Abstract
This paper examines the implications of AI and machine translation on traditional lexicography, using three canonical scenarios for dictionary use: text reception, text production, and text translation as test cases. With the advent of high-capacity, AI-driven language models such as OpenAI’s GPT-3 and GPT-4, and the efficacy of machine translation, the utility of conventional dictionaries comes under question. Despite these advancements, the study finds that lexicography remains relevant, especially for less-documented languages where AI falls short, but human lexicographers excel in data-sparse environments. It argues for the importance of lexicography in promoting linguistic diversity and maintaining the integrity of lesser-known languages. Moreover, as AI technologies progress, they present opportunities for lexicographers to expand their methodology and embrace interdisciplinarity. The role of lexicographers is likely to shift towards guiding and refining increasingly automated tools, ensuring ethical linguistic data use, and counteracting AI biases.
Similar content being viewed by others
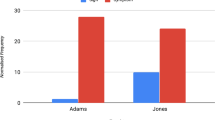
Introduction and background
The origins of lexicography
Before I assess the future path of lexicography, it is relevant to reflect on the original motivation that drove people to want, make, and finally use dictionaries. Rather uncontroversially, lexicography and dictionaries came about from communication-related challenges. The relatively slow speed with which dictionary consultation occurs, at least in its traditional print format which, historically speaking, had prevailed until quite recently, means that dictionary consultation had been viable in the context of reading or writing, rather than spontaneous conversation. The complexity and engagement level of the process of dictionary consultation does not allow for this activity to be usefully practised during conversation (it can be done while listening to pre-recorded material which can be paused, though). In broad outline, most situations of dictionary use in communication-related problems can be reduced to three general, canonical types: (1) text comprehension; (2) text production; and (3) text translation.
Text comprehension is about the understanding of text, usually written, except when the speech or conversation can be paused (such as in recorded speech) for the duration of dictionary consultation (Scholfield, 1999). Obviously, communication problems often arise in cross-linguistic contexts; that is, while reading a text written in a language that is not the reader’s first/strongest language. Historically, this was, for example, the case with written documents in Latin, and so quite a few bilingual dictionaries with Latin and another language were produced to help with the comprehension of texts written in Latin, such as those in the religious or legal domain. However, comprehension problems may also arise intra-linguistically. Texts written in what is nominally the same language as the reader’s first language may be difficult to understand because the variety of the language in which the text is written may be unfamiliar to the reader. This may, for example, be due to an unfamiliar register, regional dialect, specialized domain, or else the time period in which the text was originally written. There may be occasional unfamiliar lexical items or usages that the reader may wish to look up. A technical text from a domain in which the reader does not have domain knowledge and specialized language competence is an interesting case that I will come back to later.
Text production concerns writing rather than speaking, as speaking is too time-sensitive to be usefully supported by a dictionary (if we ignore rather marginal and special use cases such as preparing speeches to be read out loud at a later time). Other things being equal, understanding a text that is already out there is easier than producing one from scratch. To understand a text, we just have to decode semantically an existing text with its lexis and structures. This is inherently less cognitively challenging than having to come up with all the words and structures to construct a complete well-formed text, even in a language that we are proficient in. For example, it is well established that one’s receptive vocabulary is as a rule a superset of one’s productive vocabulary (Webb, 2008). All in all, there is greater potential for dictionary use while writing than while reading, even if it is not always obvious how to use a dictionary effectively while writing (Rundell, 1999). Amongst the challenges of text production is finding the lexical items appropriate for the expression of the intended meaning. These lexical items may well be known to the speaker, but recalling (retrieving) them may be difficult. Then there is the issue of using conventional constructions for the given lexical item, as well as natural collocations (that is, conventional co-occurrences of words). The last point is particularly tricky for non-native writers, even advanced ones, but, as it turns out, also for native writers when faced with a specific text genre such as academic English (Hyland and Shaw, 2016, Frankenberg-Garcia, 2018). Until a few decades ago, spelling may have been a problem, but spellcheckers built into text editors and word processors had gradually and largely taken over the task.
Text translation involves transforming a text from one language to another. This is a complex task that can be said to include reception and production phases (see above). Other things being equal, the more challenging phase is — on the one hand — the one involving the language in which one is less proficient, and — on the other hand — production as opposed to reception. The more ‘natural’ dictionaries to use in translation are bilingual rather than monolingual dictionaries (Tarp, 2004, Augustyn, 2013, Adamska-Sałaciak, 2015); however, monolingual dictionaries, including specialized collocation dictionaries, may be more appropriate in post-editing.
The classification of tasks calling for dictionary use into the above three categories is obviously a simplification (for an attempt at a finer taxonomy of dictionary use situations, see e.g. Tarp, 2008) but it is, with some confidence, a broadly correct sketch of the fundamental situations and tasks in which dictionaries have historically been used. The interesting question to be addressed today is to what extent this is still the case at present. Do we still need to use dictionaries in these situations, and are they the best choice in terms of efficiency and accuracy? Before this question is tackled, let me take a closer critical look at the form of dictionaries.
The form of dictionaries serves access needs
Until quite recently, lexicography had been a rather conservative type of activity, working with a set of conventions that had prevailed over centuries. These conventions formed a canon for the dictionary makers, who looked to the earlier creations for inspiration, and for the dictionary users, who expected a certain familiar form and format. One of the best (if not the best) elucidations of the circumstances that had conspired to produce the canonical dictionary format and structure is that by Dwight Bolinger (1985):
Lexicography is an unnatural occupation. It consists in tearing words from their mother context and setting them in rows — carrots and onions and beetroot and salsify next to one another — with roots shorn like those of celery to make them fit side by side, in an order determined not by nature but by some obscure Phoenician sailors who traded with Greeks in the long ago. Half of the lexicographer’s labor is spent repairing this damage to an infinitude of natural connections that every word in any language contracts with every other word, in a complex neural web knit densely at the center but ever more diffusely as it spreads outward. A bit of context, a synonym, a grammatical category, an etymology for remembrance’ sake, and a cross-reference or two — these are the additives that accomplish the repair.
Bolinger sketches — with rare insight as well as no small dose of wit — the lexicographic practice of taking individual words out of their natural textual environment and lining them up in a vertical list that in structuralist lexicographic theories is referred to as the macrostructure of a dictionary (Gouws, 2018).
What Bolinger does not do is to explain the reason for pursuing the distortion of language that he so aptly characterizes. In my view, the reason was a very practical one, guiding the design by a desire to create an efficient and usable work of reference. In the times long before hypertext and digital devices, text took the form of markings on paper (if not parchment, silk, tablets, or another usable surface), grouped into distinct strings of characters (or other symbols, depending on a particular writing system adopted). A properly designed work of reference should provide users with a way to navigate to the pertinent section. For this, early lexicography adopted a signposting system using distinct strings of characters, most typically words for word-based languages. They were arranged in a conventional order, that is alphabetical for languages having an alphabet. Non-alphabetic languages resorted to other ordering principles. For Chinese, for example, this could be by character properties (number of strokes or radical), pronunciation-based, or respelling-based. Going back to alphabetic languages, signposts in the form of words appeared as headings of dictionary entries, acting as gateways to the detailed information contained within a dictionary, serving as entry headwords. To simplify vertical scanning, these headwords were often typographically emphasized with bold formatting and/or hanging indentation. This whole arrangement followed a conventional system supposedly understood by dictionary users, although user studies launched in the late 20th century (e.g. Atkins, 1998) revealed this assumption to be overly optimistic.
Therefore, the structural distortions noted by Bolinger served the purpose of efficient retrieval of information arranged into conventional dictionary entries. Lexicographic conventions also governed the internal organization of the entries (termed entry microstructure). Dictionary users seeking lexical information needed a point of access to extended information nested under what was, by common (though generally inaccurate: compare the following section) perception, a minimal unit of meaning: the word.
From word to multi-word
The ‘repair’ process further mentioned by Bolinger has meant a gradual shift towards a more comprehensive treatment of meaning and structural units that extend beyond the word itself. Dictionaries spearheading this change started introducing into their entries phraseological units: phrases, lexical chunks, idioms, collocations, syntactic patterns, colligations, multi-word expressions, multi-word units, or multi-words, and a host of other terms for similar or distinct types of combinations of words. The trend was led by British monolingual dictionaries for learners of English (Cowie, 1999), and received a significant boost from the transition of dictionaries to the digital medium (Lew and de Schryver, 2014). The shift to the digital medium lifted many of the space constraints that had until then forced physical limits on the content that could be included in dictionaries. Of more relevance, a digital dictionary afforded access paths that were no longer dependent on a particular alphabetic (or other) ordering. Search terms could now be entered directly: first by typing into the search box, and more recently by dictating or snapping a picture, making alphabetic ordering irrelevant. Another development consisted in enhancing the findability of multi-word units. Implementations relying on a bag-of-words approach allowed users to find a multi-word unit by simply entering two of its component words in any order. Semantic tagging and word lists were added, affording semantically driven searches. Finally, large corpora were used as a source of additional example sentences to accompany entries. However, this has not always produced useful content, as at the end of the LDOCE Online entry for the phrasal verb do somebody/something over (https://www.ldoceonline.com/dictionary/do-over) shown below, where not even a single example sentence taken from the corpus is an actual illustration of this phrasal verb. Still, where examples do fit, they presumably strengthen the connection of the isolated headword with its network of possible textual contexts.
do over
-
You can transfer your pension scheme at any time, provided you do so over a year before you retire.
-
So do think this over carefully over the weekend-especially for the sake of the company-noblesse oblige and all that!
-
There was nothing to do but start over: I went into the hospital with two infections, pneumocystis and chicken pox.
-
It took him longer to get a grip on his feelings than it did to get over the climb from the beach.
-
If they were able to do this over the course of the whole meal-time they received a sticker on the chart.
-
Even men who have been accidentally thrown into primary child care do get over their resistance.
-
Of course, the rules of games do change over time.
Post-lexicography
So far, I have argued that dictionaries originated as a tool to help users resolve communication problems encountered in the context of text reception, production, and translation, usually (though not always) in cross-linguistic contexts. Until late last century, dictionaries were largely offered as printed books. Structurally, they may have consisted of several texts (megastructure), but the most important part would be a list of entries (also called, in some terminologies, articles) headed by headwords (entry lemmas), and arranged in an order facilitating access to the microstructure embedded within the entry. As explained above, it is the requirements of access structure that divorced dictionaries from the structure of running texts by breaking natural connections that words exhibit in normal text.
The digital revolution opened up new possibilities that lifted some of these limitations. The linear organization of entries was no longer needed, as the search term could be entered directly, by typing or (more recently) dictating; it would be looked up in an internal index and throw up (hopefully) relevant content. At first, the search box accepted headwords, but soon after, dictionary designers realized that the search terms need not be limited to headwords, and could broaden its scope to elements of the microstructure: phrases, examples, definitions, labels, etc. A well-known early adopter of the rich search options was the digital version of the OED (Brewer, 2013), which allowed the more demanding user to formulate complex searches filtered for specific microstructural elements, such as etymology, pronunciation, etc. Enhanced search options capable of identifying multi-word items have already received some discussion in the previous section.
Parallel to improvements in dictionaries, there was growing realization that technological progress now opened up the possibility of making lexical tools that were more fitting to specific tasks than the one-size-fits-all dictionary. One early version of such a tool was dubbed the leximat (Verlinde, 2009, Verlinde et al. 2010). The name did not stick, but the concept did. Let us now revisit the three basic contexts of dictionary use and consider the use of non-dictionary tools in those contexts.
Machine translation
Of the three canonical contexts of dictionary use (see The origins of lexicography above), text translation deserves first mention, as machine translation technology became more generally available early in the twenty-first century. The free Google Translate service launched in 2006, and although the initial translation quality left much to be desired (depending on the language pair involved), it was already the case that a large part of the job could be done automatically in a matter of seconds, with manual post-editing to refine the final translation. At present, the capability of machine translation (at least for language pairs with English), has reached a level that rivals (and in many cases surpasses) all but the most skilled human translators (Corpas Pastor, 2023). The traditional approach utilizing a dictionary for translation is not only time-consuming but also tends to result in a literal, word-for-word translation, oftentimes displaying unnatural collocational choices, L1-influenced paragraph structure, and possibly a host of other problems. Dictionaries, particularly specialized dictionaries, may still have a role in ensuring terminological precision in technical translations, but the emergence of term bases and translation memory systems has largely marginalized their use in this context (Reinke, 2018). To illustrate this point, I conducted a test using the free version of DeepL Translator (DeepL 2023), translating a two-paragraph description from Polish to English available on the home page of the Faculty of English of a leading Polish university. The resulting translation was, in my assessment, not only accurate, but also characterized by a more natural and idiomatic flow than a professional human translation until recently available on the English-language version of the unit’s website. Admittedly, an institutional web profile is not as much of a challenge to translate as would be, for example, culturally loaded or literary texts. My informal testing using a recent state-of-the-art handbook that lists tricky translation problems (Baker, 2018) shows that popular MT systems such as Google Translate of DeepL Translator can now handle some, but not all of such tricky cases.
There are many aspects to translation, and its evaluation is a science in its own right, with dimensions such as functional effectiveness and ability to convey cultural otherness (Asscher and Glikson, 2023). However, with relatively few exceptions, using dictionaries as the main translation tool no longer seems to be the most efficient or optimal strategy. Nowadays, there are specific tools designed for translation that have effectively replaced dictionaries as the primary resource for such tasks, at least in most everyday scenarios. Let us now turn our attention to lexical assistance in the context of text reception.
Text reception
Assisting in the understanding of challenging texts has traditionally been a key function of dictionaries. This type of challenge often arises when the text is in a language that is not the reader’s primary language, which ties back to the discussion in the section Machine translation above. In such cases, explanations in the reader’s primary language are usually the most effective.
Reading a text in a foreign language presents obvious challenges, but difficulty can also stem from technical vocabulary or unfamiliar domain-specific content in texts written in one’s first language. A good example of this was the medical texts emerging in large quantities during the acute phases of the recent COVID-19 pandemic, when many individuals who were not medical professionals began following primary medical literature. Much of this scholarly literature is ripe with esoteric specialized terminology and complex underlying concepts. In such scenarios, one might typically turn to a dictionary for assistance. In this context, I conducted a small experiment by using ChatGPT 3.5 (in its free version) to help interpret a complex abstract from the field of epidemic modelling. My one and only prompt asked the bot to explain the meaning of the complex term “susceptible-exposed-infectious-recovered metapopulation model” taken from an abstract of an academic paper published in the respectable journal Lancet (Wu et al. 2020). In my prompt, I cited an excerpt from this abstract as below (with the original typo):
Please explain what “A susceptible-exposed-infectious-recovered metapopulation model” is in the following excerpt from an article abstract: Serial interval estimates were based on previous studies of severe acute respiratory syndrome coronavirus (SARS-CoV). A susceptible-exposed-infectious-recovered metapopulation model was used to simulate the epidemics across all major cities in China. The basic reproductive number was estimated using Markov Chain Monte Carlo methods and presented using the resulting posterior mean and 95% credibile [sic!] interval (CrI).
Quite a few of the terms used in the abstract are too specialized to be found in a general dictionary, and some, like the very long multi-word expression in question, not even in a specialized dictionary of medical terminology. By contrast, ChatGPT 3.5 (in its free version) provided a popularized yet quite comprehensive explanation, given below in full:
In the given excerpt from the article abstract, a “susceptible-exposed-infectious-recovered metapopulation model” is a mathematical model used to simulate the spread of an infectious disease within a population. Let’s break down what each component of this model means:
1. Susceptible (S): This refers to individuals in the population who are not infected with the disease but can become infected if they are exposed to the infectious agent (e.g., a virus).
2. Exposed (E): These are individuals who have been exposed to the infectious agent but are not yet infectious themselves. This stage represents the incubation period when an individual has been in contact with the disease but is not yet showing symptoms or capable of transmitting it to others.
3. Infectious (I): This group comprises individuals who are infected with the disease and capable of transmitting it to others.
4. Recovered (R): These individuals have recovered from the disease and are no longer susceptible to it. In some cases, they may also be immune to reinfection.
“Metapopulation” in this context refers to the fact that the model considers not just a single, homogeneous population but multiple populations in different geographical locations, such as major cities in China. It accounts for the interaction between these populations, as the spread of infectious diseases often involves the movement of infected individuals between different areas.
The model is used to simulate how the disease spreads over time in various cities in China, taking into account the stages of susceptibility, exposure, infection, and recovery. By using this model, researchers can estimate the basic reproductive number (R0), which is a key epidemiological parameter that indicates how many people, on average, one infected individual will transmit the disease to. The estimation of R0 is performed using statistical methods, specifically Markov Chain Monte Carlo (MCMC) methods, and the results are presented as a posterior mean (a measure of central tendency) along with a 95% credible interval (CrI), which represents the range of plausible values for R0. This information is valuable for understanding and controlling the epidemic’s dynamics and informing public health measures.
ChatGPT systematically deconstructed the complex term, explaining not just its meaning as such, but also its role and function in the very research reported — which, after all, is exactly what a reader might wish for. The explanation was both factually correct and delivered in a register appropriate for popular-science discourse. In addition, the bot paraphrased in simpler terms the remainder of the passage, apparently unfazed by the typo “credibile” for “credible”, and correcting it while at it. This example, though anecdotal, gives a fairly clear idea of the typical performance of the free version of ChatGPT as of this writing. We might reasonably expect further improvement in performance in the coming months, although it is hard to think what could have been done better in this case (but see the Explain mode of AcademicGPT, Petersson, 2024). As it is, it is more than clear that no dictionary could have done this task with anything approaching the performance displayed.
Text production and writing assistants
Following translation and text reception, text production is the last of the three canonical types of situations linked to dictionary use. By its very nature, production presents a greater challenge than comprehension. In the latter, the text is already out there, and all the reader needs is to figure out what the existing text is saying, thus essentially a passive task. And, since the text is given, it can readily be used to cue lexical tools: in small or longer fragments, depending on what the tool at hand is capable of.
Production is different. In production, the message is incipient in the writer’s mind but needs to be made more specific and be given linguistic substance in the form of structures and words. Again, the level of difficulty will normally be greater if the encoding is to be done into what is an additional language for the writer. In such a case, and if the message is relatively straightforward, the writing task again reduces to translation, this time going from the writer’s first language to their additional language. Here, bilingual L1➜ L2 dictionaries have traditionally been used, even though few offer sufficient support for text production (Adamska-Sałaciak, 2010, Lew and Adamska-Sałaciak, 2015). However, writing is a difficult creative task in itself which can be a challenge even in the native language. In such cases, bilingual dictionaries will not be useful, as there is no higher-proficiency language for the writer that could ease the task. Monolingual dictionaries are also of limited use in writing, as there is no easy way to find the relevant entries in such dictionaries: canonical dictionaries are semasiological, built for a search from form to meaning, which is the exact reverse of what is needed for writing. Onomasiological dictionaries do exist, in the form of synonym dictionaries, thesauri, and related word finders, but their search mechanisms are highly unreliable in practice, with users running the risk of wasting time only to get nowhere. The inadequacy of dictionaries in supporting writing tasks is what led to the development of dedicated writing assistants in the last decade or two (Verlinde and Peeters, 2012, Granger and Paquot, 2015, Tarp et al. 2017, Alonso-Ramos and García Salido, 2019, Frankenberg-Garcia et al. 2019, Tarp, 2023). An important factor to consider is the high cognitive demand that the writing process puts on the writer (especially so in their additional language). Therefore, effective tools for writing should tie up as little of the writer’s attention as possible, rather than distract them from the main task. This is an aspect in which AI tools might hold much promise.
Writing assistants can support the user in the process of writing as it happens, or they can be used for post-editing manual drafts. Tarp (2023) lists the following types of functionalities found in writing assistants:
-
(1)
detection of possible problems in the written text
-
(2)
correction with suggestions for alternative solutions
-
(3)
prediction of word terminations and next words
-
(4)
transformation of syntax, style, sentences, etc.
-
(5)
translation with provision of L2 equivalents
-
(6)
consultation with look-ups in lexicographical databases.
In Tarp’s assessment, there was no writing assistant at the time of writing that would include all of these six functions. With generative AI at our disposal, it may be possible to delegate more of the task to a trained system. In another of my small-scale experiments, I tasked ChatGPT 4 with drafting a letter to a (fictitious) conference organizer, asking for the conference fee to be waived or reduced. The prompt was in Polish and consisted of just three short sentences as below. These sentences translat into English as follows: “In an email in English, ask the organisers of the WorldLex 2024 conference to waive the conference fee, or at least reduce it. Reason: my university did not receive funding from the government because the president blocked the budget bill. I am very keen to participate.”
You
W emailu poproś po angielsku organizatorów konferencji WorldLex 2024 o odstąpienie od opłaty konferencyjnej lub przynajmniej obniżenie jej. Powód: moja uczelnia nie otrzymała funduszy od rządu, bo prezydent zablokował ustawę budżetową. Bardzo mi zależy na udziale.
ChatGPT
Certainly, here’s a draft for your email to the organizers of the WorldLex 2024 conference:
Subject: Request for Waiver or Reduction of Conference Fee for WorldLex 2024
Dear WorldLex 2024 Organizers,
I hope this message finds you well. My name is [Your Name], a professor at Adam Mickiewicz University in Poznań. I am writing to you regarding the upcoming WorldLex 2024 conference, an event I have been eagerly looking forward to attending.
Unfortunately, I am currently facing a significant financial constraint that hinders my ability to cover the conference fee. Due to recent developments in our national budget, where the President has blocked the budget bill, my university has not received the anticipated government funding. This unexpected turn of events has impacted our financial planning, particularly in supporting faculty participation in international academic events.
Given these circumstances, I am kindly requesting if it would be possible to consider a waiver or at least a reduction of the conference fee for my attendance at WorldLex 2024. My participation in this conference is of great importance to me. It offers a vital opportunity to engage with the latest developments in our field, contribute to scholarly discussions, and bring back invaluable insights to my students, many of whom are future English language educators.
I understand the challenges and costs involved in organizing such a prestigious event and appreciate any assistance you can provide in this matter. I am hopeful for a positive response and look forward to the possibility of contributing to the success of WorldLex 2024.
Thank you very much for considering my request. I am looking forward to your reply.
Best regards,
[Your Full Name]
[Your Position]
Faculty of English
Adam Mickiewicz University
[Your Contact Information]
The substantial message that ChatGPT produced appeared basically good to go. Should this not be the case, further refining (fine-tuning) could easily follow by adding just a sentence of instruction on how the request should be modified. Still, on the first attempt, the email was appropriately formal, well-suited to its pleading pragmatic character, and written in idiomatic English. The sensitive task was accomplished within seconds, and the quality of the outcome is already much better than a non-native speaker of English with just a working knowledge of English in their professional domain could ever attain using the best dictionaries.
My ad hoc but hopefully realistic survey of how post-lexicographic tools can tackle the three canonical types of tasks (i.e., text reception; translation; and text production) suggests that AI-powered tools — even those available free of charge and not trained for these specific tasks — appear to be able to achieve significant success in all these tasks traditionally handled by consulting dictionaries. In addition, they do that in a fraction of the time it would take the most skilful of dictionary users.
Discussion and outlook
So far, we have learned from recent studies that generative pre-trained transformers are capable of producing good-quality dictionary entries (De Schryver, 2023, Lew, 2023, Rees and Lew, 2023). It is, however, time to question the point behind continuing to create complex and “unnatural” (pace Bolinger) tools, when we could more easily and more directly deal with our lexical challenges by interacting with an intelligent agent. Preliminary results (Ptasznik and Lew Submitted) from a study with 223 university students showed that the free version of ChatGPT performed much better than a mobile web version of the excellent LDOCE dictionary on both reception and production tasks. These results are shown graphically as success rates estimated by a mixed-effects model in Fig. 1.
An aspect that cuts across the rough division into task types (reception, production, and translation) that I have not discussed here, but which calls for investigation, is accessibility to people with impairments, be it cognitive or physical (as well as children). This is an aspect that has recently received some attention (Arias-Badia and Torner, 2023, Rees, 2023), and the message so far is that digital dictionaries are not necessarily as accessible as we might wish. From that point of view, conversational chatbots are in principle able to adjust both the level of technicality (the now famous ‘explain to me like I’m a ten-year-old’), and the modality of communication (e.g. via voice: speaking and listening).
Way forward: What is left for lexicography?
Having completed this brief tour of lexicography, in the context of recent AI-related technological developments, we might ask: Is lexicography still relevant today? Even though AI-driven tools like OpenAI’s GPT, which powers ChatGPT, and machine translation systems have made huge progress in tasks traditionally done with dictionaries, such as text reception, production, and translation, there may yet be a niche for lexicography.
One critical area where traditional lexicography retains its relevance is in any languages with limited digital presence. Admittedly, GPT and similar Large Language Models demonstrate remarkable proficiency in English, but their capabilities in other languages, especially minor or less-documented ones, are not nearly as impressive (Bang et al. 2023, Lai et al. 2023). This is largely due to the scarcity of substantial data in these languages that is required for training these models. Here, human lexicographers may have an advantage, particularly in their ability to generalize and extrapolate from limited datasets. Consequently, lexicography remains vital for bridging linguistic divides and preserving the linguistic diversity of these smaller language communities. Additionally, AI can play a supportive role in fieldwork related to these languages, such as in conducting field interviews with native speakers of indigenous languages, thereby aiding in data collection and preservation.
Furthermore, lexicography continues to have a crucial role in projects that depend on non-digitized sources. This includes historical and diachronic lexicography insofar as it relies on traditional scholarship and paper-based archives. These areas, not yet fully embraced by digital technology, offer a fertile ground for lexicographic work.
While the scope of lexicography seems to be narrowing, the field is not facing obsolescence. In the foreseeable future, lexical projects, even those incorporating machine learning, will still require expert oversight. This oversight involves setting project objectives, guiding the AI’s learning process, and critically assessing its output. Lexicographers are well-positioned to provide this expertise, although the demand for their skills might diminish compared to past requirements. Moreover, the role of lexicographers is evolving, necessitating a shift towards a more interdisciplinary approach that blends traditional lexicographic skills with knowledge of computational linguistics, big data, and data science (compare Grabowski, 2023).
The field of lexicography is transitioning rather than disappearing. As we integrate machine learning and AI into the lexicon, there’s a transformative shift in how lexicographic content is produced, managed, and utilized. This does not render the role of the lexicographer obsolete but instead reshapes it. The future may see lexicographers as curators and analysts of linguistic data, guiding AI to better understand and process less-represented languages and complex linguistic phenomena. They might also play a pivotal role in overseeing the ethical and accurate representation of languages and dialects in digital platforms, ensuring linguistic diversity and cultural sensitivity.
This redefined role of lexicographers could extend to collaborating with technologists in developing AI models that are more inclusive of diverse linguistic data, thus enhancing the AI’s ability to understand and generate text in a broader array of languages (see e.g. Adebara et al. 2024). Moreover, their expertise will be crucial in identifying and correcting biases in language models (Navigli et al. 2023), thereby contributing to the development of more equitable and representative language technologies.
There is little doubt that the nature of lexicography must change in the face of advanced AI and machine learning, yet there remains a clear and evolving need for the expertise and insight that lexicographers provide. Their role is not so much diminishing as it is adapting to the new linguistic landscape shaped by technology, where their skills are essential for ensuring the richness, accuracy, and ethical use of linguistic data in an increasingly digital world.
Data availability
This article does not rely on any analysed or generated data beyond that given in the text of the article.
References
Adamska-Sałaciak A (2015) Bilingual lexicography: Translation dictionaries. In: Hanks P, De Schryver G-M (Eds) International Handbook of Modern Lexis and Lexicography. Springer, 1–11
Adamska-Sałaciak A (2010) Why we need bilingual learners’ dictionaries. In: Kernerman IJ, Bogaards P (Eds.) English learners’ dictionaries at the DSNA 2009. K Dictionaries, Tel Aviv, 121–137. Available from: Adamska_2010 Why we need bilingual learners dictionaries.pdf
Adebara I, Elmadany A, Abdul-Mageed M (2024) Cheetah: Natural Language Generation for 517 African Languages. https://doi.org/10.48550/ARXIV.2401.01053
Alonso-Ramos M, García Salido M (2019) Testing the use of a collocation retrieval tool without prior training by learners of Spanish. Int J Lexicogr 32:480–497. https://doi.org/10.1093/ijl/ecz016
Arias-Badia B, Torner S (2023) Bridging the gap between website accessibility and lexicography: information access in online dictionaries. Universal Access in the Information Society. https://doi.org/10.1007/s10209-023-01031-9
Asscher O, Glikson E (2023) Human evaluations of machine translation in an ethically charged situation. N. Media Soc 25:1087–1107. https://doi.org/10.1177/14614448211018833
Atkins BTS (Ed.) (1998) Using dictionaries. Studies of dictionary use by language learners and translators. Niemeyer, Tübingen
Augustyn P (2013) No dictionaries in the classroom: Translation equivalents and vocabulary acquisition. Int J Lexicogr 26:362–385. https://doi.org/10.1093/ijl/ect017
Baker M (2018) In Other Words: A Coursebook on Translation. 3rd ed. Routledge, Third edition. Routledge, Abingdon, Oxon; New York, NY, p 2017. 10.4324/9781315619187
Bang Y, Cahyawijaya S, Lee N, Dai W, Su D, Wilie B, Lovenia H, Ji Z, Yu T, Chung W, Do QV, Xu Y, Fung P (2023) A Multitask, Multilingual, Multimodal Evaluation of ChatGPT on Reasoning, Hallucination, and Interactivity. https://doi.org/10.48550/ARXIV.2302.04023
Bolinger D (1985) Defining the undefinable. In: Ilson RF (Ed.) Dictionaries, lexicography and language learning. Pergamon Press, Oxford, p 69–73
Brewer C (2013) OED Online re-launched: Distinguishing old scholarship from new. Dictionaries: J Dict Soc North Am 34:101–126. https://doi.org/10.1353/dic.2013.0002
Corpas Pastor G (2023) At a loss with technology? Some current research initiatives to assist (or even replace) interpreters
Cowie AP (1999) English dictionaries for foreign learners: A history. Clarendon Press, Oxford
DeepL (2023) DeepL Translator. Available from: https://www.deepl.com/en/translator
Frankenberg-Garcia A (2018) Investigating the collocations available to EAP writers. J Engl Acad Purp 35:93–104. https://doi.org/10.1016/j.jeap.2018.07.003
Frankenberg-Garcia A, Lew R, Roberts JC, Rees GP, Sharma N (2019) Developing a writing assistant to help EAP writers with collocations in real time. Recall 31:23–39. https://doi.org/10.1017/S0958344018000150
Gouws RH (2018) Dictionaries and Access. In: Fuertes-Olivera PA (Ed.) The Routledge Handbook of Lexicography. Routledge Handbooks in Linguistics. Routledge, London, p 43–58. https://www.routledge.com/The-Routledge-Handbook-of-Lexicography/Fuertes-Olivera/p/book/9781138941601
Grabowski Ł (2023) Statistician, programmer, data scientist? Who is, or should be, a Corpus linguist in the 2020s? J Linguist/Jazykovedný Cas 74:52–59. https://doi.org/10.2478/jazcas-2023-0023
Granger S, Paquot M (2015) Electronic lexicography goes local: Design and structures of a needs-driven online academic writing aid / Die elektronische Lexikographie wird spezifischer: Das Design und die Struktur einer auf die Benutzerbedürfnisse berzogenen akademischen Online- Schreibhilfe / La lexicographie électronique devient plus spécifique: conception et structure d’une aide à l‘écriture académique. Lexicographica 31:118–141. https://doi.org/10.1515/lexi-2015-0007
Hyland K, Shaw P (2016) Introduction. In: Hyland K, Shaw P (Eds) The Routledge Handbook of English for Academic Purposes. Routledge, London, p 1–14
Lai VD, Ngo NT, Veyseh APB, Man H, Dernoncourt F, Bui T, Nguyen TH (2023) ChatGPT Beyond English: Towards a comprehensive evaluation of large language models in multilingual learning. https://doi.org/10.48550/ARXIV.2304.05613
Lew R (2023) ChatGPT as a COBUILD lexicographer. Hum Soc Sci Commun 10:704. https://doi.org/10.1057/s41599-023-02119-6
Lew R, Adamska-Sałaciak A (2015) A case for bilingual learners’ dictionaries. ELT J 69:47–57. https://doi.org/10.1093/elt/ccu038
Lew R, de Schryver G-M (2014) Dictionary users in the digital revolution. Int J Lexicogr 27:341–359. https://doi.org/10.1093/ijl/ecu011
Navigli R, Conia S, Ross B (2023) Biases in large language models: origins, inventory, and discussion. J Data Inf Qual 15:1–21. https://doi.org/10.1145/3597307
Petersson L (2024) AcademicGPT. Available from: https://academicgpt.net/
Ptasznik B, Lew R (Submitted) A learners’ dictionary versus ChatGPT in receptive and productive lexical tasks
Rees GP (2023) Online dictionaries and accessibility for people with visual impairments. Int J Lexicogr 36:107–132. https://doi.org/10.1093/ijl/ecac021
Rees GP, Lew R (2023) The effectiveness of OpenAI GPT-generated definitions versus definitions from an English learners’ dictionary in a lexically orientated reading task. Int J Lexicogr 37:50–74. https://doi.org/10.1093/ijl/ecad030
Reinke U (2018) State of the art in translation memory technology. In: Rehm G, Sasaki F, Stein D, Witt A (Eds) Language technologies for a multilingual Europe. Language Science Press, Berlin, p 55–84. 10.5281/ZENODO.1291930
Rundell M (1999) Dictionary use in production. Int J Lexicogr 12:35–53
Scholfield P (1999) Dictionary use in reception. Int J Lexicogr 12:13–34
De Schryver G-M (2023) Generative AI and Lexicography: The Current state of the art using ChatGPT. Int J Lexicogr: ecad021. https://doi.org/10.1093/ijl/ecad021
Tarp S (2008) Lexicography in the borderland between knowledge and non-knowledge: General lexicographical theory with particular focus on learner’s lexicography. Max Niemeyer Verlag, Tübingen
Tarp S (2023) Eppur si muove: Lexicography is Becoming Intelligent. Lexikos 33:107–131. https://doi.org/10.5788/33-2-1841
Tarp S, Fisker K, Sepstrup P (2017) L2 Writing assistants and context-aware dictionaries: new challenges to lexicography. Lexikos 27:494–521. https://doi.org/10.5788/27-1-1412
Tarp S (2004) How can dictionaries assist translators? In: Sin-wai C (Ed.) Translation and bilingual dictionaries. Lexicographica Series Maior 119, Niemeyer, Tübingen, p 23–38
Verlinde S (2009) The Base Lexicale Du Français: a Multi-Purpose Lexicographic Tool. In: Granger S, Paquot M (Eds) Proceedings of eLex 2009, Louvain-la-Neuve, 22-24 October 2009. Cahiers du Cental, 7. UCL Presses, Louvain-la-Neuve, p 335–342. https://pul.uclouvain.be/Resources/titles/29303100621500/extras/82577-Cental-Fairon-cahier7-INT-V3.pdf#page=347
Verlinde S, Leroyer P, Binon J (2010) Search and you will find. from stand-alone lexicographic tools to user driven task and problem-oriented multifunctional leximats. Int J Lexicogr 23:1–17. https://doi.org/10.1093/ijl/ecp029
Verlinde S, Peeters G (2012) Data access revisited: The Interactive Language Toolbox. In: Granger S, Paquot M (Eds) Electronic lexicography. Oxford University Press, Oxford, p 147–162
Webb S (2008) Receptive and Productive Vocabulary Sizes of L2 Learners. Studies in Second Language Acquisition 30. https://doi.org/10.1017/S0272263108080042
Wu JT, Leung K, Leung GM (2020) Nowcasting and forecasting the potential domestic and international spread of the 2019-nCoV outbreak originating in Wuhan, China: a modelling study. Lancet 395:689–697. https://doi.org/10.1016/S0140-6736(20)30260-9
Author information
Authors and Affiliations
Contributions
RL: Conceptualization, Data curation, Formal Analysis, Funding Acquisition, Investigation, Methodology, Project administration, Resources, Software, Supervision, Validation, Writing – original draft, Writing – review & editing.
Corresponding author
Ethics declarations
Competing interests
The author declares no competing interests.
Ethics approval
Ethics approval was not required as the study did not involve human participants.
Informed consent
Informed consent was not required or possible as the study did not involve human participants.
Additional information
Publisher’s note Springer Nature remains neutral with regard to jurisdictional claims in published maps and institutional affiliations.
Rights and permissions
Open Access This article is licensed under a Creative Commons Attribution 4.0 International License, which permits use, sharing, adaptation, distribution and reproduction in any medium or format, as long as you give appropriate credit to the original author(s) and the source, provide a link to the Creative Commons licence, and indicate if changes were made. The images or other third party material in this article are included in the article’s Creative Commons licence, unless indicated otherwise in a credit line to the material. If material is not included in the article’s Creative Commons licence and your intended use is not permitted by statutory regulation or exceeds the permitted use, you will need to obtain permission directly from the copyright holder. To view a copy of this licence, visit http://creativecommons.org/licenses/by/4.0/.
About this article
Cite this article
Lew, R. Dictionaries and lexicography in the AI era. Humanit Soc Sci Commun 11, 426 (2024). https://doi.org/10.1057/s41599-024-02889-7
Received:
Accepted:
Published:
DOI: https://doi.org/10.1057/s41599-024-02889-7