Abstract
Flood-prone people and decision-makers are often unwilling to discuss and prepare for exceptional events, as such events are hard to perceive and out of experience for most people. Once an exceptional flood occurs, affected people and decision-makers are able to learn from this event. However, this learning is often focussed narrowly on the specific disaster experienced, thus missing an opportunity to explore and prepare for even more severe, or different, events. We propose spatial counterfactual floods as a means to motivate society to discuss exceptional events and suitable risk management strategies. We generate a set of extreme floods across Germany by shifting observed rainfall events in space and then propagating these shifted fields through a flood model. We argue that the storm tracks that caused past floods could have developed several tens of km away from the actual tracks. The set of spatial counterfactual floods generated contains events which are more than twice as severe as the most disastrous flood since 1950 in Germany. Moreover, regions that have been spared from havoc in the past should not feel safe, as they could have been badly hit as well. We propose spatial counterfactuals as a suitable approach to overcome society's unwillingness to think about and prepare for exceptional floods expected to occur more frequently in a warmer world.
Export citation and abstract BibTeX RIS

Original content from this work may be used under the terms of the Creative Commons Attribution 4.0 license. Any further distribution of this work must maintain attribution to the author(s) and the title of the work, journal citation and DOI.
1. Introduction
Flooding is the natural hazard which affects more people globally than any other natural hazard (CRED and UNDRR 2019). The July 2021 flood in Western Europe alone caused more than 220 fatalities and almost € 50 billion damage. Climate change, socio-economic and population growth are expected to further increase disastrous flood impacts (Merz et al 2021). Despite tremendous impacts of exceptional floods in recent decades and their projected, more frequent occurrence in the future, society is often unwilling to discuss and prepare for such events (de Bruijn et al 2022).
We use the term exceptional flood to characterize events that are rare and have the potential to generate disastrous impacts. For Germany, this translates often into floods with return periods larger than 100 years, as many rivers have flood protection against the 100 year flood. For those rivers without structural flood defences, exceptional floods can also include smaller events, such as the 50 year flood. However, the flood needs to be rare enough, so that flood-prone people experience it as exceptional.
The unwillingness to discuss and prepare for exceptional floods starkly contrasts their relevance for society (Merz et al 2009). When they occur, they are either unprecedented or they are perceived as unprecedented, as their previous occurrence is out of memory. For instance, the Western European flood in July 2021 caused 134 fatalities and huge havoc in the Ahr river valley. Its flood peak at the streamflow gauge Altenahr-Ahrweiler was approximately five times larger than the flood-of-record of the last seven decades. However, reconstructions of historical floods showed that a flood in 1804 had the same peak flow (Roggenkamp and Herget 2014). In both cases (unprecedented or perceived as unprecedented), society tends to be surprised and little prepared, which leads to disastrous impacts (Kreibich et al 2022).
Although damages cannot completely be prevented when exceptional floods occur, risk management can limit disastrous impacts. Forecasting, early warning and evacuation schemes can prevent fatalities. Spatial planning and infrastructure management can ensure that sensitive infrastructure (e.g. homes for elderly care) and critical infrastructure (e.g. power plants) are either not located in hazardous zones or are waterproofed and protected against inundation. Further, infrastructure management can design backup and redundancy measures for continuous operation during inundation and develop measures to rapidly return to minimum service levels when failure cannot be prevented (Koks et al 2022).
There exist a range of approaches to develop scenarios of exceptional events (Paté-Cornell 2012, Merz et al 2015, Albano et al 2016, Woo 2019). This range includes: (1) extrapolation from frequent floods to exceptional events using statistical or simulation models. (2) Stochastic Simulation, i.e. embedding process models in a stochastic environment and generate very large event sets to search for exceptional floods. (3) Perfect Storms, i.e. searching for the most unfavorable superposition of processes that lead to inundation. (4) Storylines, i.e. developing scenarios based on expert knowledge. (5) Downward Counterfactuals, i.e. using past events to develop scenarios where things turn out for the worse.
A problem with exceptional events is that people cannot easily relate to such situations. People cannot predict the negative effects of severe flooding, when they have not experienced it (Siegrist and Gutscher 2008). Further, people tend to evaluate the probability of an event according to the ease with which they can imagine such an event (availability bias, Merz et al 2015). Thus people might assign a probability of zero to events they find very hard to imagine. Moreover, people and organizations find it difficult to think about threatening prospects and to plan for situations that would be damaging to them (Bunn and Salo 1993).
Information about exceptional floods is often provided in the form of flood maps. For instance, European Union member states are obliged to map an extreme flood scenario for flood-prone areas. Its return period varies within the EU; in Germany it ranges from 200 to 1 000 years. These maps are useful for a range of purposes, but they are difficult to interpret for lay people, also because they cannot easily relate to the abstract concept of the 500 year flood area (Meyer et al 2012, Percival et al 2020). In addition, these maps do not show events with a spatial footprint, but are obtained by aggregating local assessments. The resulting flood maps do not show plausible flood events (Nguyen et al 2020), but spatially homogeneous situations that will never occur in this way. They are thus of limited help, for instance, for disaster management that is required to prepare for large-scale events.
Motivating society to think about and prepare for exceptional events requires a vehicle to overcome this unwillingness. We argue that spatial counterfactuals, where an observed precipitation field is shifted in space, offer a straightforward approach for this purpose. We assume that even for lay people it is easy to understand that a past storm track that has hit a certain region could have developed in a slightly different way. Shifting observed event precipitation offers new, and possibly exceptional, flood scenarios for the affected region and prevents it from falling prey to too focussed learning. In addition, it can convince neighboring regions that have been spared by this event, that they were simply lucky.
In this paper, we systematically explore the use of spatial counterfactuals to develop exceptional flood scenarios. To this end, we select ten past flood disasters, shift the event precipitation in space, and analyse how this shift impacts flooding across Germany.
2. Study area
Germany has a long history of devastating flooding. For instance, intense precipitation in December 1993 saturated soils in the Rhine catchment (figure 1). Widespread, and partially extreme, precipitation starting on 19 December then led to the Christmas flood in the middle and lower Rhine basin, causing several fatalities, inundations in three federal states, and more than 13 500 flood-affected households in Cologne alone. Another disaster was the August 2002 flood in the Elbe and Danube catchments. Extreme precipitation and associated flooding caused more than 130 dike breaches, 21 fatalities and losses of € 14.9 billion (in values of 2013; Kreibich et al 2017). The most expensive disaster in German history is the July 2021 flood in Western Europe. Widespread intensive rainfall led to flash floods in many small and medium sized catchments; many of them experienced their flood-of-record. The high number of fatalities and tremendous damage (in Germany alone: 191 fatalities; € 33 billion damage) confirm that flood risk management is not well prepared for exceptional floods (Thieken et al 2023).
Figure 1. Main river basins (Rhine, Elbe, Danube, Weser, Ems) and river network of Germany. Yellow circles indicate the location of the 516 gauges used to calculate the flood event severity.
Download figure:
Standard image High-resolution imageThe timing of flooding in Germany shows a distinct spatial pattern (Beurton and Thieken 2009). In the central and western parts of Germany, almost all floods occur in winter, while the pre-alpine areas in southern Germany are dominated by summer floods. The northern and eastern regions are mostly affected by winter flooding. However, the fraction of spring and summer floods is substantial, and the most disastrous floods in these regions were summer events.
3. Methods and data
To develop a plausible set of exceptional flood scenarios, we select ten past flood disasters. We shift the event precipitation in space using three distances and eight directions, yielding 24 (3 × 8) counterfactual precipitation events. These events and the factual event are used as atmospheric forcing for a hydrological model, calibrated for flooding in Germany. Finally, we analyse how these spatial shifts affect the flood severity in Germany. The data supporting this research is published as data publication (Nguyen et al 2024).
3.1. Selecting flood events and shifting event precipitation
We selected the eleven river floods in Germany with the highest damage from the HANZE database (Paprotny et al 2018). We did not include events before 1950, as data availability, particularly for streamflow, is low in Germany prior to the 1950s. From this list, we deleted the Oder flood in 1997 and the flood in June 1994. The Oder marks the border between Germany and Poland and is not included in our flood model. For the 1994 flood the affected river basin is not given in the HANZE database, and our model does not show evidence of severe flooding during this period. However, we added the July 2021, i.e. the most expensive disaster in Germany. For each of these ten events, we define the start day of the flood event as given in the HANZE database and from available rainfall and streamflow data for the 2021 flood (table 1). To ensure that the event precipitation is captured completely across Germany, we define the shifting day as seven d prior to the event start day. Starting from the shifting day, we use the shifted precipitation to force the flood model. The selected floods contain winter, spring and summer events and represent the spectrum of flood timing in Germany.
Table 1. Selected river flood disasters affecting Germany. Losses are taken from the HANZE dataset (Paprotny et al 2018).
Flood | Start day | Affected river basins | Losses (million € in 2011 values) |
---|---|---|---|
1978, May | 22/05/1978 | Rhine, Neckar | 695 |
1984, February | 06/02/1984 | Saar, Main, Saale, Mosel, Rhein, Neckar | 172 |
1993, December | 20/12/1993 | Mosel, Saar, Rhine, Neckar, Aisch, Nahe | 762 |
1994, April | 13/04/1994 | Bode, Saale, Wipper | 178 |
1995, January | 22/01/1995 | Mosel, Rhine, Neckar, Nahe, Main, Saar | 321 |
1998, October | 30/10/1998 | Main, Tauber, Mosel, Hase, Ilse, Oker | 155 |
1999, May | 12/05/1999 | Isar, Amper, Ammer, Wertach, Lech, Iller, Vils, Inn, Danube | 458 |
2002, August | 11/08/2002 | Elbe, Danube, Mulde | 9.952 |
2013, May | 28/05/2013 | Danube, Elbe, Saale, Mulde | 6.452 |
2021, July | 12/07/2021 | Rhine basins (left tributaries mainly; e.g. Ahr Erft, Rur) | 33.000 |
a in 2021 values (Mohr et al 2023).
For each of the ten events, we consider 25 precipitation scenarios, consisting of the observed (no-shift) precipitation and 24 shifted precipitation fields. To this end, we shift the observed precipitation in eight directions (N, NE, E, SE, S, SW, W, NW) by three distances (20, 50 and 100 km).
Shifting the event precipitation fields up to 100 km in all directions is well justified given the process scales and mechanisms involved. The paths of precipitation-generating low-pressure systems are dominated by non-linear interaction with synoptic- and larger-scale phenomena (at scales of ∼1 000 km or more). Cyclone tracks do not have fixed trajectories as previously assumed (van Bebber 1891). For example, Vb cyclone tracks can yield extreme precipitation at locations scattered across Central Europe, and the Atlantic winter cyclone path type impacts precipitation all over western Germany (Hofstätter et al 2016, Akhtar et al 2019).
Therefore, the precipitation fields of single events might unfold differently, given a slightly different synoptic situation. For example, the major moisture transport trajectory responsible for the 2021 flood was located on the western flank of a synoptic-scale persistent blocking system (Mohr et al 2023). A slight change in the blocking position or extension would have implied a shift in the moisture transport trajectory and the associated precipitation pattern of a few ten km, still influenced by orographic enhancement. The orography impacts the precipitation amounts in case of long-lasting events (>12 h), especially in the area of the Black Forest in southwest Germany, the Ore mountains in southeast Germany and the European Alps with length scales of ∼100 km and larger. This constraint is, however, less effective for precipitation events with larger return periods (e.g. five years, as demonstrated by Lengfeld et al 2021, using 20 years of German weather radar data).
3.2. Hydrological modelling for Germany
We apply the grid-based mesoscale hydrologic model (mHM) (Samaniego et al 2010, Kumar et al 2013); specifically, the implementation of Samaniego et al (2019), which considers land use changes by using four historical land use layers, with spatial resolution of five km and daily time step. mHM is forced using the E-OBS product with spatial resolution of 0.25° covering Germany and the headwater parts in neighbouring countries for the period 01/1950–12/2021 at daily resolution. We interpolate the E-OBS data to obtain five km resolution as input for mHM. mHM utilizes a seamless multiscale parameterization regionalization to generate a spatially consistent set of regionalized model parameter fields, enabling a more accurate representation of the spatial patterns of hydrological processes.
To ensure the reliability of our findings, a multi-basin optimization is performed across a wide range of hydrologic regimes throughout Germany using twelve river gauges across the major river basins during the ten year period 01/2000–12/2009. The optimization utilizes the dynamically dimensioned search algorithm (Tolson and Shoemaker 2007) to calibrate 24 mHM transfer-function parameters globally. As objective function, the weighted Nash–Sutcliffe Efficiency (wNSE) that is highly focused on flood peaks is applied (Hundecha and Merz 2012).
In this way, we obtain a spatially consistent parameter set that can represent the hydrological processes across Germany. The performance of the calibrated model is assessed for 119 gauges across entire Germany over the period 01/1985–12/1994. Some smaller catchments show poor performance due to the coarse resolution of the meteorological forcing (0.25°) and the hydrological modelling resolution (5 km). However, the median values of wNSE (0.64) and KGE (0.44) indicate that the calibrated parameter set can effectively represent the observed discharge and especially the high flows for multiple catchments across Germany.
The validated mHM version is forced by observed precipitation for 01/1950–12/2021 to obtain the baseline flood situation for Germany. The spatial counterfactuals for a given event are generated by running mHM with observed precipitation until the specific shifting day. Then the observed precipitation is replaced by the shifted precipitation fields for a period of three weeks. In this way, the shifted precipitation fields are combined with the actual antecedent catchment conditions.
3.3. Quantifying the severity of flooding
For quantifying the severity of flood events, we compile a dataset of 516 streamflow gauges (figure 1). We use the simulated streamflow, whereas mHM is driven by observed meteorology, to derive the annual maximum flow for each gauge. Then we estimate the flood frequency by fitting the generalised extreme value distribution to the annual maxima via the maximum likelihood method. These flood frequency curves are then used to assign a return period to each gauge for each flood event. We search for the highest streamflow value within three weeks after the event start day and transfer this streamflow value into the respective return period based on the flood frequency curve of the gauge.
To obtain a quantitative indicator of the severity of floods, that affect several rivers and tributaries, we introduce the severity indicator SI:
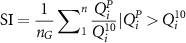
where nG
= 516 (total number of gauges), is the peak flow of the event maximum at gauge i, and
is the 10 year flood quantile at gauge i. SI considers only gauges that have a peak larger than the 10 year flood. Smaller peaks are assumed to be irrelevant for flood damages in Germany. SI combines the local severity (by considering the local flood peaks normalised by the 10 year flood) and the spatial extent of the event (by summing up the local severity of all gauges that are affected by a flood larger than the 10 year event).
The proposed indicator is similar to the flood severity indicator developed by Uhlemann et al (2010), however, it uses a higher threshold (10 year flood instead of 2 year flood) and does not weigh the severity with the length of the flood-affected river reach. We use a higher threshold, as the indicator of Uhlemann et al (2010) tends to label large-scale events with relatively small return periods as more severe than spatially confined events with very high return periods; the overall severity of floods thus tends to disagree with their impacts. Further, we refrain from weighting as our set of streamflow gauges is rather homogenous across Germany and we can thus assume that each gauge has a similar weight.
4. Results and discussion
4.1. Counterfactuals of the disasters 1993, 2002 and 2021
Here, we present the results for three important disasters in detail. The overall patterns of flooding are well represented by our model (figure 2, second row): the 1993 flood affected mainly the middle and lower parts of the Rhine basin, while the 2002 flood caused heavy damage in the Elbe and Danube catchments. The 2021 flood was exceptional as it occurred mostly as flash flooding in small rivers. Although our model works with a comparatively coarse space-time resolution, the overall spatial pattern of the event is well represented.
Figure 2. Comparison of three important flood disasters (1993, 2002, 2021) with their spatial counterfactuals. Subplots A1-C1: Event severity SI for the observed floods (filled red, centre) and their 24 spatial counterfactuals. Circle size represents severity. The no-shift event has the same size for each event; thus, circle size can only be compared for an event and its counterfactuals. Yellow (blue) represents counterfactuals that are more (less) severe than the observed flood (also presented by unfilled red circles for reference). Subplots A2-C4: maps of Germany with 516 streamflow gauges where each gauge is coloured according to the return period of the event shown. A2-C2: observed, i.e. no-shift events. A3-C4: selected counterfactuals.
Download figure:
Standard image High-resolution imageShifting the event precipitation creates upward and downward counterfactuals, i.e. less severe and more severe situations (figures 2(A1)–(C1)). Whether, and to which extent, the spatial shift aggravates the flood situation depends on the distance and direction of the shift. The larger the shifting distance, the larger the change in flood severity. The influence of direction is explained by the boundary effect. All three disasters occurred in regions close to the German border. Shifting the precipitation towards the center of Germany tends to increase the severity, as more gauges within Germany are flood-affected. For instance, the 2021 flood hit the border region of The Netherlands, Luxembourg, Germany and Belgium. In Germany, mostly rivers left to the Rhine were heavily affected (figure 2(C2)). Shifting it towards the border relaxes the flood situation for Germany; however, it aggravates the situation for the respective neighbouring countries. This effect, however, cannot be quantified as our hydrological model does not include the river network in the neighboring countries.
Shifting precipitation can create much more severe flooding than the historical realization. For instance, if the storm track that caused the 1993 Christmas flood had occurred 100 km towards northeast (figure 2(A4)), then the flooding would have been 35% more severe (when measured by SI.) The gauges that showed strong flooding (streamflow larger than the 50 year flood peak) in the historical realization would be similarly affected in this scenario—in addition, many other gauges with observed flood peaks smaller than the 50 year event would also show strong flooding.
Spatial counterfactuals can also create heavy flooding in regions that have been spared from havoc by the observed event. For instance, shifting the precipitation by 100 km towards northeast for the 1993 Christmas flood leads to flood peaks with return periods of up to 1 000 years in the Weser river basin (figure 3(A4)), which was only mildly affected in reality.
Figure 3. Comparison of the severity of the observed floods and their spatial counterfactuals for the ten most disastrous floods in Germany. The horizontal axis represents the SI-ratio, defined as the ratio between the shifted and observed, non-shifted, event. A counterfactual flood with a ratio value of 1 is thus equally severe compared to the factual flood at the scale of Germany. The density function represents the severity of the 24 counterfactuals. The density plot assumes that each counterfactual has the same probability of occurrence; an assumption which may not be valid.
Download figure:
Standard image High-resolution image4.2. Severity of counterfactuals for all selected flood disasters
Next, we compare the severity of all selected disasters with their spatial counterfactuals in an aggregated way. To this end, we plot the SI ratio (figure 3), defined as the ratio of the severity index between the shifted and observed, no-shift, events. Shifting the event precipitation can transform a disaster into a non-flood situation with SI ratios of zero; i.e. there is no gauge that shows a peak larger than the 10 year flood. Shifting also generates much more severe situations. For instance, we obtain floods up to three times more severe for the January 1995 event. The most disastrous flood in German history, the 2021 flood, is aggravated by up to 2.4 times by shifting the event rainfall.
The sensitivity of flood severity to shifts in event precipitation varies strongly across the event set. The most sensitive event is the 1993 flood. Shifting the precipitation can reduce the disaster to a non-flood situation or increase its severity by 300%. The least sensitive event is the 2013 flood. Most of the spatial counterfactuals of this event show SI ratios between ±20%.
The different sensitivity is a consequence of boundary effects; shifting a precipitation event, that occurred close to the border, inwards (outwards) may strongly increase (decrease) the flood severity for Germany. Another factor influencing the sensitivity is the spatial variation of the antecedent state and the event precipitation. For instance, the small sensitivity of the 2013 flood is partially explained by the rather homogeneous antecedent state. In the weeks prior to the event, exceptional rain led to wet soils across large parts of Germany (Schröter et al 2015). The situation is different when the antecedent state is spatially heterogeneous; in that case, shifting the event precipitation may strongly affect the flood severity depending on whether it is shifted from a wet area to a dry area or vice versa.
4.3. Unprecedented floods
Because unprecedented floods tend to overwhelm disaster management, we analyze to which extent the spatial counterfactuals lead to flood peaks higher than the flood-of-record. To this end, we first select four gauges from the major river basins and plot the counterfactual flood peaks along with the flood frequency curves (figure 4). In two out of four cases cases, we observe counterfactuals that are substantially larger than the flood-of-record, thus qualifying as unprecedeted flood. For the gauge Oberthau/Weiße Elster, we obtain a flood peak 35% larger than the flood-of-record. While the flood-of-record has a return period of 55 years, the largest counterfactual represents a 200 year event.
Figure 4. Flood frequency curves and selected flood disasters for four gauges (location see figure 1) in the major river basins Elbe (Oberthau/Weiße Elster), Danube (Landsberg/Lech), Rhine (Hoffnungsthal/Sülz) and Weser (Intschede/Weser). The frequency curves are derived by fitting the GEV distribution to the annual maximum flow. Coloured circles show the counterfactual peak flows for selected disasters. Red stars indicate the flood-of-record. Black stars show the flood peak for the no-shift situation.
Download figure:
Standard image High-resolution imageFigure 5 shows how the spatial shifting of event precipitation generates unprecedented floods. At 369 gauges (72% of all gauges) we find at least one counterfactual flood that has a peak higher than the flood-of-record. At several gauges, particularly in the west of Germany, there are around 30 instances where counterfactuals exceed the flood-of-record. Obtaining unprecedented floods in 72% of all gauges demonstrates that spatial counterfactuals are an adequate means to develop exceptional floods, given that the ten observed disasters led to flood-of-records in only 24% of gauges (figure 5).
Figure 5. Gauges where spatial counterfactuals exceed the flood-of-record. At 369 gauges (out of 516 gauges in total), there is at least one counterfactual flood that exceeds the observed flood-of-record. Colours code the number of counterfactuals with peaks larger than the flood-of-record. Circles with green boundary indicate gauges, where one out of ten disasters led to the flood-of-record.
Download figure:
Standard image High-resolution imageGiven that spatial counterfactuals produce many unprecedented events, we propose to use this approach to overcome people's reluctance to discuss exceptional floods. It could be a lever to overcome the availability bias, which is often a barrier to thinking about exceptional events, as people can more easily relate to an actual event that is used as starting point of the counterfactuals compared to a more abstract extreme scenario such as a 500 year flood. In addition, it might help to overcome the near-miss effect in risk perception. After a near-miss, i.e. an event with potentially serious adverse outcomes that did not materialize, people tend to feel safe and underestimate the risk (Bogani et al 2023). Demonstrating what could have happened if the storm track had taken a slightly different trajectory, might convince people that they just got lucky and that their feeling of safety is biased. However, people can show a place-based optimism bias and wrongly believe that an extreme event is more likely to hit nearby places instead of their own place (Klockow et al 2014). Hence, the role of spatial counterfactuals in the communication of flood risk and mitigation measures to lay people needs to be addressed in the future.
4.4. Limitations
A potential weakness of our spatial counterfactual approach is a degree of subjectivity. Expert knowledge of the flood-triggering meteorological mechanisms in the given region is needed to exclude spatial shifts that are not plausible. Furthermore, flood generation depends on the interactions between atmospheric and land surface processes. A priori, it is not clear which shifts in terms of direction and distance cause the largest flood. Hence, one might need to simulate a large number of scenarios to identify the most severe response for a given region.
Our flood model used does not include the consequences of flooding in terms of inundation and losses. A counterfactual analysis including inundation areas and losses would be even more informative. Conceptually, it would be straightforward to extend the approach and to propagate the simulated streamflow through a hydrodynamic model providing inundation areas and through a loss model. For Germany, this could be achieved by the continuous simulation approach of the entire flood process chain (Sairam et al 2021). A computationally less demanding approach would be to transfer the point-based peak estimates into inundation areas by sampling and mosaicking the pre-computed inundation maps (Bates et al 2021). Such an extension would open the possibility to investigate counterfactuals that emerge at a later stage in the flood process chain. For instance, one could explore the effects of failing warning systems or evacuation plans on the flood situation.
5. Conclusions
We propose a method to explore exceptional floods which should be easy to communicate and to understand even for lay people. Shifting the event precipitation of a flood, that has actually occurred and that has been experienced by (some) people, is a straightforward way to link the experience of people to exceptional flooding. Applying the idea of spatial counterfactual floods to Germany leads to exceptional floods, some of which are much higher than the floods experienced. For instance, the most expensive disaster in German history, the July flood 2021, could have been twice as severe if the storm path of the low-pressure system Bernd would have been shifted 100 km to the east or northeast due to a change in the atmospheric persistent blocking system. Our counterfactuals generate at more than 70% of the gauges (369 out of 516) peak flows that exceed the current flood-of-record. Given that risk management tends to focus on the largest observed floods, the ease with which many new flood-of-records are generated with our approach is disturbing.
We propose to complement flood maps with spatial counterfactuals. Current maps are based on the concept of the n-year flood, which causes widespread confusion (Pielke 1999). In addition, spatial counterfactuals are developed in terms of events. This makes them easier to comprehend compared to traditional flood maps that show the flooded area for a given return period. This event information is also highly relevant for a range of stakeholders; for instance, for disaster managers who need to understand the spatial and temporal characteristics of possible future events. For them it is important to know how widespread a flood situation can be and how long it may take.
Actual floods open a window of opportunity to communicate the risk and potential mitigation measures. The rapid development of spatial counterfactuals, similar to the rapid provision of attribution statements after extreme weather events (www.worldweatherattribution.org), could utilize this window of opportunity to motivate society to reduce risk.
Data availability statement
The data that support the findings of this study are openly available at the following URL/DOI: https://doi.org/10.5880/GFZ.4.4.2024.002. Data will be available from 01 April 2024.
Funding
This work was funded by the DFG Research Group FOR 2416 'Space-Time Dynamics of Extreme Floods—SPATE', Grant Number 278017089) and by the Federal Ministry of Education and Research of Germany (BMBF ClimXtreme project FLOOD, Grant Number 01LP1903E; and BMBF KAHR project; Grant Number 01LR2102A is gratefully acknowledged.