Abstract
Medical image segmentation is one of the key tasks in the medical field and is crucial for accurate lesion detection and treatment planning. However, the small sample problem has been one of the challenges in medical image segmentation. In this study, the small sample medical image segmentation problem is evaluated experimentally based on two different datasets, the VOC dataset and the esophageal medical images. In the VOC dataset experiments, we used the code from the BAM project on GitHub as a benchmark and compared it. Additionally, we improved the benchmark code by adding the Attention Mechanism (CBAM), placing its position before the support set undergoes global average pooling. By comparing the experimental results, we found that the model with the added CBAM achieved better segmentation results under small sample conditions. In the esophageal medical image experiments, the code from the BAM project was also used as a benchmark and a similar experimental design was performed. We further added CBAM by placing it before the global average pooling of the support set. The experimental results show that the model with the addition of CBAM achieves a significant improvement in the small sample segmentation task of esophageal medical images. To summarize, this study validates the effectiveness of using the BAM model in the small-sample medical image segmentation task on two different datasets. By adding CBAM, our model exhibits better segmentation performance under small sample conditions. These findings provide useful insights for small-sample medical image segmentation and are important for future research and practice.
Export citation and abstract BibTeX RIS
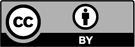
Content from this work may be used under the terms of the Creative Commons Attribution 3.0 licence. Any further distribution of this work must maintain attribution to the author(s) and the title of the work, journal citation and DOI.