Abstract
The crucial task of polyp recognition in medical imaging plays a pivotal role in the early detection and prevention of colorectal cancer. Semantic segmentation, particularly utilizing sophisticated deep learning models such as U-Net, has demonstrated promising results in the realm of polyp segmentation. However, the traditional U-Net structure sometimes grapples with accurately delineating the edges of polyps, which subsequently impacts the overall performance of segmentation. To address this issue, the current study introduces a novel approach by proposing a modified framework of U-Net, equipped with an enhanced edge loss function. This function is designed to ameliorate the accuracy of segmentation within polyp images. The aim is to elevate the model's capacity to capture intricate details, specifically the edges, which is an area where standard U-Net structures often falter. Experimental outcomes of this study serve to underscore the effectiveness of the proposed approach in accomplishing superior segmentation of edges and improved overall performance in polyp recognition. By successfully tackling the challenges inherent to polyp edge segmentation, the modified U-Net model contributes significantly towards more precise diagnostic systems in the field of medical imaging. Consequently, this research is poised to make a valuable contribution to advancements in the prevention and early detection of colorectal cancer.
Export citation and abstract BibTeX RIS
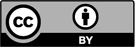
Content from this work may be used under the terms of the Creative Commons Attribution 3.0 licence. Any further distribution of this work must maintain attribution to the author(s) and the title of the work, journal citation and DOI.