist Papers 56:531–567
Özkale MR, Can F (2017) An evaluation of ridge estimator in linear mixed models: an example from kidney failure data. J Appl Stat 44:2251–2269
Özkale MR, Kuran Ö (2018) Principal components regression and r-k class predictions in linear mixed models. Linear Algeb Appl 543:173–204
Pinherio JC, Bates DM (2000) Mixed-effects in S and S-Plus. Springer-Verlag, New York
Potthoff RF, Roy SN (1964) A generalized multivariate analysis of variance model especially useful for growth curve problems. Biometrika 51:313–326
Robinson GK (1991) That BLUP is a good thing: the estimation of random effects (with discussion). Statist Sci 6:15–51
Schabenberger O (2004) Mixed model influence diagnostics. Statist Data Anal 1:17
Searle SR, Casella G, McCulloch CE (1992) Variance Components. Wiley, New York
Sheather SJ (2009) A Modern Approach to Regression with R. Springer Science and Business Media, New York
Vonesh EF, Chinchilli VM (1997) Linear and Nonlinear Models for the Analysis of Repeated Measurements. Marcel Dekker, New York
Wahba G, Golub GH, Heath M (1979) Generalized cross-validation as a method for choosing a good ridge parameter. Technometrics 21:215–223
Wang J, Schaalje GB (2009) Model selection for linear mixed models using predictive criteria. Comm Statist Simul Comput 38:788–801
Wenren C (2014) Mixed model selection based on the conceptual predictive statistic. Ph. D. Thesis, Department of Mathematics and Statistics, University of Bowling Green State
Wenren C, Shang J, Pan J (2016) Marginal conceptual predictive statistic for mixed model selection. Open J Statist 6:239–253
Wenren C, Shang J (2016) Conditional conceptual predictive statistic for mixed model selection. J Appl Stat 43:585–603
Tarpey T (2000) A note on the prediction sum of squares statistic for restricted least squares. Am Stat 54:116–118
Zewotir T, Galphin JS (2005) Influence diagnostics for linear mixed models. J Data Sci 3:153–177
Author information
Authors and Affiliations
Corresponding author
Ethics declarations
Conflict of interest
No potential conflict of interest was reported by the authors.
Additional information
Publisher's Note
Springer Nature remains neutral with regard to jurisdictional claims in published maps and institutional affiliations.
Rights and permissions
Springer Nature or its licensor (e.g. a society or other partner) holds exclusive rights to this article under a publishing agreement with the author(s) or other rightsholder(s); author self-archiving of the accepted manuscript version of this article is solely governed by the terms of such publishing agreement and applicable law.
About this article
Cite this article
Kuran, Ö., Özkale, M.R. The ridge prediction error sum of squares statistic in linear mixed models. Metrika (2023). https://doi.org/10.1007/s00184-023-00927-z
Received:
Accepted:
Published:
DOI: https://doi.org/10.1007/s00184-023-00927-z