Abstract
The detection of all sorts of birds has become increasingly important in the fields of ecological balance and biological protection. To tackle the problems of low accuracy, high omission rate and low detection confidence levels in the application of artificial intelligence and deep learning in bird detection, this paper proposes a bird detection method that leverages the YOLOv5s model and incorporates the asymptotic feature pyramid network module (AFPN). Diverging from the conventional pyramid network module (FPN), AFPN offers a more efficient solution, characterized by reduced computation time and memory consumption. It also minimizes conflicts that may arise during feature training. To further enhance the model's performance and efficiency, the project introduces an object detection regression loss function along with several distinct loss functions. These functions are employed to train and analyze a standardized dataset, enabling the identification of an optimal solution. Through rigorous model construction, training, and repeated testing, notable improvements have been achieved in the accuracy rate and other relevant indicators, meeting the necessary application standards. This refined method exhibits great potential for fulfilling diverse bird detection requirements.
Export citation and abstract BibTeX RIS
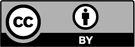
Content from this work may be used under the terms of the Creative Commons Attribution 3.0 licence. Any further distribution of this work must maintain attribution to the author(s) and the title of the work, journal citation and DOI.