The past, present and future of neuroscience data sharing: a perspective on the state of practices and infrastructure for FAIR
- 1Department of Neurosciences, University of California, San Diego, CA, United States
- 2San Francisco Veterans Administration Hospital, San Francisco, CA, United States
Neuroscience has made significant strides over the past decade in moving from a largely closed science characterized by anemic data sharing, to a largely open science where the amount of publicly available neuroscience data has increased dramatically. While this increase is driven in significant part by large prospective data sharing studies, we are starting to see increased sharing in the long tail of neuroscience data, driven no doubt by journal requirements and funder mandates. Concomitant with this shift to open is the increasing support of the FAIR data principles by neuroscience practices and infrastructure. FAIR is particularly critical for neuroscience with its multiplicity of data types, scales and model systems and the infrastructure that serves them. As envisioned from the early days of neuroinformatics, neuroscience is currently served by a globally distributed ecosystem of neuroscience-centric data repositories, largely specialized around data types. To make neuroscience data findable, accessible, interoperable, and reusable requires the coordination across different stakeholders, including the researchers who produce the data, data repositories who make it available, the aggregators and indexers who field search engines across the data, and community organizations who help to coordinate efforts and develop the community standards critical to FAIR. The International Neuroinformatics Coordinating Facility has led efforts to move neuroscience toward FAIR, fielding several resources to help researchers and repositories achieve FAIR. In this perspective, I provide an overview of the components and practices required to achieve FAIR in neuroscience and provide thoughts on the past, present and future of FAIR infrastructure for neuroscience, from the laboratory to the search engine.
Introduction
The transformation of neuroscience from a closed to an open science, where the entirety of research products like data and code produced during a study are routinely made available, has accelerated in recent years. Data sharing requires that the necessary human and technical infrastructure be in place to make these data broadly available. The first Human Brain Project, funded by the US National Institute of Mental Health in the 1990s, launched some of the first efforts to “database the brain,” envisioning a “paradigm shift in which primary data are openly shared with the worldwide neuroscience community” (Koslow, 2000). Despite this early optimism, neuroscience had a rocky history with open data sharing. Unlike the genomics and structural biology communities where the mechanisms and value of sharing primary sequence and structural data were agreed upon fairly early, the how and why of sharing the more diverse and complex data types of neuroscience was met with early resistance (Whose Scans Are They, Anyway?, 2000). In these early days, before the spotlight was shown on reproducibility problems facing neuroscience (Ioannidis, 2007; Button et al., 2013) and before “big data” became a buzzword in neuroscience and across biomedicine, there were few motivations or incentives for researchers to share their data openly. Like other areas of biomedicine (Nelson, 2009), neuroscience archives were largely underpopulated relative to the amount of data generated in Table 1 (Ferguson et al., 2014).
Neuroscience started to put its first big stake in the ground for open data sharing with the commissioning of large prospective data sharing efforts where large, comprehensive data sets were collected by large teams of scientists with the goal of making them openly available. Some of early efforts include the Alzheimer’s Disease Neuroimaging Initiative (ADNI; Weiner et al., 2010) launched in 2004 and Allen Brain Atlas launched in 2005, followed by large consortia such as the Human Connectome Project (2011) and the Big Brain (2013; Amunts et al., 2013) among many others. The large national and international brain projects launched in the second decade of the 21st century articulated a strong commitment to the open sharing of data and tools. The European Human Brain Project (HBP) was launched in 2013, followed by the US Brain Research through Advancing Innovative Neurotechnologies (BRAIN) Initiative (2014), the Korean Brain Initiative (2016), Canadian Brain Research Strategy (2017), Japan BRAIN/Minds (2018), and the China (2021) and Australian Brain Projects (International Brain Initiative, 2020; Quaglio et al., 2021). These projects have provided a significant infusion of resources to develop the next generation infrastructures necessary to house the sizes and complexity of data developed through new imaging, genomic, and physiological techniques.
An updated analysis of the repositories listed in Ferguson et al. (2014) provides some data on the current state of data sharing. Table 1 shows that data sharing has increased overall, but it is uneven, with explosive growth in some repositories, e.g., NeuroMorpho.org and FigShare, and more modest growth in others. But with the release of the data sharing mandates by funding agencies around the globe (Funders’ Policies, 2015; Eke et al., 2022), neuroscience-whether practiced by large consortia or individual labs-is now expected to be “open by default and open by design” (National Academies of Sciences, Engineering, and Medicine, 2018). So the question is no longer whether neuroscience as a whole will share data, it is how effectively? We are seeing some real success stories emerging in neuroscience from the reuse of data, (e.g., Torres-Espín et al., 2021; Almeida et al., 2022) and the ability for multiple groups to analyze the same datasets are providing new insights into notions of reproducibility and robustness (Botvinik-Nezer et al., 2020), but public data are still often difficult to find and use. Effective data sharing, that is, data sharing that views data as a public product of research meant to be reused, referenced, and respected requires the infrastructure, skills, tools, and willingness on the part of the neuroscience community to value data as a research product (Martone and Nakamura, 2022).
Effective data sharing starts with the FAIR data principles (Wilkinson et al., 2016) which grew out of frustrations experienced when trying to use open data on the web in the early days of sharing data. Through the Neuroscience Information Framework (NIF), started in 2008 (Gardner et al., 2008), we were tasked with cataloging all the neuroscience-relevant digital products that were being created (Cachat et al., 2012). NIF was also tasked with developing a strategy to query across the dozens of neuroscience data-and knowledge bases and the 100’s of biomedical databases with neuroscience-relevant information that were coming on-line. In these early days of on-line databases, the problems with accessing the data were legion: broken links, insufficient metadata, non-standardized vocabularies and nomenclature, non-actionable data formats, cryptic variables, and proprietary formats to name a few.
FAIR states the minimum set of requirements for digital data for it to be useful: data should be findable, accessible, interoperable, and reusable. FAIR then lays out a set of practices that would make it more likely that data will meet these requirements. The FAIR data principles were formulated in a workshop in Leiden in 2014 (Wilkinson et al., 2016), and were first released through FORCE11, the Future of Research Communications and e-Scholarship. The paper came out 2 years later in 2016. When our group participated in the 2017 kick off meeting for the BRAIN Initiative Cell Census Network (BICCN), a large consortium designed to use multimodal data techniques to determine the major cell types in the brain, few hands were raised when we asked how many people had heard of FAIR. Fortunately, FAIR eventually made its way to neuroscience and found a natural home in the International Neuroinformatics Coordinating Facility (INCF.org), an international organization devoted to developing standards and coordinating infrastructures for neuroscience. INCF incorporated FAIR into its mission statement and has served as a coordinating center for introducing neuroscience to FAIR through its role as a standards organization for neuroscience, its training programs, and other resources (Abrams et al., 2021).
The FAIR partnership
The FAIR acronym itself is now likely better known among practicing neuroscientists, as funders and journals have started to support FAIR in their data sharing policies; but the details of FAIR as elaborated in the detailed recommendations are fairly arcane. Anyone outside the field of informatics is likely to look at these and scratch their head. Persistent identifiers? Knowledge representation languages? A plurality of relevant attributes? Thus, while the practicing neuroscientist may understand what FAIR stands for, they are often at a loss to explain exactly how to achieve it. In reality, no one can create fully FAIR data alone; it requires the interplay of data acquisition and documentation practices, infrastructure, informatics, and community consensus. FAIR is therefore best thought of as a partnership between investigators, data repositories, data aggregators and community organizations (Figure 1). Navigating the landscape of FAIR data sharing and neuroscience infrastructure requires understanding the roles, responsibilities, and interfaces between each of these stakeholder groups. In the following I discuss the different components and some of the tasks required for FAIR and provide information and resources to help navigate the different components required for fully FAIR neuroscience.
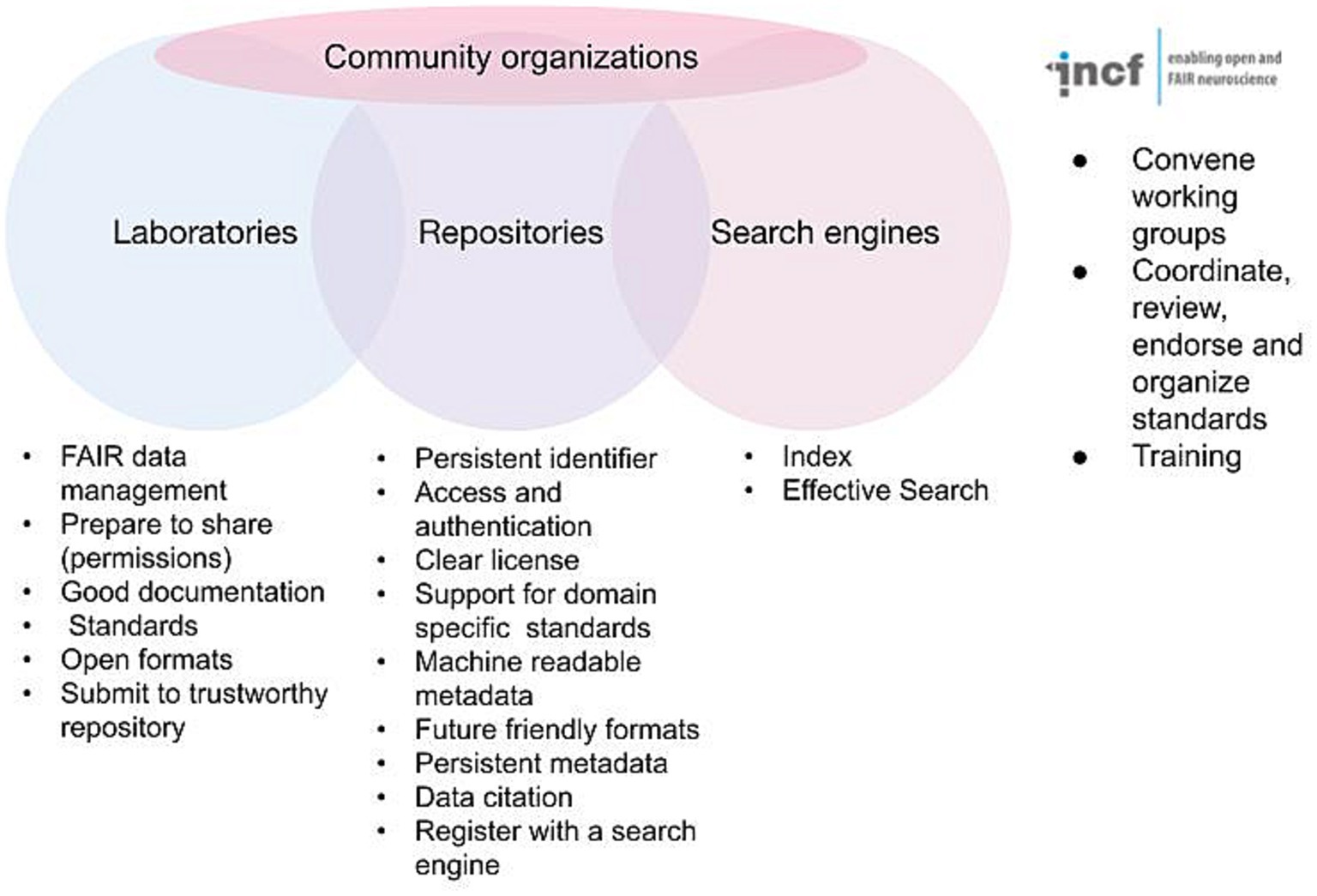
Figure 1. Major stakeholders involved in defining and implementing FAIR. Some of the major requirements for achieving FAIR are listed under each stakeholder group. The INCF is given as an example of a community organization supporting FAIR for neuroscience.
Laboratories
FAIR data management
In the US National Academies of Science, Engineering and Medicine workshop on “Changing the Culture on Data Management and Sharing (Martone and Nakamura, 2022), one of the main takeaways was that the focus of data sharing efforts should not be targeted toward the individual investigator, but the laboratory. As one participant noted: “If you can share data with people in your lab, you are much more likely to have something worthwhile to share outside the lab.” FAIR data management is therefore an intentional lab-wide strategy that ensures that data can be shared with lab mates, the PIs, and other colleagues, your future self and eventually with the broader scientific community. Across all stages of the data lifecycle, the management strategy puts in place processes so that data can be found, accessed, combined when necessary, and reused. By paying attention to FAIR in the laboratory throughout the life cycle, benefits start to accrue to the data creator, the laboratory, PI, and collaborators well before data flows out to the wider scientific community (Bush et al., 2022; Dempsey et al., 2022).
Examples of lab management practices built on the FAIR principles are given in Table 2.
We are starting to see neuroscience researchers sharing their experiences with developing and utilizing lab-centric data management systems. They range from tightly integrated digital infrastructures (Bush et al., 2022; Dempsey et al., 2022) to a set of practices that can be implemented using “off the shelf” components for an average neuroscience wet lab (Fouad et al., 2023).
Choosing a repository
One of the most important steps for a researcher in ensuring that their data is FAIR for the long term is to submit their data to a trustworthy repository that supports FAIR. The new NIH data sharing policy requires researchers to indicate where they will be sharing their data as part of the data management and sharing plan. As recommended in Table 2, knowing in what repository the data will be published allows the researcher to understand what standards are required so they can be built into the laboratory management workflow. With its growing ecosystem of specialized databases, researchers have a choice about where to publish their data.
Understanding how the neuroscience repository landscape is organized may help in finding the right repository. Repositories are generally specialized by data type (Figure 2). However, repositories also exist that are specialized for a domain, e.g., the SPARC database accepts all data associated with the peripheral nervous system, or serve researchers within a particular region, e.g., CONP, or institution, e.g., BrainCode and the Donders Repository. Often, multiple repositories may be appropriate, in which case there are additional features that may make a given repository more or less attractive. These include tool support, curation services, support for data citation, choice of license, size of data allowed, help with data management plans (see Table 2) and possible costs (Murphy et al., 2021). A functioning neuroscience ecosystem also requires open neuroscience repositories that have few restrictions on data types, regions, or subdisciplines to ensure that all data has a home. The EU EBRAIN infrastructure is an example of such a repository, as it takes multiple types of data regardless of discipline or geographical location, although there may be issues with transferring certain types of data across international borders (Eke et al., 2022).
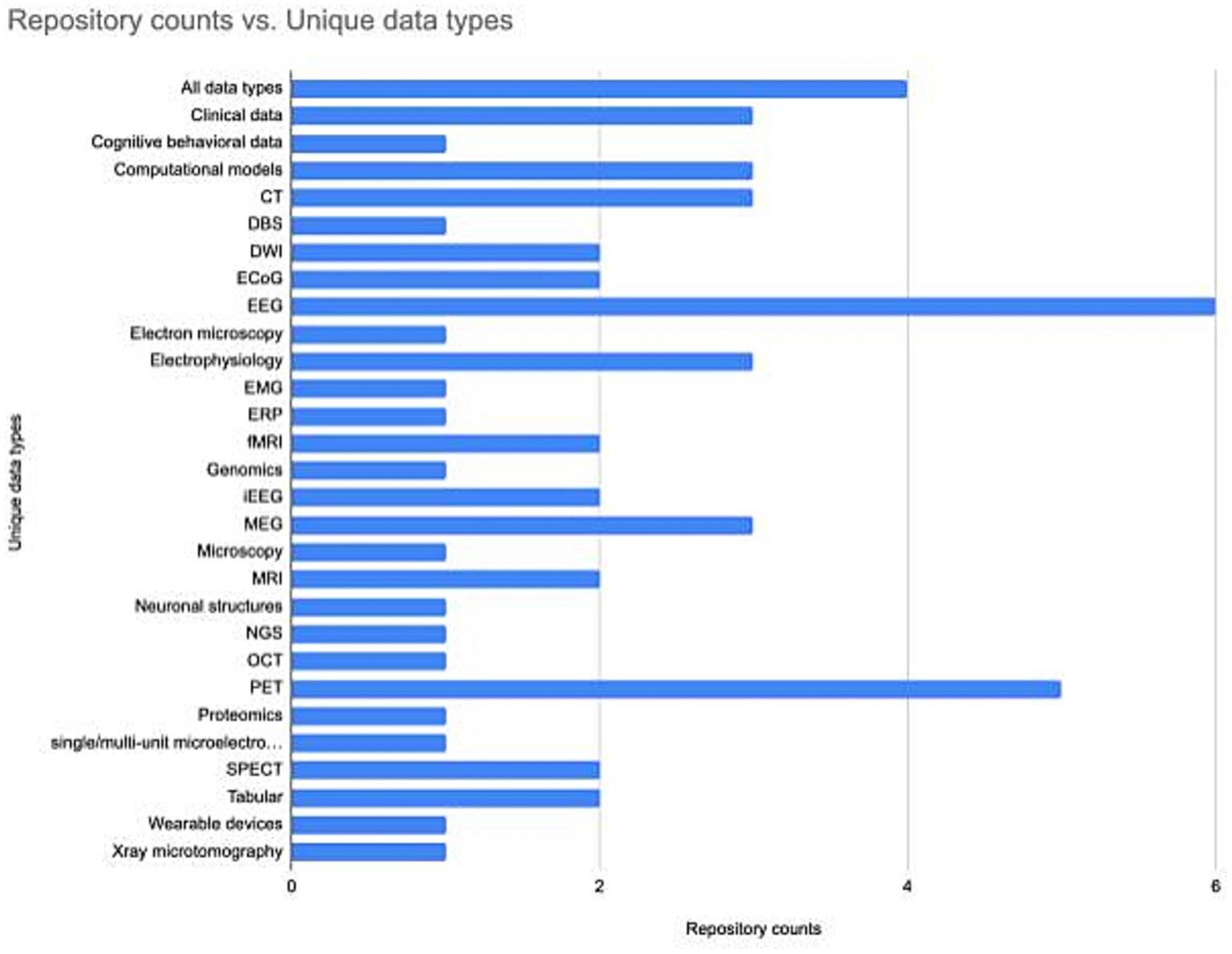
Figure 2. The number of neuroscience specialist repositories supporting different data types. The repository list and associated data types was assembled using information available through the INCF Infrastructure Portfolio and the SciCrunch Registry. The data underlying the figure is available at Zenodo, DOI: 10.5281/zenodo.8239845.
Supplementing the specialist repository landscape are the generalist repositories, data repositories that span scientific disciplines and data types (Assante et al., 2016). These repositories are often useful for publishing smaller supplemental datasets that are required for a publication (Stall et al., 2023). Specialist repositories generally provide more standards, tools and services for harmonizing and using data, and make it easier for researchers to find data of a particular type. To aid researchers in choosing an appropriate neuroscience data repository, the INCF has a searchable infrastructure catalog, where each repository is described according to the checklist developed by Sandström et al. (2022). Other repository finder tools include NITRC for neuroimaging related repositories, re3data, the catalog of open data repositories maintained by the National Library of Medicine, and the NIF listing of BRAIN Initiative Repositories.
Repositories
The central role of community repositories
While the investigator takes the central role in acquiring data in a manner that supports FAIR, the community repository is arguably the central player in implementing the basic requirements for achieving FAIR for the long term (Figure 1). We are using the term “community repository” here to designate infrastructures that are designed to accept primary data contributed by outside researchers, rather than a single data set produced by a given project (e.g., the Allen Brain Atlas) or a knowledge base that aggregates information about a particular entity (e.g., CoCoMac).1 As shown in Figure 1, the repositories have critical responsibilities for ensuring that submitted data are made available according to the FAIR principles (Lin et al., 2020). These practices include issuing and maintaining persistent identifiers, tying those identifiers to rich metadata, providing access and any necessary access controls, enforcing or supporting annotation with FAIR vocabularies, enforcing or supporting community standards, supporting data versioning, providing links to other critical products like experimental protocols and code, and provisioning a clear data license for each data set. Repositories also have the critical role of ensuring that data is available for the long term.
From the earliest days of neuroinformatics, it was envisioned that neuroscience would likely best be served by a decentralized system of federated databases (Koslow, 2000). Due to the variety and complexity of neuroscience data, a single large repository like Genbank or the Protein Data Bank was likely not going to be feasible. The early investments in neuroinformatics by the US Human Brain Project and the success of the International Neuroinformatics Coordinating Facility in growing the field of neuroinformatics globally, led to the first generation of neuroscience databases. These databases were largely organized around data type, e.g., structural neuroimaging (XNAT), functional neuroimaging (fMRI Data Center; Open fMRI), neurophysiology (CARMEN; Neurodatabase.org,” GNode), EEG (open EEG, iEEG), neuronal morphology (NeuroMorpho), microscopic images (Cell Centered Database), neuromodeling (ModelDB). Some examples are shown in Table 1.
When the first generation of neuroscience databases were started, there were few standard practices for designing web-accessible databases. As documented by NIF, each database had a different mode of access, different data structure, and the use of standards was very limited. It was a time of tremendous technological fluidity, with standard features we take for granted today (e.g., RESTful web APIs) still being invented. The cloud did not exist, and attempts to build resources on the early version of a cloud-like system (“the grid”) met with considerable challenges (Grethe et al., 2005). With today’s emphasis on data sharing, increased attention is starting to be paid to these critical infrastructures and how they are constructed, operated, and evaluated (Nelson, 2022). Various recommendations on desired characteristics for data repositories have been issued by different groups (Sansone et al., 2020; Shearer, n.d.), including NIH (Selecting a Data Repository) and additional sets of principles, e.g., the TRUST principles (Lin et al., 2020) and principles for open infrastructures (Bilder et al., 2015) have been formulated to help further guide how these critical infrastructures should operate. The Elixir project, a large scale bioinformatics consortium in the EU, has developed a maturity model for evaluating the success of repositories which is designed to be used by funders to determine the criticality of various infrastructures (Bahim et al., 2020). The INCF Infrastructure working group recently issued a set of guidelines from a neuroscience perspective, that provide a mix of technical and “customer service” recommendations for operating repositories (Sandström et al., 2022). Although these various lists of desiderata do not overlap completely (Murphy et al., 2021), over time we will likely converge on a core set of functions and expectations for these critical infrastructures, balancing the often dual requirement for these infrastructures to serve as both publishing platforms and dynamic scientific gateways (Sandström et al., 2022).
INCF has served as an important conduit by which the FAIR principles have permeated the construction of neuroscience data repositories and gateways. Investigators who have been active in INCF through governance, committees and working groups are involved with several of the next generation neuroscience infrastructures including EBrains, CONP, SPARC, DANDI, Open Neuro, and BRAIN/Minds. Table 3 lists and compares some of the key ways that these infrastructures implement FAIR and “FAIR-adjacent” practices. Following consistent design principles that support FAIR provides a level of common functionality and services that make it easier to work across these databases for an individual user or an automated agent. The more similar FAIR practices are across repositories, the more likely it is that the repositories themselves are interoperable.
Standards: role of repositories
A significant and positive change that is accelerating progress toward FAIR is the emergence of a set of robust standards for neuroscience data types that are starting to gain adoption. The INCF was created to help with this process of standardization and produced some early successes, e.g., the Waxholm space for registration of mouse and rat brain data (Hawrylycz et al., 2011; Papp et al., 2014), the Neuroimaging Data Model (Keator et al., 2013) the Brain Imaging Data Structure (Gorgolewski et al., 2016) were produced with support from INCF. Over the last few years, a set of standards has emerged for major neuroscience data types that can accommodate the increased size and complexity of neuroscience data through additional investments by funders and the efforts of the large brain projects, e.g., NWB, 3D-MMS (Ropelewski et al., 2022). Repositories serve as important stakeholders in ensuring that standards are followed by supporting or requiring them for data submission (Figure 2). Data uploaded to OpenNeuro, for example, must be validated against BIDS before it is accepted. The INCF has implemented an open community review and endorsement process to help improve the quality, usability, interoperability and awareness of these standards (Abrams et al., 2021). They have made available a searchable Standards and Best Practices Portfolio2 where researchers can learn about each standard and how it can be used. FAIRsharing.org more broadly aggregates standards from across biomedicine and makes them available through a searchable catalog.
As neuroscience standards become more mature, better supported, and more widely used, they provide the seeds for knitting the landscape of neuroscience data repositories into a true data ecosystem, where (meta)data can flow from the laboratory to repositories and from repositories to computational tools and back again. Figure 3 shows a graph illustrating the connections between standards (light gray) and infrastructures that support them (dark gray). The data was assembled from the INCF Infrastructure Catalog, FAIRsharing, the SciCrunch Registry (Subash et al., 2023) and examination of repository websites. As shown in Figure 3, multiple repositories and infrastructures are connected via these standards. For example, the Brain Imaging Data Structure (BIDS; Gorgolewski et al., 2016) links 10 different repositories and computational platforms. The success of BIDS has led to extensions of BIDS for other modalities through a formal governance process (Governance, n.d.). The adoption of these BIDS-based standards starts to create a degree of interoperability across data types.
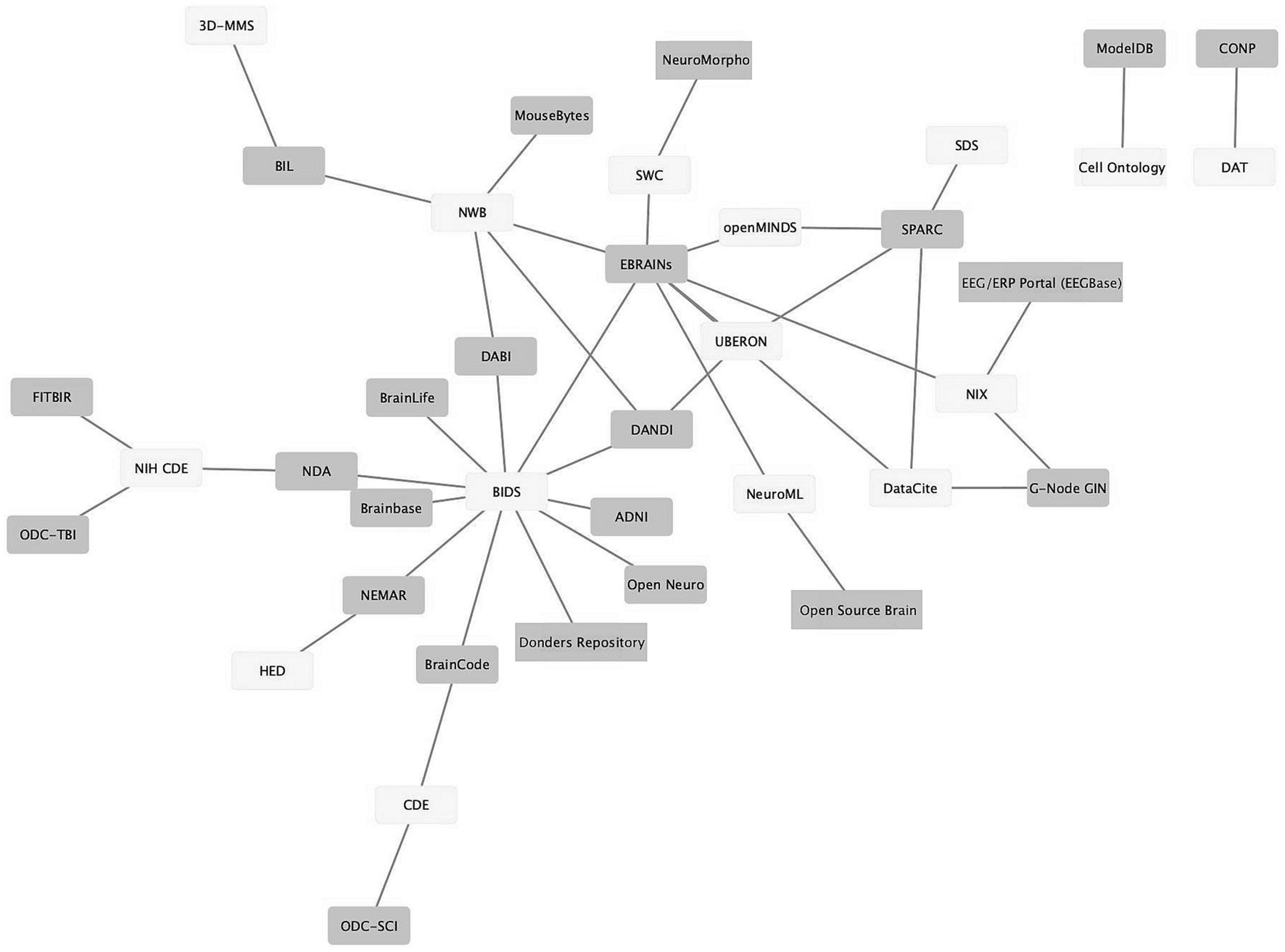
Figure 3. Ecosystem of neuroscience resources emerging around standards. Network graph of neuroscience data repositories and gateways (purple) and some of the standards they support (yellow). The graph shows repositories/gateways connected via the use of a common standard. A description of how standards were determined is given in the text.
As tool support grows, standards are also making their way into the laboratory. BIDS, for example, has been estimated to have been used to organize over 100,000 datasets containing millions of images, indicating significant uptake by the research community (Poldrack et al., 2023). In a recent paper that outlined a neuroimaging center’s implementation of BIDS, Bush et al. (2022) stated: “Learning the BIDS specification, implementing software pipelines to map the data, and validating that the resultant mappings met the BIDS standard consumed many months of effort across multiple imaging center team members… The benefits of mapping our data to BIDS, however, far exceed the costs.” (Bush et al., 2022). These benefits included access to BID-APPS, a set of containerized analysis tools and pipelines that run on validated BIDS data, as well as improved code sharing within the lab and with colleagues, as well as a reduced barrier to publishing the data in OpenNeuro. Similarly, the electrophysiology standard, NWB, has made inroads in tackling one of the most challenging data types in neuroscience, evidenced by uptake in laboratories (Rübel et al., 2022) and support by platforms such as DANDI and EBRAINs.
Standards: use of FAIR vocabularies and common coordinate frameworks
Interoperability across neuroscience data has always been hampered by the multiplicity of nomenclatures and parcellation schemes from brain regions and nerve cells (Martone et al., 2004). Although slow, progress has been made. Some repositories are starting to map generic neuroanatomical structures to community ontologies like UBERON (Mungall et al., 2012). Mapping data to a common coordinate framework (CCF) allows more precise localization independent of labels applied to them (Hawrylycz et al., 2023). Encouraging signs are emerging, as CCFs for multiple species are in use or in development for the major species across the international brain projects. For example, both the BICCN/BICAN and EBrains are utilizing the Allen Institute Common Coordinate Framework v3 for mouse (Hawrylycz et al., 2009, 2023). To help manage the different versions and components that go into these atlas-based environments, a new standard for describing and versioning brain atlases was recently proposed (Kleven et al., 2023).
Standardized nomenclature for cellular taxonomies and transcriptionally defined cell types are also emerging from projects like the BICCN/BICAN to help deal with the plethora of new cell types that are emerging from new transcriptomics-based approaches (Miller et al., 2020; Tan et al., 2023). Over the years, there have been proposals for naming neurons that can bridge the multiplicity of phenotypes generated by multiple experimental techniques (Hamilton et al., 2012; Shepherd et al., 2019; Gillespie et al., 2022). However, these approaches have had difficulty in handling the complex expression patterns coming out of transcriptomics. The BICCN/BICAN recently developed the Brain Standards Data Ontology, providing a model for providing data-driven definitions of taxonomic classes (Hawrylycz et al., 2023; Tan et al., 2023). BICCN has recently introduced Cell Cards to provide a tool for exploring the BICCN taxonomic cell types for human, marmoset, and mouse primary motor cortex, including linking them to primary data sets (Hawrylycz et al., 2023). As new technologies are allowing us to derive wider scale, more complete representations of the molecular, morphological, physiological, and connectional phenotypes of neurons than was possible in the past, it is time for the global neuroscience community to come together around a common nomenclature for naming populations of cells that will aid in comparison across studies.
Services for accessing ontologies and building them into annotation and metadata pipelines have improved significantly over the past decade, with tools such as BioPortal3 and the Ontology Look Up Service4 providing programmatic access to community ontologies. Nevertheless, neuroscience is still a cutting edge science where many new terms are needed, particularly for annotating experimental data. For this reason, NIF and INCF had developed the NeuroLex Wiki (Larson and Martone, 2013) that lowered the barrier for creating new ontology terms. When the semantic wiki technology underlying NeuroLex was no longer available, the approach and content were ported to the Interlex on-line vocabulary management system by NIF (Surles-Zeigler et al., 2021). Interlex mints a unique identifier for each term (URI) when it is entered and allows the addition of basic metadata for each term, e.g., definition, synonyms. It also provides basic knowledge engineering functions, e.g., parent–child and other relationships, annotations. Interlex also provides various review and curation functions. These specialized terms can be used as controlled vocabularies or further engineered into ontologies as needed. Surles-Zeigler et al. (2021) provide a description of how Interlex is being used to enhance anatomical annotation of SPARC data, models and knowledge base, allowing new anatomical terms to be minted, curated, linked to existing ontologies and contributed as necessary to augment community ontologies.
On the sustainability of neuroscience data repositories
As most neuroscience infrastructure is researcher-led and grant-supported, questions often arise about long-term sustainability when choosing a repository, or indeed, any infrastructure. Sustainability of individual resources remains a challenge, not just for neuroscience but for all research-led infrastructures that rely on grant funding for their operation. Of the data repositories listed in Table 1 taken from Ferguson et al. (2014), 4/18 are no longer in service and 3/18 are moribund (i.e., not taking data). Three were rebranded and expanded their scope, and one merged with another database. The good news is that the majority of this first generation of neuroscience databases are still in existence, indicating a degree of stability. We can also see movement in the ecosystem, with databases merging with others, or moving across institutions indicating a degree of dynamism that keeps the ecosystem healthy. Looking at a larger sample using the SciCrunch Registry (formerly the NIF Registry; Ozyurt et al., 2016) out of a total of 563 neuroscience data resources (including data repositories, databases, data sets, atlases and knowledge bases), 71 appear to be out of service (~13%). These numbers compare favorably to a study done on the longevity of bioinformatics biological databases founded in the late 20th century, 63% of which were defunct by 2015 (Attwood et al., 2015). In 2016 NIF began to track the usage of these neuroscience resources within the scientific literature (Ozyurt et al., 2016), revealing interesting patterns including the creation of thousands of data repositories across biomedicine. A recent analysis showed that only a handful of these repositories are actively used, with many of the neuroscience repositories referenced here among them, suggesting that neuroscience is coalescing around a set of core resources (Piekniewska et al., 2023). Thus, while sustainability is always a concern, neuroscience repositories have generally been good stewards of their data, utilizing a variety of strategies to keep data safe and accessible.
As neuroscience data and repositories start to align around the FAIR principles, the ecosystem should become more robust as it will make it easier for other repositories to absorb data if a repository loses its funding. Merging of similar resources also makes the ecosystem more efficient. The ‘professionalization” of scientific data repositories also means that researchers are taking their role as an archive more seriously. The INCF recommendations for neuroscience infrastructure include that repositories should have an exit plan and they should clearly state their persistence policy (Sandström et al., 2022). For example, some repositories are partnering with institutional libraries or other resources to ensure that data remain available, even if funding is lost (e.g., EBRAINS). Another promising development is the repurposing of infrastructure components. Rather than building a separate data repository, two computational and analytic platforms, Brainlife and NEMAR, utilize Open Neuro as their data platform, even as they field their own portals with their own branding. The ODC-SCI and ODC-TBI share the same infrastructure (SciCrunch; Surles-Zeigler et al., 2021), but each have their own separate community portal where they can access data and establish their own governance rules. The more that neuroscience infrastructure can be repurposed for new projects, the less funding needs to go to building and maintaining new infrastructures.
Search engines
In tandem with the vision of a distributed system of databases laid out by the NIH HBP was the creation of a neuroscience portal where data could be accessed via a “a smart ‘neuroscience browser’ instructed to look for a particular variable or set of variables and import the data back to the user’s computer” (Koslow, 2000). For the distributed ecosystem to work effectively, users would have to be able to issue dynamic queries across these databases and be able to retrieve the necessary subsets of data. And, in fact, FAIR states that data should be registered with an appropriate index (F4). NIF set up one of the first searches across neuroscience databases by creating an index over the contents of distributed databases. At its height, NIF queried over 200 data sources across biomedicine comprising over 8 million data records (Cachat et al., 2012). NIF used the NIFSTD to help mediate across the different vocabularies and relationships that were needed to link across databases. NIF was able to align different databases covering the same content across a core set of variables, but did not have the resources to harmonize the content, especially given the lack of standards at that time. NIF was designed to allow researchers to understand what was in a given database by providing limited views of the data, but not to perform deep structured queries of the content. So you could use NIF to identify a database that had relevant data, but for more structured queries and to retrieve the complete data, users needed to visit the source database. The INCF Knowledge Space and currently performs a similar type of search over 16 major neuroscience databases (KnowledgeSpace, n.d.).
The more that repositories enforce consistent standards for metadata and data formats, the closer neuroscience gets toward achieving true federated search and retrieval across the entirety of the neuroscience repository ecosystem (Koslow, 2000). The Canadian Open Neuroscience Portal was recently launched that allows users to search across data hosted in multiple data repositories. It is currently deployed across 17 Canadian institutions and also integrates select specialist and generalist repositories. All the high level metadata is aligned to the DATS standard, developed by the NIH-funded BioCADDIE Big Data to Knowledge project (Alter et al., 2020), allowing for a unified dataset search. The portal implements some uniform functions that can be executed directly from the portal. Some data are available for download via DataLad and containerized workflows that work across these distributed data are available via Boutiques (Poline et al., 2023).
New tools are also becoming available that lower the barrier to making content available to search engines. For example, multiple neuroscience databases have marked up their content with schema.org so that their datasets are searchable through Google Dataset Search (Table 3). Neuroscience, like other domains, is building knowledge graphs that link neuroscience concepts to each other and to datasets to aid in search.EBrains, CONP and the SPARC projects are making their data available via a knowledge graph. CONP uses the Nexus knowledge graph developed by the Blue Brain Projects which provides a set of tools and resources for searching, linking and viewing data.5
Community organizations
The FAIR data principles delegate a good amount of responsibility to individual communities to define what is FAIR for their domain. Community organizations play an important role as coordinators by serving as conveners to allow researchers to come to consensus about best practices and recommendations for their community. International neuroscience is currently supported by two community organizations, the INCF and the IBI. IBI is principally focused on coordination of the large international brain projects, focusing on data sharing among these projects, as well as issues such as data governance and ethics. INCF works across all neuroscience efforts, whether individual or team based, and focuses on standards, infrastructure coordination and training. Both organizations provide support for working groups that come together to tackle issues such as the development of international data governance (IBI), standards and best practices (INCF, IBI), training (INCF), and coordination of infrastructures (INCF, IBI). Any member of INCF can propose a working group and membership is open to the community, while IBI working groups are set by the Strategy Committee. The two organizations work together and with other organizations such as the IEEE Neuro Standards working group and the Global Brain Consortium.6 In this way, there is a level of coordination across these international organizations. Eke et al. (2022) raised the issue of whether neuroscience needs an umbrella organization modeled after the Global Alliance for Genomic Health, to more effectively address data reuse at the technical, ethical, sociological and political level.
Is neuroscience FAIR yet?
Neuroscience has made tremendous progress over the first two decades of the 21st century in establishing the infrastructure, standards, expertise and tools for moving neuroscience significantly toward FAIR. It is now served by a set of robust international data repositories and scientific gateways specialized for neuroscience data, implementing the vision laid out in the dawn of neuroinformatics for a distributed ecosystem of repositories. The first inroads have been made in establishing FAIR practices and supporting infrastructure in the lab to manage data in a way that smooths the transition between private, semi-private, and public sharing. As best practices for FAIR are articulated, tested, and shared, we can expect that the quality of both the databases and the data will continue to improve.
A federated system allows neuroscience infrastructure to respond more rapidly to new data types and technologies as they are developed. While there are more resources to be sustained, there are also more resources from which to draw should a repository need to be decommissioned. We see from the last 20 years that there is movement in the repository landscape, with some resources ceasing operations, but others merging or changing ownership. As repositories start to align around sets of core features, both interoperability and flexibility will be increased, providing some measure of stability in an otherwise dynamic ecosystem.
While the distributed nature of neuroscience infrastructure brings many benefits, there are concomitant challenges it imposes on both those who submit their data and those that wish to use it. As the motivations and incentives for these two user groups can differ (Subash et al., 2023), balancing the efforts required to submit vs. reuse data will need to be a priority. Until these are addressed, neuroscience will not be considered a fully FAIR discipline:
• Findable: We still do not have an effective query system over the ecosystem of neuroscience data, that allows for aggregation relevant data distributed across multiple repositories. Important steps have been taken by IBI, INCF and CONP, but these efforts will need support if they are to be fully realized.
• Accessible: Users are increasingly acquiring multimodal datasets that may require deposition in multiple repositories. Currently, that requires a user to navigate multiple repositories, set up multiple accounts, entering the same metadata repeatedly and creating the necessary linkages across the different parts of the dataset (Subash et al., 2023). Some work is underway in the US BRAIN Initiative BICCN and BICAN projects to create a more unified workflow including a centralized registry, but such a service would be useful across all neuroscience. Many repositories are starting to implement login and authorization via ORCID, making it easier for users to work across multiple repositories.
• Interoperable: In a distributed system, interoperability is not just about the data but also about the infrastructures. Working across multiple repositories means working across multiple front ends, back ends and data access policies. As core sets of features are described for data repositories, neuroscience infrastructure may also start to converge on certain design patterns that make it easier for users to work across them. A term was introduced in an NIH Workshop on a FAIR Data Ecosystem for Generalist Repositories: coopetition (NIH workshop on the role of generalist repositories to enhance data discoverability and reuse: Workshop summary, 2012). Repositories can compete on certain features to encourage innovation, but there should be a set of features that are shared across repositories and work similarly.
• At the same time, competition among different data providers also can lead to a decrease in data interoperability, as repositories must compete for users. Thus, many repositories lower their requirements for standards compliance (Subash et al., 2023) recommending rather than requiring standards so as to lower the barrier of data submission. Instead of making compliance optional, neuroscience repositories should work on improving their customer service, providing both human and tool support to make it easier for researchers to comply with standards. SPARC has taken this approach, employing customer-oriented curators who assist researchers to comply with SPARC standards. SPARC also developed the SODA tool directed toward researchers with few computational skills to guide and support them in organizing and uploading their files according to the SPARC SDS (Bandrowski et al., 2021). In this way, the burden on the submitter is lessened, while data quality and standards compliance are not sacrificed.
• Reusable: Despite FAIR, most neuroscience data is still very difficult to use. Different projects have devoted different amounts of resources to curation of data and quality control. Generally curated data is of higher quality because it is more completely documented and some QC is performed (Gonçalves and Musen, 2019). Particularly with the push to make data AI/ML ready, funders should be prepared to support curation services for the near future, to ensure that high quality data are available. Such investments will likely not be needed forever; indeed, labs are at this moment experimenting with tools such as ChatGPT to help with query and harmonization. However, investments now in well curated data can help to accelerate training of these types of algorithms, while at the same time, making high quality data immediately available for discovery science.
Finally, usability is not simply a matter of technology or documentation. As Eke et al. (2022) and (Fothergill et al., 2019) have noted, the international nature of neuroscience infrastructure also means that issues of transferring data across national borders, i.e., international data governance, also must be addressed. Federation across distributed databases provides a model that can minimize data governance issues, as the data can remain in place, while compute is brought to the data (Poline et al., 2023).
The good news is that routine data sharing, if not exactly easy, is now at least possible across the sizes and complexities of neuroscience data. Islands of interoperation are starting to emerge among these different resources promoting federated search and shared computational platforms and services. Those of us who were involved from the beginning in attempts to “database the brain” cannot help but be impressed with how far neuroscience sharing and infrastructure has come, even as there is still quite a way to go. As the paradigm continues to shift toward open and effective data sharing in neuroscience, we will fulfill the early vision of neuroinformatics as a driver for “..a new depth of understanding of how the nervous system works in both health and disease.” (Koslow, 2000).
Author contributions
MM: Writing – original draft, Writing – review & editing.
Funding
The author(s) declare financial support was received for the research, authorship, and/or publication of this article. MM is supported by grants from NIH Office of the Director OT2OD030541 for the SPARC Knowledge Management and Curation Core and the US BRAIN Initiative grant U24MH130919.
Acknowledgments
I would like to thank my colleagues Anita Bandrowski and Mathew Abrams for their helpful comments.
Conflict of interest
MM is a founder and board member of SciCrunch Inc., which develops tools and services around rigor and reproducibility.
The author(s) declared that they were an editorial board member of Frontiers, at the time of submission. This had no impact on the peer review process and the final decision.
Publisher’s note
All claims expressed in this article are solely those of the authors and do not necessarily represent those of their affiliated organizations, or those of the publisher, the editors and the reviewers. Any product that may be evaluated in this article, or claim that may be made by its manufacturer, is not guaranteed or endorsed by the publisher.
Footnotes
1. ^http://cocomac.g-node.org/
2. ^https://www.incf.org/resources/sbps
3. ^https://bioportal.bioontology.org/
4. ^https://www.ebi.ac.uk/ols/index
References
Abrams, M. B., Bjaalie, J. G., Das, S., Egan, G. F., Ghosh, S. S., Goscinski, W. J., et al. (2021). A standards Organization for Open and FAIR neuroscience: the international Neuroinformatics coordinating facility. Neuroinformatics. 20, 25–36. doi: 10.1007/s12021-020-09509-0
Almeida, C. A., Abel Torres-Espin, J., Huie, R., Sun, D., Noble-Haeusslein, L. J., Young, W., et al. (2022). Excavating FAIR data: the case of the multicenter animal spinal cord injury study (MASCIS), blood pressure, and neuro-recovery. Neuroinformatics 20, 39–52. doi: 10.1007/s12021-021-09512-z
Alter, G., Gonzalez-Beltran, A., Ohno-Machado, L., and Rocca-Serra, P. (2020). The data tags suite (DATS) model for discovering data access and use requirements. GigaScience 9. doi: 10.1093/gigascience/giz165
Amunts, K., Lepage, C., Borgeat, L., Mohlberg, H., Dickscheid, T., Rousseau, M.-É., et al. (2013). BigBrain: an ultrahigh-resolution 3D human brain model. Science 340, 1472–1475. doi: 10.1126/science.1235381
Assante, M., Candela, L., Castelli, D., and Tani, A. (2016). Are scientific data repositories coping with research data publishing? Data Sci. J. 15, 1–24. doi: 10.5334/dsj-2016-006
Attwood, T. K., Agit, B., and Ellis, L. B. M. (2015). Longevity of biological databases. EMBnet.journal 21:803. doi: 10.14806/ej.21.0.803
Bahim, C., Casorrán-Amilburu, C., Dekkers, M., Herczog, E., Loozen, N., Repanas, K., et al. (2020). The FAIR data maturity model: an approach to harmonise FAIR assessments. Data Sci. J. 19, 1–7. doi: 10.5334/dsj-2020-041
Bilder, Geoffrey, Lin, Jennifer, and Neylon, Cameron. (2015). “Principles for open scholarly infrastructures.” Science in the Open. Available at: https://cameronneylon.net/blog/principles-for-open-scholarly-infrastructures/.
Bandrowski, A., Grethe, J. S., Pilko, A., Gillespie, T., Pine, G., Patel, B., et al. (2021). SPARC Data Structure: Rationale and Design of a FAIR Standard for Biomedical Research Data. bioRxiv. doi: 10.1101/2021.02.10.430563
Botvinik-Nezer, R., Holzmeister, F., Camerer, C. F., Dreber, A., Huber, J., Johannesson, M., et al. (2020). Variability in the analysis of a single neuroimaging dataset by many teams. Nature 582, 84–88. doi: 10.1038/s41586-020-2314-9
Bush, K. A., Calvert, M. L., and Kilts, C. D. (2022). Lessons learned: a neuroimaging research Center’s transition to open and reproducible science. Front. Big Data 5:988084. doi: 10.3389/fdata.2022.988084
Button, K. S., Ioannidis, J. P. A., Mokrysz, C., Nosek, B. A., Flint, J., Robinson, E. S. J., et al. (2013). Power failure: why small sample size undermines the reliability of neuroscience. Nature Rev. Neurosci. Advan. 14, 365–376. doi: 10.1038/nrn3475
Cachat, J., Bandrowski, A., Grethe, J. S., Gupta, A., Astakhov, V., Imam, F., et al. (2012). A survey of the neuroscience resource landscape: perspectives from the neuroscience information framework. Int. Rev. Neurobiol. 103, 39–68. doi: 10.1016/B978-0-12-388408-4.00003-4
Dempsey, W., Foster, I., Fraser, S., and Kesselman, C. (2022). Sharing begins at home: how continuous and ubiquitous FAIRness can enhance research productivity and data reuse. Harvard Data Sci. Rev. 4. doi: 10.1162/99608f92.44d21b86
Eke, D. O., Bernard, A., Bjaalie, J. G., Chavarriaga, R., Hanakawa, T., Hannan, A. J., et al. (2022). International data governance for neuroscience. Neuron 110, 600–612. doi: 10.1016/j.neuron.2021.11.017
Ferguson, A. R., Nielson, J. L., Cragin, M. H., Bandrowski, A. E., and Martone, M. E. (2014). Big data from small data: data-sharing in the ‘long tail’ of neuroscience. Nat. Neurosci. 17, 1442–1447. doi: 10.1038/nn.3838
Fothergill, B. T., Knight, W., Stahl, B. C., and Ulnicane, I. (2019). Responsible data governance of neuroscience big data. Front. Neuroinform. 13:28. doi: 10.3389/fninf.2019.00028
Fouad, K., Vavrek, R., Surles-Zeigler, M. C., Huie, J. R., Radabaugh, H., Gurkoff, G. G., et al. (2023). A practical guide to data management and sharing for biomedical laboratory researchers. Zenodo. doi: 10.5281/zenodo.8206341
“Funders’ Policies.” (2015). Available at: https://www.data.cam.ac.uk/funders.
Gardner, D., Akil, H., Ascoli, G. A., Bowden, D. M., Bug, W., Donohue, D. E., et al. (2008). The neuroscience information framework: a data and knowledge environment for neuroscience. Neuroinformatics 6, 149–160. doi: 10.1007/s12021-008-9024-z
Gillespie, T. H., Tripathy, S. J., Sy, M. F., Martone, M. E., and Hill, S. L. (2022). The neuron phenotype ontology: a FAIR approach to proposing and classifying neuronal types. Neuroinformatics. 20, 793–809. doi: 10.1007/s12021-022-09566-7
Gonçalves, R. S., and Musen, M. A. (2019). The variable quality of metadata about biological samples used in biomedical experiments. Scientific Data 6:190021. doi: 10.1038/sdata.2019.21
Gorgolewski, K. J., Auer, T., Calhoun, V. D., Cameron Craddock, R., Das, S., Duff, E. P., et al. (2016). The brain imaging data structure, a format for organizing and describing outputs of neuroimaging experiments. Scientific Data 3:160044. doi: 10.1038/sdata.2016.44
“Governance.” (n.d.). Brain imaging data structure. Available at: https://bids.neuroimaging.io/governance.
Grethe, J. S., Baru, C., Gupta, A., James, M., Ludaescher, B., Martone, M. E., et al. (2005). Biomedical informatics research network: building a National Collaboratory to hasten the derivation of new understanding and treatment of disease. Stud. Health Technol. Inform. 112, 100–109.
Hamilton, D. J., Shepherd, G. M., Martone, M. E., and Ascoli, G. A. (2012). An ontological approach to describing neurons and their relationships. Front. Neuroinform. 6:15. doi: 10.3389/fninf.2012.00015
Hawrylycz, M., Baldock, R. A., Burger, A., Hashikawa, T., Johnson, G. A., Martone, M. E., et al. (2011). Digital Atlasing and standardization in the mouse brain. PLoS Comput. Biol. 7:e1001065. doi: 10.1371/journal.pcbi.1001065
Hawrylycz, M., Boline, J., Burger, A., Hashikawa, T., Johnson, G. A., Martone, M. E., et al. (2009). “The INCF digital Atlasing program: report on digital Atlasing standards in the rodent brain.” Available at: http://precedings.nature.com/documents/4000/version/1.
Hawrylycz, M., Martone, M. E., Ascoli, G. A., Bjaalie, J. G., Dong, H.-W., Ghosh, S. S., et al. (2023). A guide to the BRAIN initiative cell census network data ecosystem. PLoS Biol. 21:e3002133. doi: 10.1371/journal.pbio.3002133
Hodson, Simon, Jones, Sarah, Collins, Sandra, Genova, Françoise, Harrower, Natalie, Laaksonen, Leif, et al. (2018). Turning FAIR data into reality: Interim report from the European Commission expert group on FAIR data. Amsterdam, Washington, DC: IOS Press.
International Brain Initiative (2020). International brain initiative: an innovative framework for coordinated global brain research efforts. Neuron 105, 212–216. doi: 10.1016/j.neuron.2020.01.002
Ioannidis, J. P. A. (2007). Why Most published research findings are false: Author’s reply to Goodman and Greenland. PLoS Med. 4:2. doi: 10.1371/journal.pmed.0040215
Keator, D. B., Helmer, K., Steffener, J., Turner, J. A., Van Erp, T. G. M., Gadde, S., et al. (2013). Towards structured sharing of raw and derived neuroimaging data across existing resources. NeuroImage 82, 647–661. doi: 10.1016/j.neuroimage.2013.05.094
Kennedy, D. N., Abraham, S. A., Bates, J. F., Crowley, A., Ghosh, S., Gillespie, T., et al. (2019). Everything matters: the ReproNim perspective on reproducible neuroimaging. Front. Neuroinform. 13:1. doi: 10.3389/fninf.2019.00001
Kleven, H., Gillespie, T. H., Zehl, L., Dickscheid, T., Bjaalie, J. G., Martone, M. E., et al. (2023). AtOM, an ontology model to standardize use of brain atlases in tools, workflows, and data infrastructures. Scientific Data 10:486. doi: 10.1038/s41597-023-02389-4
“KnowledgeSpace.” (n.d.). Available at: https://knowledge-space.org/.
Koslow, S. H. (2000). “Should the Neuroscience Community Make a Paradigm Shift to Sharing Primary Data?” Nature Neuroscience 3: 863–65.
Larson, S. D., and Martone, M. E. (2013). NeuroLex.org: an online framework for neuroscience knowledge. Front. Neuroinform. 7:18. doi: 10.3389/fninf.2013.00018
Lin, D., Crabtree, J., Dillo, I., Downs, R. R., Edmunds, R., Giaretta, D., et al. (2020). The TRUST principles for digital repositories. Scientific Data 7:144. doi: 10.1038/s41597-020-0486-7
Martone, Maryann E., and Nakamura, Richard. (2022). “Changing the culture on data management and sharing: overview and highlights from a workshop held by the National Academies of sciences, engineering, and medicine.” Available at: https://hdsr.mitpress.mit.edu/pub/p1xu0son/release/1?readingCollection=b697ca32.
Martone, M. E., Gupta, A., and Ellisman, M. H. (2004). E-neuroscience: challenges and triumphs in integrating distributed data from molecules to brains. Nat. Neurosci. 7, 467–472. doi: 10.1038/nn1229
Miller, J. A., Gouwens, N. W., Tasic, B., Collman, F., van Velthoven, C. T. J., Bakken, T. E., et al. (2020). Common cell type nomenclature for the mammalian brain. elife 9. doi: 10.7554/eLife.59928
Mungall, C. J., Torniai, C., Gkoutos, G. V., Lewis, S. E., and Haendel, M. A. (2012). Uberon, an integrative multi-species anatomy ontology. Genome Biol. 13:R5. doi: 10.1186/gb-2012-13-1-r5
Murphy, F., Bar-Sinai, M., and Martone, M. E. (2021). A tool for assessing alignment of biomedical data repositories with open, FAIR, citation and trustworthy principles. PLoS One 16:e0253538. doi: 10.1371/journal.pone.0253538
National Academies of Sciences, Engineering, and Medicine (2018). Open Science by design National Academies of Sciences, Engineering, and Medicine. Washington DC. doi: 10.17226/25116
Nelson, Alondra. (2022). Office of Science and Technology Policy (OSTP). Letter to Heads of us Executive Departments and Agencies. “08-2022-OSTP-public-access-memo.Pdf,” August 25, 2022.
“Neuroshapes.” (n.d.). Accessed August 4, 2023. Available at: http://neuroshapes.org/.
“NIH workshop on the role of generalist repositories to enhance data discoverability and reuse: Workshop summary.” (2012). NIH. Available at: https://datascience.nih.gov/data-ecosystem/nih-data-repository-workshop-summary.
Ozyurt, I. B., Grethe, J. S., Martone, M. E., and Bandrowski, A. E. (2016). Resource Disambiguator for the web: extracting biomedical resources and their citations from the scientific literature. PLoS One 11:e0146300. doi: 10.1371/journal.pone.0146300
Papp, E. A., Leergaard, T. B., Evan Calabrese, G., Johnson, A., and Bjaalie, J. G. (2014). Waxholm space atlas of the Sprague Dawley rat brain. NeuroImage 97, 374–386. doi: 10.1016/j.neuroimage.2014.04.001
Piekniewska, A., Haak, L. L., Henderson, D., McNeill, K., Bandrowski, A., and Seger, Y. (2023). Establishing an early Indicator for data sharing and reuse. Piekniewsk, doi: 10.31219/osf.io/ryxg2
Poldrack, Russell A., Markiewicz, Christopher J., Appelhoff, Stefan, Ashar, Yoni K., Auer, Tibor, Baillet, Sylvain, et al. (2023). “The past, present, and future of the brain imaging data structure (BIDS).” arXiv [q-bio.OT]. arXiv. Available at: http://arxiv.org/abs/2309.05768.
Poline, J.-B., Das, S., Glatard, T., Madjar, C., Dickie, E. W., Lecours, X., et al. (2023). Data and tools integration in the Canadian open neuroscience platform. Scientific Data 10:189. doi: 10.1038/s41597-023-01946-1
Quaglio, G., Toia, P., Moser, E. I., Karapiperis, T., Amunts, K., Okabe, S., et al. (2021). The international brain initiative: enabling collaborative science. Lancet Neurol. 20, 985–986. doi: 10.1016/S1474-4422(21)00389-6
Ropelewski, A. J., Rizzo, M. A., Swedlow, J. R., Huisken, J., Osten, P., Khanjani, N., et al. (2022). Standard metadata for 3D microscopy. Scientific Data 9:449. doi: 10.1038/s41597-022-01562-5
Rübel, O, Andrew, T, Ryan, Ly, Benjamin, K., et al. (2022). “The Neurodata Without Borders Ecosystem for Neurophysiological Data Science.” eLife 11 (October). doi: 10.7554/eLife.7836
Sandström, Malin, Abrams, Mathew, Bjaalie, Jan, Hicks, Mona, Kennedy, David, et al. (2022). “Recommendations for repositories and scientific gateways from a neuroscience perspective.” arXiv [cs.CY]. arXiv. Available at: http://arxiv.org/abs/2201.00727.
Sansone, S.-A., McQuilton, P., Cousijn, H., Cannon, M., Chan, W. M., Callaghan, S., et al. (2020). Data repository selection: criteria that matter. doi: 10.5281/zenodo.4084763
Shearer, Kathleen. (n.d.). “COAR community framework for best practices in repositories.” Accessed April 3, 2021. Available at: https://www.coar-repositories.org/news-updates/coar-community-framework-for-best-practices-in-repositories/.
Shepherd, G. M., Marenco, L., Hines, M. L., Migliore, M., McDougal, R. A., Carnevale, N. T., et al. (2019). Neuron names: a gene-and property-based name format, with special reference to cortical neurons. Front. Neuroanat. 13:25. doi: 10.3389/fnana.2019.00025
Stall, S., Martone, M. E., Chandramouliswaran, I., Federer, L., Gautier, J., Gibson, J., et al. (2023). Generalist Repository Comparison Chart. doi: 10.5281/zenodo.7946938
Subash, P., Gray, A., Boswell, M., Cohen, S. L., Garner, R., Salehi, S., et al. (2023). A comparison of Neuroelectrophysiology databases. ArXiv 10:719. doi: 10.1038/s41597-023-02614-0
Surles-Zeigler, M. C., Sincomb, T., Gillespie, T. H., de Bono, B., Bresnahan, J., Mawe, G. M., et al. (2021). Extending and Using Anatomical Vocabularies in the Stimulating Peripheral Activity to Relieve Conditions (SPARC) Project. bioRxiv. doi: 10.1101/2021.11.15.467961
Tan, S. Z., Kai, H. K., Aevermann, B. D., Gillespie, T., Harris, N., Hawrylycz, M. J., et al. (2023). Brain data standards - a method for building data-driven cell-type ontologies. Scientific Data 10:50. doi: 10.1038/s41597-022-01886-2
Torres-Espín, A., Haefeli, J., Ehsanian, R., Torres, D., Almeida, C. A., Russell Huie, J., et al. (2021). Topological network analysis of patient similarity for precision Management of Acute Blood Pressure in spinal cord injury. elife 10. doi: 10.7554/eLife.68015
Weiner, M. W., Aisen, P. S., Jack Jr, C. R., Jagust, W. J., Trojanowski, J. Q., Shaw, L., et al. (2010). The Alzheimer’s Disease Neuroimaging Initiative: Progress report and future plans. Alzheimers Dement. 6, 202–11.e7. doi: 10.1016/j.jalz.2010.03.007
Wilkinson, M. D., Michel Dumontier, I., Aalbersberg, J. J., Appleton, G., Axton, M., Baak, A., et al. (2016). The FAIR guiding principles for scientific data management and stewardship. Scientific Data 3:160018. doi: 10.1038/sdata.2016.18
Glossary
Keywords: data sharing, neuroinformatics, data bases, FAIR (findable accessible interoperable and reusable) principles, data management, incf
Citation: Martone ME (2024) The past, present and future of neuroscience data sharing: a perspective on the state of practices and infrastructure for FAIR. Front. Neuroinform. 17:1276407. doi: 10.3389/fninf.2023.1276407
Edited by:
Maaike M. H. Van Swieten, Netherlands Comprehensive Cancer Organisation (IKNL), NetherlandsReviewed by:
Leonardo Candela, National Research Council (CNR), ItalyAlexandre Rosa Franco, Nathan Kline Institute for Psychiatric Research, United States
Copyright © 2024 Martone. This is an open-access article distributed under the terms of the Creative Commons Attribution License (CC BY). The use, distribution or reproduction in other forums is permitted, provided the original author(s) and the copyright owner(s) are credited and that the original publication in this journal is cited, in accordance with accepted academic practice. No use, distribution or reproduction is permitted which does not comply with these terms.
*Correspondence: Maryann E. Martone, mmartone@ucsd.edu