Abstract
Ionospheric indices give information about ionospheric perturbations, which may cause absorption, diffraction, refraction, and scattering of radio signals, including those from global navigation satellite systems (GNSS). Therefore, there may be a relationship between index values and GNSS positioning results. A thorough understanding of ionospheric indices and their relationship to positioning results can help monitor and forecast the reliability and accuracy of GNSS positioning and support the precision and safety of life applications. In this study, we present the relationship between three indices: Gradient Ionosphere indeX (GIX), Sudden Ionospheric Disturbance indeX (SIDX), and Rate of Total electron content Index (ROTI) in relation to precise positioning results. We used two approaches: precise point positioning (PPP) and relative positioning for long baselines. We focus on GNSS stations located in Europe for two selected geomagnetic storms: March 17, 2015, and May 22, 2015. Our results show that in the case of PPP, positioning degradation occurred mainly at high latitudes and was mostly caused by rapid small-scale changes in ionospheric electron content represented by SIDX and ROTI. We also showed a significant correlation between cycle slips of GNSS signals and ROTI (0.88). The most significant degradations for relative positioning for low and medium latitudes were associated with large spatial gradients reflected by the GIX.
Similar content being viewed by others
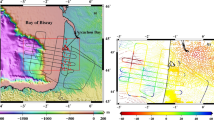
Introduction
The ionospheric plasma affects the propagation of radio waves, causing changes in the signal’s polarization, phase, and amplitude due to absorption, diffraction, refraction, and scattering. These effects also apply to signals received from global navigation satellite systems (GNSS), negatively affecting the quality of positioning results. The connection between the occurrence of ionospheric disturbances and a decrease in the accuracy of precise positioning, both relative and absolute, has been the subject of numerous studies. An early study was conducted by Mayer et al. (2009) using data from a dense GNSS network over Germany. They investigated spatial and temporal gradients caused by ionospheric disturbances and found that sudden variations in the total electron content (TEC) could potentially reduce the integrity and availability of the Ground-Based Augmentation System (GBAS), which is mainly used by aircrafts. Moreno et al. (2011) showed that TEC variations observed in the equatorial region cause significant degradation of dual-frequency positioning. Marques et al. (2018) linked the decrease in precise point positioning (PPP) accuracy with losses of signal lock and cycle slips effects caused by the scintillations. Other studies also reported similar findings (Lu et al. 2020; Valdés-Abreu et al. 2022). Luo et al. (2018a) also found that single and dual-frequency PPP are degraded during geomagnetic storm periods. They showed a clear relationship between the Rate of TEC Index (\(ROTI\)) and position errors for high-latitude stations. Similar conclusions were drawn by Nie et al. (2022), who showed that large ROTI values for high-latitude regions cause PPP errors related to cycle slips. For low-latitudes, similar studies were conducted by Rodríguez-Bilbao et al. (2015), Luo et al. (2018b; 2020), Guo et al. (2019), and Li et al. (2022). Wielgosz et al. (2005) presented that large ionospheric gradients could prevent proper modeling of the ionospheric delay in relative positioning, causing problems with on-the-fly ambiguity resolution and decreasing positioning accuracy. Similar conclusions were reported by Arlsan and Demirel (2008). They found that large temporal gradients negatively affect the positioning and ambiguity resolution success rates during high geomagnetic activity, especially for the baselines located at high latitudes. That was also confirmed by Jacobsen and Andalsvik (2016), who investigated positioning errors of the real-time kinematic (RTK) and PPP approaches. They found that errors increased rapidly during the geomagnetic storm. However, results for PPP gave more precise results. It is worth mentioning that not only ionospheric disturbances caused by a geomagnetic storm can affect GNSS positioning. Carter et al. (2023) showed that large- and medium-scale traveling ionospheric disturbances from the Hunga Tonga volcano eruption influenced the positioning of PPP in Australia. Yu and Liu (2021) presented a decrease in positioning accuracy due to ionospheric disturbances caused by tropical cyclone-related atmospheric gravity waves.
Since ionospheric disturbances may cause positioning degradation, there is a need to describe the ionospheric state in the best possible way using perturbation indices that can be useful for monitoring and mitigating ionospheric impact on GNSS measurements. The most used ionospheric index is, previously mentioned, \(ROTI\) (Pi et al. 1997). It is a GNSS-based index developed for detecting and measuring ionospheric irregularities and characterization of GNSS phase oscillations. To describe the scintillation of the amplitude and phase of the GNSS radio signals while passing through the ionosphere, scintillation indices like S4 and \({\sigma }_{\varphi }\) are commonly used (Forte and Radicella 2002; Kintner et al. 2007; Hlubek et al. 2014). \(ROTI\) can also measure phase scintillations (van der Meeren et al. 2015; Clausen et al. 2016; Yang and Liu 2016; Olwendo et al. 2018). A helpful index for investigating disturbed periods affecting GNSS positioning is the Along Arc TEC Rate (\(AATR\)), which give information to the Space-Based Augmentation System (SBAS) users about availability anomalies (Juan et al. 2018). In Jakowski et al. 2012 introduced Disturbance Ionosphere indeX (\(DIX\)), a dual-frequency-based robust and objective ionospheric index, which can properly characterize small and medium scales temporal and spatial ionospheric variations. In 2019, Jakowski and Hoque, expanded this index by proposing the Gradient Ionosphere indeX (\(GIX\)) and Sudden Ionospheric Disturbance indeX (\(SIDX\)) to investigate spatial and temporal characteristics of ionospheric perturbations better. A comprehensive description of various types of ionospheric indices can be found in Borries et al. (2020).
We present for the first time a comparison of three indices: \(GIX\), \(SIDX\), and \(ROTI\), with the results of precise positioning. We focus on the PPP method and the relative positioning of long baselines in Europe. We discuss the occurrence of the errors and their connection with the analyzed indices. We also discuss the possible causes of positioning degradation. The presented results better explain the relationship between indices and precise positioning errors.
Data and methodology
In our study, we used about 350 GNSS stations located in Europe belonging to the EUREF Permanent GNSS Network (EPN). The computations were performed using 30-s GPS observations. We used two precise positioning approaches: dual-frequency PPP and long baseline relative positioning. In the first case, the position of all stations was calculated using gLAB software (Hernández-Pajares et al. 2010) with precise ephemerides and clocks from the International GNSS Service (IGS). For the relative positioning, we selected representative baselines and computed them using three different approaches: (a) single-frequency positioning with the Klobuchar model (hereinafter named L1 broadcast), (b) single-frequency positioning with global maps of the ionosphere from IGS (L1 IONEX), (c) dual-frequency positioning where the ionospheric delay was eliminated by the ionospheric-free linear combination (Iono-free). We used the final IGS ionospheric product, which has a resolution of 5° (longitude) by 2.5° (latitude) and is 2 h in time. All calculations were performed by applying the kinematic method using the RTKLIB software (Takasu and Yasuda 2009).
Earlier studies (Moreno et al. 2011; Luo et al. 2018b; Poniatowski and Nykiel 2020) show that significant electron content variations can cause an increase in the number of cycle slips and, consequently, decrease positioning accuracy. Cycle slip is a discontinuity, apparent as a jump of an integer number of wavelengths in the phase measurement caused by the receiver’s loss of lock. In our study, a cycle slip is considered a single break in phase observations. Both gLAB and RTKLIB consider a loss of lock indicator (LLI) flag stored in the observational files to indicate epochs where cycle slips occurred. They also use a detection method based on geometry-free linear combination. Additionally, gLAB uses an approach based on the Melbourne-Wübbena linear combination (Blewitt 1990). RTKLIB also uses the differences between the phase and Doppler measurements on both frequencies.
To assess the relationship between the obtained positioning results and ionospheric perturbations, we chose three different indices. First, we used \(ROTI\), which is calculated for each time step \(t\), as a standard deviation of Rate of TEC (\(ROT\)) for the assumed time window \(N\):
where \(ROT\) is the \(VTEC\) differences between two epochs divided by the sampling interval (\(\Delta t\)):
both \(ROTI\) and \(ROT\) are expressed in TECU/min. In practice, \(ROTI\) is determined over a 5-min window in the case of 30-s measurements and over a 1-min window in the case of 1-s observations, which translates into \(N\) values of 10 and 60, respectively.
In addition, we used two indices introduced by Jakowski and Hoque (2019): \(GIX\) and \(SIDX\). \(GIX\) was developed to provide the magnitude of spatial changes of \(VTEC\) in a selected area. It can be written as follows:
where \(\nabla VTEC\) denotes the value of the \(VTEC\) gradient between two ionospheric pierce points (IPPs); \({\nabla VTEC}_{X}\), \({\nabla VTEC}_{X}\) are zonal and meridional gradients, respectively. \(\langle \rangle\) means average of all values within a specified area. The \(GIX\) unit is mTECU/km. In our study, \(\nabla VTEC\) values were estimated only between IPPs for the same satellite and within the range between 50 and 500 km.
\(SIDX\), dedicated to describing \(VTEC\) time changes and expressed in mTECU/sec, can be understood as the instantaneous average value of \(ROT\) in a given region representing the temporal changes of \(VTEC\) in the considered area:
In analogy to geomagnetic or solar indices, ionospheric indices can help to detect, monitor, and study the structure and dynamics of ionospheric disturbances. SIDX is calculated on the basis of only two consecutive epochs, which makes this index sensitive to average temporal variations. Thanks to this, SIDX is well suited to instantaneously detect solar flare events at different intensity levels, as shown by Jakowski and Hoque (2019). The solar flares can significantly affect GNSS positioning, as shown by Berdermann et al. (2018). ROTI is computed using a time window of observations. By aggregating measurements up to several minutes, ROTI represents a mixture of ionospheric perturbations: temporal variations and small-scale spatial irregularities. On the other hand, GIX is an index that describes the characteristics of spatial gradients that are part of ionospheric disturbances. GIX is able to detect large scale ionization fronts, e.g., during sunrise in the east–west direction at mid to low latitudes. GIX can also provide information on the amplitude and direction of the front, e.g., ionization fronts propagating to lower latitudes caused by the strong solar wind induced disturbances at high latitudes (Jakowski and Hoque 2019). With early knowledge of the direction and velocity of the ionization front, it is possible to warn users in advance of the front's arrival.
All investigated indices were estimated using self-developed scripts. We used dual-frequency 30-s GPS observations from GNSS reference stations belonging to the EPN network. All indices were computed with an interval equal to the observations. For \(ROTI\), we used a 5-min moving window to aggregate enough observations. For \(GIX\), calibrated \(VTEC\) values were obtained using the methodology proposed by Ma and Maruyama (2003).
For our analyses, we chose two geomagnetic storms, which differ in characteristics: March 17–18, 2015, and June 22–23, 2015. Both storms are among the strongest during the solar cycle 24. They are characterized by the geomagnetic activity index Kp as 8.0, and ionospheric disturbance index Dst with peaks as − 223 and -195 nT, respectively.
Results
Here, we show the results of the calculations. Firstly, we present \(GIX\), \(SIDX\), and \(ROTI\) estimation for the analyzed geomagnetic storms. Next, we present results and statistics for dual-frequency PPP. Finally, we show the coordinates variations for selected baselines estimated using different strategies of relative positioning. We also discuss the relationship between positioning degradation and ionospheric indices.
GIX, SIDX, and ROTI
The values of \(\nabla VTEC\) and \(ROT\) have a large spatial variation. Calculation of the \(GIX\) and \(SIDX\) parameters for the entire area of Europe would imply that these indices would not reflect the state of the ionosphere in details. Therefore, for further analysis, we divided the area into three equal latitude zones: 30° N–45° N, 45° N–60° N, and 60° N–75° N. In each case, the longitude is limited to between 15° W and 35° E. The results of \(GIX\), \(SIDX\), and \(ROTI\) for the three zones for both analyzed geomagnetic storms are presented in Fig. 1. Besides, we also show 95-percentile of occurrences for each zone.
Both storms show high values of \(GIX\), \(SIDX\) and \(ROTI\) for high latitudes. Disturbances during the main phase of the storm in March 2015 lasted from 16:00 to approximately 22:00. At that time, \(GIX\) values were up to 20 mTECU/km (\({GIX}_{95}\) around 45 mTECU/km), \(SIDX\) up to 20 mTECU/s (\({SIDX}_{95}\) over 60 mTECU/s), and \(ROTI\) up to 2.2 TECU/min (\({ROTI}_{95}\) around 5 TECU/min). It is worth noting that there are clear peaks in the \(GIX\) and \({GIX}_{95}\) values. At the same time, peaks can also be seen in the \(SIDX\) and \(ROTI\) values. They were caused by particle precipitation which is very common at high latitudes during the geomagnetic storm. It causes spatial and temporal variations, which are very well reflected through the analyzed indices. However, the magnitude of small-scale changes are higher than spatial gradients in our cases.
The situation is different for the middle zone (45° N–60° N). There is a clear peak in the \(GIX\) value of around 25 mTECU/km and the \({GIX}_{95}\) around 55 mTECU/km being larger than those for high latitudes. At the same time, the \(SIDX\) and \(ROTI\) values are smaller compared to high latitude values. Therefore, for this zone, we recorded very high values of spatial gradients, the maximum of which was at 18:00. For the low-latitude zone (30°N-45°N), we did not observe significant \(SIDX\) and \(ROTI\) values. On the other hand, \(GIX\) reached values of about 15 mTECU/km, which suggests the occurrence of moderate spatial gradients, but no sharp changes in values are visible here. For the storm in June 2015, it is seen that storm’s active phase was shorter. The greatest disturbances are observed between 18:00 and 30:00 (Fig. 1 bottom). At high latitudes, the magnitudes of \(SIDX\) and \(ROTI\) were like those of the storm in March. In contrast, the \(GIX\) values were slightly lower. There were also fewer sharp peaks. Unlike the storm in March, we don not see significant mid-latitude disturbance during the June event. \(ROTI\) does not exceed 0.2 TECU/min, and \(GIX\) values do not exceed 10 mTECU/km. It indicates that for the area 45°N-60°N, we found neither significant temporal changes in TEC nor large spatial gradients. The situation is different for the analyzed low latitude region. We can see clear gradients represented by \(GIX\) with a value of 20 mTECU/km, and \({GIX}_{95}\) has peaks of around 55 mTECU/km. In contrast, the \(SIDX\) and \(ROTI\) values do not show significant TEC variations. Only \({ROTI}_{95}\) indicates that there were changes of up to 1.2 TECU/min for several stations.
Precise point positioning
Here we present the results of the PPP in which the dual-frequency measurements eliminate the ionospheric delay. However, many studies (Luo et al. 2018b; Poniatowski and Nykiel 2020) show that this may not be a sufficient approach to avoid positioning degradation during severe geomagnetic storms.
Figure 2 shows averaged topocentric coordinates for stations in each latitude zone. The values were estimated in relation to the reference coordinates from the weekly EPN solution. Thus, the results can be interpreted as positioning errors. Despite using the ionospheric-free combination, positioning degradation is clearly visible, especially for latitudes above 60° N. For this zone, during the geomagnetic storm in March 2015, we obtained an average absolute error of about 0.18, 0.21, and 0.46 m for the North, East, and Up components, respectively. However, during the main phase of this storm, the maximum values reached about 5.67, 6.37, and 23.06 m, respectively. Significant positioning errors continued throughout the storm. For latitude zone 45° N–60° N, the positioning errors were much lower. The maximum values were about 0.18, 0.16, and 0.42 m. It is also seen that the period when the positioning accuracy decreased was limited only to the hours between 15:00 and 18:00. In the low latitude zone, 30°N to 45°N, the magnetic storm has no significant influence on the positioning results. Higher values at the beginning of each day are related only to the initialization time of the PPP method itself.
Dual-frequency precise point positioning (PPP) results for different latitude zones for two geomagnetic storms: March 17, 2015 (left) and June 22, 2015 (right). The lines represent the average positioning error calculated for all stations in the zone. Results presented in the topocentric coordinates: North (blue), East (yellow), and Up (red). The number of stations in each zone is also showed
For stations in the 60° N–75° N sector, we see an apparent increase in positioning errors for all components. The jump in solutions occurred around 13:30. At the same time, there is also a clear jump in the values of \(ROTI\) (about 1.5 TECU/min), \({ROTI}_{95}\) (about 5 TECU/min), \(SIDX\)(± 10 mTECU/sec), and \({SIDX}_{95}\) (more than 60 mTECU/sec). By comparing Figs. 1 and 2, we see that the increase in errors occurred exactly in the periods of \(SIDX\) and \(ROTI\) growth and lasted from 13:30 to 23:00. It did not fully coincide with the \(GIX\) distribution, where we observe an increase in values from 10:00. However, at 13:00 clear and sudden jumps related to ionospheric patches are also visible in the \(GIX\). Therefore, the obtained results indicate that the discussed indices can successfully detect the ionospheric irregularities/gradients (in this case high latitude patches) that cause the degradation of the position solutions. In general, ionospheric irregularities/gradients negatively affect GNSS observations and reduce their quality (rapid fluctuations in signal amplitudes and phases, which can cause even cycle slips and thus loss of signal lock).
For the latitude zone 45° N–60°N, positioning errors are related to the small-scale TEC variation, evidenced by the fact that we see at the same time (15:00–18:00) increased values of \(SIDX\) and \(ROTI\). There is no clear peak in the positioning error related to the large \(GIX\) value at 18:00.
For the storm in June 2015, we obtained a similar distribution of results. The largest average positioning errors, reaching about 2.87, 3.67, and 11.13 m, for the North, East, and Up components, respectively, were obtained for the 60° N–75° N zone. The positioning results clearly show that the storm had a smaller impact on the error values and the duration of the positioning degradation. Nevertheless, most of the time, we still see significant jumps of several meters in accuracy, especially for the altitude component. For zones 30° N–45° N and 45° N–60° N, the average errors did not exceed 0.06, 0.18, and 0.32 m for the North, East, and Up components, respectively. These highest values occurred only for a short period in the evening hours of June 22.
The relationship between the ionospheric perturbation index and positioning errors is similar to the March storm. Significant positioning degradation occurred at the time of large \(SIDX\) and \(ROTI\) changes. This is mainly true for the high-latitude zone, while no large temporal variations were noticed for mid- and low-latitude regions. Even significant spatial gradients between 20:00 and 24:00 did not cause an increase in errors.
Relative positioning
For the date of March 17, we chose two baselines: one between stations POTS (Potsdam, Germany) and AUBG (Augsburg, Germany), and the other between the POTS and DELF (Delft, Netherlands). The first, about 485 km long, is approximately parallel to the direction of the \(VTEC\) gradients. The second baseline is 595 km long, lying perpendicular to the direction of the \(\nabla VTEC\) (Fig. 3 left). The stations were selected at locations where large spatial gradients occurred. However, we did not choose the baseline from the high latitude to avoid significant degradation for the single station (presented above), which could affect the estimation of the baseline distance.
Figure 4 shows the positioning results for the baselines during the geomagnetic storm on March 17, 2015. For better visualization of the results, we show only the values of differences from the average length of the baseline in the North, East, and Up directions. The figure shows three solutions: L1 broadcast (cyan line), L1 IONEX (green line), and Iono-free (red line). The statistics of the positioning results are presented in Table 1.
Figure 4 (left) presents positioning errors for the POTS-DELF baseline. We see an evident degradation of positioning during the active storm phase between 06:00 and 24:00. We obtained the highest error values for the L1 broadcast solution. They amounted to a maximum of about −3.46, −4.21, and −4.88 m for the North, East, and Up components. The L1 IONEX solution had maximum errors of about 2.03, −1.59, and 3.12 m. For comparison, the Iono-free solution, treated as a reference, had the largest errors of about 2.11, 2.16, and −4.19 m. However, this was only for single jumps between 15:00 and 18:00. When looking without these jumps, the errors were at most as the values of 0.15, 0.13, and 0.41 m for the North, East, and Up components. Standard deviations of the solution L1 IONEX were significantly lower than for the solution with the Klobuchar model. Figure 4 (right) shows the results of relative positioning for the baseline parallel to the direction of the gradients—POTS-AUBG. In this case, the L1 broadcast solution was also characterized by the most significant errors, reaching even about 5.59, 7.20, and −9.26 m for the North, East, and Up components. These values were higher than for the POTS-DELF baseline. Similarly, higher values were also obtained for the standard deviation. The L1 IONEX solution also showed higher error values, but only for the North and Up components. For the eastern direction, it can be assumed that both baselines were characterized by similar precision. The maximum degradations were about −4.72, 1.36, and 4.41 m, and the standard deviations were 0.99, 0.27, and 0.82 m. We also obtained similar statistics for the ionospheric-free solution. It is worth noting that, in this case, the solution was characterized by a smaller number of jumps and a smaller magnitude. The maximum error values were about 1.97, 1.58, and 3.31 m, and the standard deviation was 0.11, 0.09, 0.21 m.
In the second case, we focused on lower latitudes. During the June 22, 2015 storm, TEC had minor temporal but more extensive spatial changes, as presented earlier in Fig. 1. The \(GIX\) index, in this case, reached values of about 20 mTECU/km (\({GIX}_{95}\) about 50 mTECU/km), which was more significant than for higher latitudes. In turn, the \(SIDX\) and \(ROTI\) values were significantly lower. Due to this, we can deduce how such large \(VTEC\) gradients affect the positioning of long baselines. Therefore, we chose two baselines located in Spain: VILL (Villafranca, Spain)–GAIA (Gaia, Portugal) and VILL–MALA (Malaga, Spain). The lengths of these baselines were 398.2 km and 465.8 km, respectively. As in the previous case, these baselines have different directions—almost perpendicular to each other (Fig. 3 right). The positioning results are shown in Fig. 5. For the VILL–GAIA baseline (perpendicular to the direction of the gradients), we see that the positioning results using the Klobuchar model are like those using the IONEX file. In both cases, the maximum errors occurred at similar times. For the North component, the largest errors occurred around midnight and amounted to 5.78 and 6.56 m for the L1 broadcast and L1 IONEX solutions, respectively. For the East component, the degradation of the baseline determination occurred at similar times and amounted to about 4.61 and 3.78 m. In the case of altitude, there are three moments where the most significant decrease in accuracy is seen. The first occurred around 22:30 and resulted in errors of about 10.97 and 13.51 m for L1 broadcast and L1 IONEX; the second, around 24:00, with values of −11.72 and −17.23 m, and the third, around 26:00, where the baseline error reached about −8.24 and −9.64 m. As of 30:00, all solutions have stabilized. For the broadcast solution, the error values did not exceed 0.29, 0.79, and 1.35 m for the North, East, and Up components, respectively. For the solution with the IONEX model, these values were 0.37, 1.00, and 1.67 m. For positioning using the ionospheric-free linear combination, we obtained some decreases in accuracy only during the intense period of the geomagnetic storm. The values of these jumps were the highest for the altitude component and amounted to about 3 m. Beyond that, the maximum error values were only about 0.17, 0.33, and 0.35 m for the North, East, and Up components. The VILL-MALA baseline was located parallel to the direction of gradient propagation. In this case, the positioning errors were clearly different, started earlier, and lasted longer. The period of the greatest positioning degradation occurred from 17:00 and lasted as long as 15 h. During this time, it can be observed that very large, numerous spikes characterized both the L1 broadcast and L1 IONEX solutions. Unlike the previous case, it is difficult to discuss the similarity of these two solutions here. Both showed significant positioning errors, but the distribution and size were different. The maximum errors for the L1 IONEX solution were about 16.58, 7.40, and 21.01 m for the North, East, and Up components. However, these values were much larger for the broadcast solution and amounted to about 47.29, 21.93, and 92.87 m. Also, the two-frequency solution was characterized by more jumps than for the VILL-GAIA baseline. Apart from the jumps, the error values were at a similar level.
Discussion
This section discusses the reasons for the degradation of PPP and relative positioning. We present analyses of the occurrence of cycle slips, determine correlations with ionospheric indices, and analyze the values of ionospheric gradients.
Precise point positioning
To answer the question of why large, temporal changes in \(VTEC\), represented mainly by the \(SIDX\) and \(ROTI\) indices, degraded the positioning results, we analyzed the occurrence of cycle slips. Similarly, we prepared the average value of the cycle slip occurrence for the positioning results as a function of time for individual sectors. We see that the results presented in Fig. 6 coincide with the results of positioning in Fig. 2, i.e., an increase in the number of cycle slips causes an increase in positioning errors. The highest number of cycle slips occurs for the highest latitudes, reaching about 1.5 cycle slips per station. Dealing with cycle slips requires resolving the ambiguity from the beginning or repairing it. The first approach causes a decrease in the quality of positioning, especially when the determination needs to be made for many satellites simultaneously. The second option is not easy and often requires complicated algorithms, which are not always fully effective. Additionally, cycle slips caused by single interruptions in the receiver's phase tracking may decrease the number of satellites used for positioning. It means that there may not be enough satellites to resolve the position. This can be avoided using a multi-GNSS solution as Marques et al. (2018) pointed out. As expected, for latitude zones 30° N–45° N and 45° N–60° N the number of cycle slips found for both storms were significantly lower. This coincides with the values of positioning errors and the distribution of \(SIDX\) and \(ROTI\) indices. However, it is seen that the \(GIX\) values for these zones were not necessarily consistent with the occurrence of cycle slips. This is clearly visible for the 30° N–45° N zone during the storm in June 2015, where the \(GIX\) and \({GIX}_{95}\) values were higher than for the 60° N–75° N zone, and the cycle slips number was lower. It is worth pointing out that the data gap in the phase observations caused 5–10% of total detected cycle slips. The others were found using methods implemented in gLAB. During both events, at least 90% of detected cycle slips had a value of at least two times greater than the adopted threshold. Therefore, we can assume a low number of falsely detected cycle slips and their insignificant impact on our analyses.
To estimate the relationship between the occurrence of cycle slips and the temporal variations of \(VTEC\), we plotted the number of cycle slips occurrences depending on the values of the \(GIX\), \(SIDX\), and \(ROTI\) indices (Fig. 7). We presented the results for the 60° N–75° N zone, and both analyzed magnetic storms. Since \(SIDX\) takes positive and negative values, we plot its absolute value. We fitted a straight line for each case and determined the Pearson correlation coefficient. The p-values did not exceed 1e-6. Therefore, we considered the obtained values to be statistically significant. The highest correlation value for both storms was obtained for the \(ROTI\) index, as much as 0.88 in both cases. Moreover, the parameters of the fitted line were very similar in both events: a = 0.949 and b = −0.149 for the storm in March 2015 and a = 0.996 and b = −0.136 for the storm in June 2015. We also performed a similar calculation for the zone 45° N–60° N. Also, in this case, we obtained high correlations, 0.79 and 0.73, for March and June, respectively. In this case, however, the line fit coefficients differed more: a = 0.547 and b = −0.041 for the storm in March 2015 and a = 0.317 and b = −0.011 for June 2015. For other indices, the correlations were different depending on the event. \(GIX\) had a very high correlation during the storm in June 2015 and was 0.79. However, in March, it was much lower −0.54. In addition, the straight-line fit parameters differed significantly and had a larger fit error (Fig. 7). Even lower correlations were obtained for the \(SIDX\) index, 0.61 and 0.45. For the 45° N–60° N zone, the results were even lower and amounted to 0.17 and 0.13 for the storm in June and March. In this case, \(GIX\) resulted in better scores with 0.44 and 0.66. However, these values are lower than those obtained for the \(ROTI\). The correlations for \({ROTI}_{95}\), \({GIX}_{95}\), and \({SIDX}_{95}\) are lower because these parameters represent values that only occur 5% of the time for a given zone.
Relative positioning
For relative positioning, we chose such baselines which were in the zones where there are large \(GIX\) values and \(VTEC\) time changes, represented by \(ROTI\) and \(SIDX\), are relatively minor. Thanks to this, we have limited the possibility of cycle slips and their impact on positioning results. Figures 4 and 5 show the results of relative positioning. The Iono-free shows the level of possible precision for long baselines. For the analyzed cases, whose length was between 400 and 600 km, we obtained an average error value not exceeding 0.1 m for horizontal components and 0.2 m for altitude. These results were affected by spikes observed in the afternoon and evening hours during the active phase of the storm. The occurrence of these jumps was related to the \(ROTI\) and \(SIDX\) peaks occurring at the same time (Fig. 1). These sudden and large \(VTEC\) changes caused the cycle slips effects, which decreased the precision of baseline estimation. However, this does not explain the degradation of positioning in L1 broadcast and L1 IONEX solutions. In both cases and for both analyzed storms, we see that the positioning degradation occurs at different times than the increase in ROTI and SIDX values. For the POTS-AUBG baseline, we see a jump in the solution after the altitude component is already around 12:00. At the same time, we see that the \(GIX\) for the zone where this baseline was located (45°N-60°N) was 13.7 mTECU/km, and the parameter \({GIX}_{95}\), 24.8 mTECU/km. Similar relationships can be found for the VILL-GAIA and VILL-MALA baselines during the storm in June 2015.
The \(GIX\) shows the average values of gradients for the entire zone and represents the periods when the positioning errors increased. However, the gradients of individual stations/baselines may differ. Therefore, to better look at the relationship between gradients and relative positioning degradation, we determined the gradients between the satellites observed by the stations forming a given baseline. The results for both analyzed cases are presented in Fig. 8. Each line presented is the gradient value between the \(VTEC\) values for satellites observed by two stations. The results show that the smallest amplitude of gradient values was for the POTS-DELF baseline. For one satellite, around 16:00, the maximum value was about −56 mTECU/km. Apart from this event, \(\nabla VTEC\) did not exceed 20 mTECU/km. This results in the highest precision of this baseline for L1 broadcast and \(L1 IONEX\) solutions.
Vertical total electron content gradients (\(\nabla VTEC)\) between satellites observed from receivers within the baselines. On top: results for baselines POTS-DELF and POTS-AUBG during the geomagnetic storm in March 2015. On bottom: results for baselines VILL–GAIA and VILL–MALA during the geomagnetic storm in June 2015. Each line represents the gradients for one satellite
The second baseline analyzed during the storm in March 2015 is POTS-AUBG. It was directed parallel to the direction of the gradients (Fig. 8). The maximum gradient was as much as −85 mTECU/km. If we look at Fig. 4 (right), it is seen that an apparent decrease in the precision of determining the baseline lasted from about 06:00 to 36:00. During that time, the gradient values exceeded 30 mTECU/km, with a break between 24:00 and 30:00, which is also visible in the positioning results. It is worth noting here that for both analyzed baselines, the maximum values of \(\nabla VTEC\) occurred at the same time as the maximum values of \(ROTI\), \(SIDX\), and jumps in Iono-free solutions caused by cycle slips.
A similar situation occurred for \(\nabla VTEC\) analyzed for the June 2015 storm. However, gradient values were much higher with lower \(ROTI\) and \(SIDX\) values. This means that most of the \(\nabla VTEC\) were dominated by spatial changes in \(VTEC\), not by their temporal changes. For both the VILL-GAIA and VILL-MALA baselines, we observe that the time of occurrence of large positioning errors coincides with the time of occurrence of high values of \(\nabla VTEC\). The maximum \(\nabla VTEC\) was about 80 mTECU/km in the first case. In the second, over 100 mTECU/km. It is also worth noting how quickly the values of the gradients changed in the case of the VILL-MALA. The values changed from −100 to 100 mTECU/km in less than an hour. These changes are also very well reflected in the positioning results for both single-frequency solutions (Fig. 5, right).
To check why these gradients significantly impacted the positioning results, we determined them from the Klobuchar model and the IONEX used in the positioning. We did it the same way as before. The results shown in Fig. 9 show how \(\nabla VTEC\) was reflected in modeling the ionospheric delay with a single-frequency solution. It is clear that the gradients have been definitely underestimated. In the case of the Klobuchar model, which is an empirical model, it can be said that we do not see any significant gradients for the analyzed baselines. The values do not exceed 10 mTECU/km. The course of \(\nabla VTEC\) determined from IGS ionospheric maps for the POTS-DELF and POTS-AUBG baseline had a course like the real one. However, also in this case, the values were significantly underestimated. At the time of maximum gradients, the difference between the actual values and those determined from the IONEX file was about 50 mTECU/km. Larger differences were observed for the VILL-GAIA and VILL-MALA baselines in June 2015. While \(\nabla VTEC\) exceeded 100 mTECU/km at that time, the values from IONEX were not greater than 25 mTECU/km. This means that the difference in the uncorrected ionospheric delay between the two satellites about 400 km apart was 30 TECU (about 4.8 m for L1 frequency). The significant pseudorange error for many satellites translated into large relative positioning errors. The IONEX data poorly represented \(\nabla VTEC\) values because of the small number of stations used to reflect local changes in the ionosphere, as well as the low temporal (2 h) and spatial resolution (5° longitude by 2.5° latitude). Also, global ionospheric maps are generated by fitting the spherical harmonics basis function to the GNSS data, which smooths out local gradients.
Vertical total electron content gradients (\(\nabla VTEC)\) between satellites observed from receivers within the baselines estimated from Klobuchar model (red lines) and global ionospheric maps stored in IONEX files (green lines). On top: results for baselines POTS-DELF and POTS-AUBG during the geomagnetic storm in March 2015. On bottom: results for baselines VILL–GAIA and VILL–MALA during the geomagnetic storm in June 2015. Each line represents the gradients for one satellite
Summary
We presented results of precise positioning and their relationship with the ionospheric indices \(GIX\),\(SIDX\), and \(ROTI\). Our analyses were performed for two selected geomagnetic storms in 2015. Our findings and conclusions are summarized in the following points:
-
In the PPP method, large positioning errors were caused by multiple cycle slip effects due to the strong temporal and small-scale \(VTEC\) changes. These changes were described by the \(ROTI\), \(SIDX\), and partly \(GIX\) indices. The increase in positioning errors was found to be related to the above-mentioned changes in indices and magnitude.
-
The average value of the number of cycle slips in given sectors was strongly correlated with the \(ROTI\) index and amounted to 0.88 for the 60°N-75°N zone for both analyzed geomagnetic storms.
-
In relative positioning, accuracy spikes can be caused by temporal \(VTEC\) changes that cause cycle slips to occur at one or both stations in the baseline, increasing positioning errors. This applies to both single-frequency and dual-frequency measurements.
-
In single-frequency relative positioning, greater errors in baseline estimation occurred for baselines parallel to the direction of the gradients. This is because neither the Klobuchar model nor the data from global ionospheric maps can model the size of these gradients properly.
-
The occurrence of large positioning errors is related to changes and size of the \(GIX\) index. However, it is difficult to find an unambiguous relationship between the magnitude of \(GIX\) and the value of the positioning error because it depends on the method of mitigating the ionospheric delay and the length of the vector.
-
Using the \(GIX\) and \(ROTI\) indices together can help assess the positioning quality by both absolute and relative methods at one or more frequencies.
-
The conducted research also shows that there is a need to develop ionospheric delay models, which will reduce the impact of ionospheric gradients to a greater extent. This is extremely important, especially in applications where long-baseline positioning is used.
Data availability
Data available upon reasonable request.
References
Arslan N, Demirel H (2008) The impact of temporal ionospheric gradients in Northern Europe on relative GPS positioning. J Atmos Solar Terr Phys 70(2008):1382–1400. https://doi.org/10.1016/j.jastp.2008.03.010
Berdermann J, Kriegel M, Banyś D, Heymann F, Hoque MM, Wilken V, Borries C, Heßelbarth A, Jakowski N (2018) Ionospheric response to the X9.3 Flare on 6 September 2017 and its implication for navigation services over Europe. Space Weather 16:1604–1615. https://doi.org/10.1029/2018SW001933
Blewitt G (1990) An automatic editing Algorithms for GPS data. Geophys Res Lett 17(3):199–202
Borries C, Wilken V, Jacobsen KS, Garcia-Rigo A, Dzik-Jakowska B, Kervalishvili G, Jakowski N, Tsagouri I, Hernandez-Pajares M, Ferreira AA, Hoque MM (2020) Assessment of the capabilities and applicability of ionospheric perturbation indices provided in Europe. Adv Space Res 66:546–562. https://doi.org/10.1016/j.asr.2020.04.013
Carter BA et al (2023) The ionospheric effects of the 2022 Hunga Tonga Volcano eruption and the associated impacts on GPS precise point positioning across the Australian Region. Space Weather 21:5. https://doi.org/10.1029/2023SW003476
Clausen LBN, Moen JI, Hosokawa K, Holmes JM (2016) GPS scintillations in the high latitudes during periods of dayside and nightside reconnection. J Geophys Res Space Phys 121:3293–3309. https://doi.org/10.1002/2015JA022199
Forte B, Radicella SM (2002) Problems in data treatment for ionospheric scintillation measurements. Radio Sci 37:8-1-8–5. https://doi.org/10.1029/2001RS002508
Guo K, Aquino M, Veettil SV, Liu Z, Chen W, Marques HA (2019) Analysis of ionospheric scintillation and its impact on PPP at low latitudes. Proceedings of the Institute of Navigation Pacific Positioning, Navigation and Timing Meeting, Pacific PNT, 2019-Apr, pp. 835–845. https://doi.org/10.33012/2019.16842
Hernández-Pajares M, Juan JM, Sanz J, Ramos-Bosch P, Rovira-Garcia A, Salazar D, Ventura-Traveset J, Lopez C, Hein G (2010) The ESA/UPC GNSS-Lab tool (gLAB): an advanced multipurpose package to process and analyse GNSS data. In: 5th ESA Workshop on Satellite Navigation User. Noordwijk: European Space Agency (ESA), pp 1–8
Hlubek N, Berdermann J, Wilken V, Gewies S, Jakowski N, Wassaie M, Damtie B (2014) Scintillations of the GPS, GLONASS, and Galileo signals at equatorial latitude. J Space Weather Space Clim 4:A22. https://doi.org/10.1051/swsc/2014020
Jacobsen KS, Andalsvik YL (2016) Overview of the 2015 St. Patrick’s day storm and its consequences for RTK and PPP positioning in Norway. J Space Weather Space Clim 6:A9. https://doi.org/10.1051/swsc/2016004
Jakowski N, Borries C, Wilken V (2012) Introducing a disturbance ionosphere index (DIX). Radio Sci 47:RS0L14. https://doi.org/10.1029/2011RS004939
Jakowski N, Hoque MM (2019) Estimation of spatial gradients and temporal variations of the total electron content using ground-based GNSS measurements. Space Weather. https://doi.org/10.1029/2018SW002119
Juan JM, Sanz J, Rovira-Garcia A, González-Casado G, Ibáñez D, Perez RO (2018) AATR an ionospheric activity indicator specifically based on GNSS measurements. J Space Weather Space Clim 8:A14. https://doi.org/10.1051/swsc/2017044
Kintner PM, Ledvina BM, de Paula ER (2007) GPS and ionospheric scintillations. Space Weather. https://doi.org/10.1029/2006SW000260
Li W, Song S, Zhou W, Cheng N, Yu C (2022) Investigating the impacts of ionospheric irregularities on precise point positioning over china and its mechanism. Space Weather. https://doi.org/10.1029/2022SW003236
Lu Y, Wang Z, Ji S, Chen W (2020) Assessing the positioning performance under the effects of strong ionospheric anomalies with multi-GNSS in Hong Kong. Radio Sci 55:8. https://doi.org/10.1029/2019RS007004
Luo X, Gu S, Lou Y, Xiong C, Chen B, Jin X (2018a) Assessing the performance of GPS precise point positioning under different geomagnetic storm conditions during solar cycle 24. Sensors 18(6):1784. https://doi.org/10.3390/s18061784
Luo X, Lou Y, Xiao Q, Gu S, Chen B, Liu Z (2018b) Investigation of ionospheric scintillation effects on BDS precise point positioning at low-latitude regions. GPS Solut 22(3):63. https://doi.org/10.1007/s10291-018-0728-8
Luo X, Gu S, Lou Y, Chen B, Song W (2020) Better thresholds and weights to improve GNSS PPP under ionospheric scintillation activity at low latitudes. GPS Solut 24:17. https://doi.org/10.1007/s10291-019-0924-1
Ma G, Maruyama T (2003) Derivation of TEC and estimation of instrumental biases from GEONET in Japan. Ann Geophys 21(10):2083–2093. https://doi.org/10.5194/angeo-21-2083-2003
Marques HA, Marques HAS, Aquino M, Veettil SV, Monico J (2018) Accuracy assessment of precise point positioning with multi-constellation GNSS data under ionospheric scintillation effects. J Space Weather Space Clim 8:A15. https://doi.org/10.1051/swsc/2017043
Mayer C, Belabbas B, Jakowski N, Meurer M, Dunkel W (2009) Ionosphere Threat Space Model Assessment for GBAS, In: Proc. ION GNSS 2009, pp 22–25. Sep 2009, Savannah, GA, USA
Moreno B, Radicella S, de Lacy MC, Herraiz M, Rodriguez-Caderot G (2011) On the effects of the ionospheric disturbances on precise point positioning at equatorial latitudes. GPS Solut 15:381–390. https://doi.org/10.1007/s10291-010-0197-1
Nie W, Rovira-Garcia A, Li M, Fang Z, Wang Y, Zheng D, Xu T (2022) The mechanism for GNSS-based kinematic positioning degradation at high-latitudes under the march 2015 great storm. Space Weather 20:6. https://doi.org/10.1029/2022SW003132
Olwendo JO, Cilliers P, Weimin Z, Ming O, Yu X (2018) Validation of ROTI for ionospheric amplitude scintillation measurements in a low-latitude region over Africa. Radio Sci 53(7):876–887. https://doi.org/10.1029/2017RS006391
Pi X, Mannucci AJ, Lindqwister UJ, Ho CM (1997) Monitoring of global ionospheric irregularities using the Worldwide GPS Network. Geophys Res Lett 24:2283–2286. https://doi.org/10.1029/97GL02273
Poniatowski M, Nykiel G (2020) Degradation of kinematic PPP of GNSS stations in central europe caused by medium-scale traveling ionospheric disturbances during the St. Patrick’s day 2015 geomagnetic storm. Remote Sens 12:3582. https://doi.org/10.3390/rs12213582
Rodríguez-Bilbao I, Radicella SM, Rodríguez-Caderot G, Herraiz M (2015) Precise point positioning performance in the presence of the 28 October 2003 sudden increase in total electron content. Space Weather 13(10):698–708. https://doi.org/10.1002/2015SW001201
Takasu T, Yasuda A (2009) Development of the low-cost RTK-GPS receiver with an open source program package RTKLIB. In: International Symposium on GPS/GNSS, International Convention Center Jeju, Korea, Nov 4–6
Wielgosz P, Kashani I, Grejner-Brzezinska D (2005) Analysis of long-range network RTK during a severe ionospheric storm. J Geod 79:524–531. https://doi.org/10.1007/s00190-005-0003-y
van der Meeren C, Oksavik K, Lorentzen DA, Rietveld MT, Clausen LBN (2015) Severe and localized GNSS scintillation at the poleward edge of the nightside auroral oval during intense substorm aurora. J Geophys Res: Space Phys 120:10607–10621. https://doi.org/10.1002/2015JA021819
Valdés-Abreu JC, Díaz MA, Báez JC, Stable-Sánchez Y (2022) Effects of the 12 May 2021 geomagnetic storm on georeferencing precision. Remote Sens 14(1):38. https://doi.org/10.3390/rs14010038
Yang Z, Liu Z (2016) Correlation between ROTI and Ionospheric Scintillation Indices using Hong Kong low-latitude GPS data. GPS Solut 20(4):815–824. https://doi.org/10.1007/S10291-015-0492-Y/FIGURES/9
Yu S, Liu Z (2021) The ionospheric condition and GPS positioning performance during the 2013 tropical cyclone Usagi event in the Hong Kong region. Earth, Planets Space 73(1):66. https://doi.org/10.1186/s40623-021-01388-2
Acknowledgements
The authors would like to thank the EUREF Permanent GNSS Network for providing observational data from GNSS reference stations. We acknowledge support for Grzegorz Nykiel from the Gdańsk University of Technology by the DEC-31/2021/IDUB/I.3.3 grant under the ARGENTUM—‘Excellence Initiative—Research University’ program.
Author information
Authors and Affiliations
Contributions
GN: Conceptualization, Methodology, Formal analysis, Investigation, Visualization, Writing—Original Draft, Writing—Review & Editing; JAC: Conceptualization, Validation, Writing—Review & Editing; MH: Conceptualization, Validation, Writing—Review & Editing; NJ: Conceptualization, Validation, Writing—Review & Editing.
Corresponding author
Ethics declarations
Conflict of interest
The authors declare that they have no known competing financial interests or personal relationships that could have appeared to influence the work reported in this paper.
Additional information
Publisher's Note
Springer Nature remains neutral with regard to jurisdictional claims in published maps and institutional affiliations.
Rights and permissions
Open Access This article is licensed under a Creative Commons Attribution 4.0 International License, which permits use, sharing, adaptation, distribution and reproduction in any medium or format, as long as you give appropriate credit to the original author(s) and the source, provide a link to the Creative Commons licence, and indicate if changes were made. The images or other third party material in this article are included in the article's Creative Commons licence, unless indicated otherwise in a credit line to the material. If material is not included in the article's Creative Commons licence and your intended use is not permitted by statutory regulation or exceeds the permitted use, you will need to obtain permission directly from the copyright holder. To view a copy of this licence, visit http://creativecommons.org/licenses/by/4.0/.
About this article
Cite this article
Nykiel, G., Cahuasquí, J.A., Hoque, M.M. et al. Relationship between GIX, SIDX, and ROTI ionospheric indices and GNSS precise positioning results under geomagnetic storms. GPS Solut 28, 69 (2024). https://doi.org/10.1007/s10291-023-01611-5
Received:
Accepted:
Published:
DOI: https://doi.org/10.1007/s10291-023-01611-5