Abstract
Dissolved oxygen is one of the important comprehensive indicators of river water quality, which reflects the degree of pollution in the water body. Monitoring and predicting dissolved oxygen are an important tool for water quality management, which helps to effectively maintain water ecological balance and prevent environmental problems. A single model cannot describe the dynamic characteristics of dissolved oxygen sequence, which affects the prediction accuracy. In order to obtain more accurate dissolved oxygen prediction results, decomposition techniques are commonly used to extract the main fluctuations and trends of water quality sequences. However, the high-frequency modes obtained from decomposition are still unstable. To solve this problem, this paper proposed a hybrid prediction model of dissolved oxygen concentration based on secondary decomposition and bidirectional gate recurrent unit. Firstly, dissolved oxygen sequence is preliminarily decomposed by complete ensemble empirical mode decomposition with adaptive noise (CEEMDAN) and obtain several intrinsic mode functions (IMF). The fuzzy entropy (FE) is calculated to quantify the complexity of the IMF. Then, variational mode decomposition improved by northern goshawk optimization is used to decompose the IMF with higher entropy. The nonlinearity and instability of the sequence are further weakened. Finally, the bidirectional gate recurrent unit (BiGRU) neural network is used to predict each IMF component, and the final prediction result is obtained by reconstructing the prediction results of each component. In order to verify the effectiveness of the proposed model, this paper selects the dissolved oxygen data of Xin'anjiang Reservoir as the research object. The experimental results show that the RMSE, MAE, MAPE, and R2 of the proposed model are 0.1164, 0.0894, 1.0403%, and 0.9939, respectively, which is best among other comparative prediction models (BP, LSTM, GRU, BiGRU, EMD-BiGRU, CEEMDAN-BiGRU, VMD-BiGRU, and GNO-VMD-BiGRU). Therefore, this model effectively deals with high volatility and nonlinear dissolved oxygen data and provides reference for water environment management and ecological protection.











Similar content being viewed by others
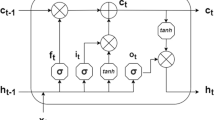
Data availability
The data used in this paper were obtained from China Environmental Monitoring Station. URL: http://www.cnemc.cn/
References
Akomeah, E., Chun, K. P., & Lindenschmidt, K. E. (2015). Dynamic water quality modelling and uncertainty analysis of phytoplankton and nutrient cycles for the upper south Saskatchewan river. Environmental Science and Pollution Research, 22(22), 18239–18251. https://doi.org/10.1007/s11356-015-4970-0
Alizadeh, M. J., & Kavianpour, M. R. (2015).Development of wavelet-ANN models to predict water quality parameters in Hilo Bay, Pacific Ocean Marine Pollution Bulletin, 98(1–2), 171–178. https://doi.org/10.1016/j.marpolbul.2015.06.052
Arora, S., & Keshari, A. K. (2023). Implementing machine learning algorithm to model reaeration coefficient of urbanized rivers. Environmental Modeling & Assessment, 28(4), 535–546. https://doi.org/10.1007/s10666-023-09895-0
Arora, S., & Keshari, A. K. (2022). Modelling re-aeration of rivers using predictive models and developed ANN models under varying hydrodynamic conditions. Hydrological Sciences Journal, 67(15), 2294–2309. https://doi.org/10.1080/02626667.2022.2138402
Bai, Y., & Li, Y. (2020). Prediction method of river water quality based on VMD-LSSVR. Journal of Safety and Environment., 20(03), 1162–1168. https://doi.org/10.13637/j.issn.1009-6094.2019.0727
Cao, X. K., Liu, Y. R., Wang, J. P., et al. (2020). Prediction of dissolved oxygen in pond culture water based on K-means clustering and gated recurrent unit neural network. Aquacultural Engineering, 91, 102122. https://doi.org/10.1016/j.aquaeng.2020.102122
Chen, W., Zhuang, J., Yu, W., & Wang, Z. Z. (2009). Measuring complexity using fuzzyen, apen, and sampen. Medical Engineering and Physics, 31(1), 61–68. https://doi.org/10.1016/j.medengphy.2008.04.005
Chung, J., Gulcehre, C., Cho, K., et al. (2014). Empirical evaluation of gated recurrent neural networks on sequence modeling. Neural and Evolutionary Computing., 1412, 3555.
Cox, B. A. (2003). A review of currently available in-stream water-quality models and their applicability for simulating dissolved oxygen in lowland rivers. Science of the Total Environment, 314, 335–377. https://doi.org/10.1016/S0048-9697(03)00063-9
Dehghani, M., Hubalovsky, S., & Trojovsky, P. (2021). Northern goshawk optimization: A new swarm-based algorithm for solving optimization problems. Ieee Access, 9, 162059–162080.
Dragomiretskiy, K., & Zosso, D. (2014). Variational mode decomposition. IEEE Trans on Signal Process, 62(3), 531–544.
Gao, R. W. (2018). Analysis of the current situation and countermeasures of water resource pollution in china. Resource Pollution and Proposes, 11, 44–51.
Hu, P., Yang, Q., Yang, Z. F., et al. (2019). Experimental study on dissolved oxygen content and its physical influencing factors in water. Journal of Hydraulic Engineering, 50(6), 679–686. https://doi.org/10.13243/j.cnki.slxb.20190108
Hu, Y. C., Li, Q. G., Wu, W., et al. (2023). Simulation and optimization of multi-source water quality coordination in the Jiaodong water diversion canal. China Rural Water Resources and Hydropower, 03, 86–93.
Huan, J., Li, H., Li, M. B., & Chen, B. (2020). Prediction of dissolved oxygen in aquaculture based on gradient boosting decision tree and long short-term memory network: A study of Chang Zhou fishery demonstration base, China. Computers and Electronics in Agriculture, 175, 105530. https://doi.org/10.1016/j.compag.2020.105530
Ji, X. L., Shang, X., Dahlgren, R. A., & Zhang, M. H. (2017). Prediction of dissolved oxygen concentration in hypoxic river systems using support vector machine: A case study of Wen-Rui Tang River. China. Environmental Science and Pollution Research, 24(19), 16062–16076.
Juan, H., Li, M. B., Xu, X. G., Hao, Z., Yang, B. E., Jiang, J. M., & Bing, S. (2022). Multi-step prediction of dissolved oxygen in river based on random forest missing value imputation and attention mechanism coupled with recurrent neural network. Water Supply, 22(5), 5480–5493. https://doi.org/10.2166/ws.2022.154
Kai, G., Sun, S., Wang, S., et al. (2018). A secondary-decomposition-ensemble learning paradigm for forecasting PM2.5 concentration. Atmospheric Pollution Research, 9(6), 989–999. https://doi.org/10.1016/j.apr.2018.03.008
Kang, J. L., Wang, H. M., Yuan, F. F., Wang, Z. Q., Huang, J., & Qiu, T. (2020). Prediction of precipitation based on recurrent neural networks in Jingdezhen, Jiangxi province China. Atmosphere, 11(3), 246. https://doi.org/10.3390/atmos11030246
Lai, X. M., Tang, H., Chen, H. Y., et al. (2021). Multi-modal emotion analysis of feature fusion–bidirectional gated loop unit based on attention mechanism. Computer Application., 41(05), 1268–1274.
Li, Y. Z., Liu, Q. L., Deng, M., et al. (2023). River water quality prediction model based on physically constrained GRU neural network. Journal of Geoinformation Science, 25(01), 102–114.
Liu, C. L., Wu, Y. J., & Zhen, C. G. (2015). Rolling bearing fault diagnosis based on variational mode decomposition and fuzzy C-means clustering. Chinese Journal of Electrical Engineering, 35(13), 358–3365.
Liu, S. Y., Xu, L. Q., & Li, D. L. (2016). Multi-scale prediction of water temperature using empirical mode decomposition with back-propagation neural networks. Computers and Electrical Engineering, 49, 1–8. https://doi.org/10.1016/j.compeleceng.2015.10.003
Nair, J. P., & Vijaya, M. S. (2022). Analysing and modelling dissolved oxygen concentration using deep learning architectures. International Journal of Mechanical Engineering, 7, 12–22. https://doi.org/10.56452/2022-11-002
Nas, S. S., Bayram, A., & Bulut, V. N. (2008). Effects of some water quality parameters on the dissolved oxygen balance of streams. Polish Journal of Environmental Studies, 17(4), 531–538.
Nemati, S., Fazelifard, M. H., et al. (2015). Estimation of dissolved oxygen using data-driven techniques in the Tai Po River Hongkong. Environmental Earth Sciences., 74(5), 4065–4073. https://doi.org/10.1007/s12665-015-4450-3
Ren, Y. Q., Jin, Z. C., Yu, Z. Y., et al. (2022). Prediction of ammonia nitrogen in surface water based on bidirectional gated circulation unit. Chinese Environmental Science, 42, 672–679. https://doi.org/10.19674/j.cnki.issn1000-6923.2022.0020
Ro, Y. J., & Choi, Y. H. (2004). Application of realtime monitoring of oceanic conditions in the coastal water for environmental management. Ocean Science Journal, 39(2), 148–154.
Song, C. G., Yao, L. H., Hua, C. Y., & Ni, Q. H. (2022). A water quality prediction model based on variational mode decomposition and the least squares support vector machine optimized by the sparrow search algorithm (VMD-SSA-LSSVM) of the Yangtze River. China. Environmental Monitoring and Assessment, 193(6), 363. https://doi.org/10.1007/s10661-021-09127-6
Tao, Z. Y., Hu, Q. Z., & Ren, X. K. (2022). Prediction of river dissolved oxygen based on two-layer decomposition technique and improved neural network. Journal of Yunnan University, 44(02), 262–270.
Torres, M. E., Colominnas, M. A., Schlottauerg, G., et al (2011). A complete ensemble empirical modedecomposition with adaptive noise. IEEE International conference on acoustics. 4144–4147
Tu, J. C., Chen, C. B., Wang, J. C., et al. (2019). Research on water quality prediction model based on deep learning. Automation and Instrumentation., 34(06), 96–100. https://doi.org/10.19557/j.cnki.1001-9944.2019.06.021
Wang, Y. W., Du, Z. H., Dai, Z., et al. (2022). Multivariate water quality index prediction model based on composite neural network. Journal of Zhejiang University Science Editio., 49(03), 354–362, 375.
Wang, W. C., Du, Y. J., Chau, K. W., et al. (2021). An ensemble hybrid forecasting model for annual runoff based on sample entropy, secondary decomposition, and long short-term memory neural network. Water Resources Management, 35(14), 4695–4726. https://doi.org/10.1007/s11269-021-02920-5
Wang, Y. T., Liu, J., Li, R., Suo, X. Y., & Lu, E. H. (2022). Medium and Long-term precipitation prediction using wavelet decomposition-prediction-reconstruction model. Water Resources Management, 36(3), 971–987. https://doi.org/10.1007/s11269-022-03063-x
Wu, J., Li, Z., Zhu, L., Li, G. Y., Niu, B. S., & Peng, F. (2018). Optimized BP neural network for dissolved oxygen prediction. IFAC PAPERSONLINE, 51(17), 596–601.
Yu, C. Z., Li, Y., & Bai, Y. (2018). Dissolved oxygen prediction based on ensemble empirical mode decomposition and support vector machine. Environmental Monitoring Management and Technology, 30(03), 27–31. https://doi.org/10.19501/j.cnki.1006-2009.20180513.014
Zhang, Z., Li, C., Zeng, X. L., et al. (2006). Application of QUAL2E model in water quality simulation of the Chongqing section of the Yangtze River. Environmental Science and Technology., 01, 1–3+115.
Zhao, X. H., Fang, Z. D., He, Y., & Cha, Y. H. (2021). Anomaly detection method of dam automatic monitoring data based on data reconstruction and isolated forest method. National Rural Water Conservancy and Hydropower., 09, 174–178.
Funding
This study is founded by Natural Science Foundation of Zhejiang Province (LQ20E090006), the Joint Funds of the Zhejiang Provincial Natural Science Foundation of China under Grant No (LZJWY24E090001) and the National Key Research and Development Program of China (No. 2023YFC3207500).
Author information
Authors and Affiliations
Contributions
All authors contributed to the study conception and design. Methods, data collection and analysis were performed by QM, JJ, LZ, and FL. The first draft of the manuscript was written by QM. SH and JJ were mainly responsible for proofreading the manuscript. All authors read and approved the final manuscript.
Corresponding author
Ethics declarations
Competing interests
The authors declare no competing interests.
Ethics approval
The research was conducted and the manuscript was prepared following the ethical guidelines advised by the environmental science and pollution research journal.
Consent to participate
Not applicable.
Consent for publication
Not applicable.
Additional information
Publisher's Note
Springer Nature remains neutral with regard to jurisdictional claims in published maps and institutional affiliations.
Rights and permissions
Springer Nature or its licensor (e.g. a society or other partner) holds exclusive rights to this article under a publishing agreement with the author(s) or other rightsholder(s); author self-archiving of the accepted manuscript version of this article is solely governed by the terms of such publishing agreement and applicable law.
About this article
Cite this article
Jiao, J., Ma, Q., Liu, F. et al. A hybrid prediction model of dissolved oxygen concentration based on secondary decomposition and bidirectional gate recurrent unit. Environ Geochem Health 46, 127 (2024). https://doi.org/10.1007/s10653-024-01884-w
Received:
Accepted:
Published:
DOI: https://doi.org/10.1007/s10653-024-01884-w