- 1Department of Reproductive Medicine, Yantai Yuhuangding Hospital Affiliated to Qingdao University, Yantai, Shandong, China
- 2Shandong Provincial Key Medical and Health Laboratory of Reproductive Health and Genetics (Yantai Yuhuangding Hospital), Yantai, Shandong, China
Background: About 10% of individuals undergoing in vitro fertilization encounter recurrent implantation failure (RIF), which represents a worldwide social and economic concern. Nevertheless, the critical genes and genetic mechanisms underlying RIF are largely unknown.
Methods: We first obtained three comprehensive microarray datasets “GSE58144, GSE103465 and GSE111974”. The differentially expressed genes (DEGs) evaluation, enrichment analysis, as well as efficient weighted gene co-expression network analysis (WGCNA), were employed for distinguishing RIF-linked hub genes, which were tested by RT-qPCR in our 30 independent samples. Next, we studied the topography of infiltration of 22 immune cell subpopulations and the association between hub genes and immune cells in RIF using the CIBERSORT algorithm. Finally, a novel ridge plot was utilized to exhibit the potential function of core genes.
Results: The enrichment of GO/KEGG pathways reveals that Herpes simplex virus 1 infection and Salmonella infection may have an important role in RIF. After WGCNA, the intersected genes with the previous DEGs were obtained using both variance and association. Notably, the subsequent nine hub genes were finally selected: ACTL6A, BECN1, SNRPD1, POLR1B, GSK3B, PPP2CA, RBBP7, PLK4, and RFC4, based on the PPI network and three different algorithms, whose expression patterns were also verified by RT-qPCR. With in-depth analysis, we speculated that key genes mentioned above might be involved in the RIF through disturbing endometrial microflora homeostasis, impairing autophagy, and inhibiting the proliferation of endometrium. Furthermore, the current study revealed the aberrant immune infiltration patterns and emphasized that uterine NK cells (uNK) and CD4+ T cells were substantially altered in RIF endometrium. Finally, the ridge plot displayed a clear and crucial association between hub genes and other genes and key pathways.
Conclusion: We first utilized WGCNA to identify the most potential nine hub genes which might be associated with RIF. Meanwhile, this study offers insights into the landscape of immune infiltration status to reveal the underlying immune pathogenesis of RIF. This may be a direction for the next study of RIF etiology. Further studies would be required to investigate the involved mechanisms.
Introduction
Despite the growing application of in vitro fertilization (IVF), success rates have remained relatively constant, and about 10% of patients undergoing IVF treatment experience recurrent implantation failure (RIF), which is a worldwide social and economic concern (Busnelli et al., 2020). The pathophysiologic process of RIF is complicated; thus, there is no consensus guideline for its diagnosis and treatment at present. RIF is frequently defined as failure to achieve a clinical pregnancy after transferring at least four good-quality embryos in at least three fresh or frozen cycles (Coughlan et al., 2014). RIF could be related to embryonic characteristics (male or female origin), embryo transfer, endometrial or immunological factors, or a composite of these. Notably, recent studies highlighted that it has become apparent that endometrial factors have an important role in RIF(Huang et al., 2017; Zhou et al., 2019). Moreover, plenty of literature suggests that endometrial gene expression profiles could be changed in RIF patients (Feng et al., 2018; Ahmadi et al., 2022). Nevertheless, the critical genes and genetic mechanisms underlying RIF are largely unknown.
With the popularity of large-scale gene expression analysis, emerging bioinformatics via public databases can detect differentially expressed genes (DEGs) concurrently at the level of transcription for numerous genes (Koot et al., 2016; Guo et al., 2018; Bastu et al., 2019). However, traditional DEG-based screening methods have the disadvantage of local dataset exploring, so it is quite possible that the master molecules will be missed. Encouragingly, weighted gene co-expression network analysis (WGCNA) can detect co-expression modules and genes in the entire biological system in several samples (Langfelder and Horvath, 2008). By comparable gene expression patterns, genes are grouped to form modules that are studied for their relevance to certain properties, like patient clinical information. Such modules and their main genes can be exploited to find potential biomarkers or therapeutic targets. WGCNA is therefore anticipated to be a novel and potent tool for revealing the RIF potential pathological mechanism.
First, we scanned the microarrays stored in the GEO (Gene Expression Omnibus) database for obtaining genes with differential expression between RIF and healthy fertile controls. Second, functional enrichment analysis was performed to determine potential biological roles and signaling pathways implicated in RIF. Next, functional modules associated with clinical characteristics were scanned employing powerful WGCNA. RIF-associated hub genes were detected and constructed a network of protein-protein interactions (PPI). Concurrently, bioinformatics chip analysis results were validated using quantitative real-time polymerase chain reaction (qRT-PCR). Next, we studied the topography of infiltration of 22 immune cell subpopulations and the association between hub genes and immune cells in RIF using the CIBERSORT algorithm. Finally, diagnostic value, and functional enrichment analyses by Gene Set Enrichment Analysis (GSEA) were sufficiently performed in succession. Thus, our objective was to investigate RIF potential hub genes and molecular mechanism(s), which will expand our understanding of the molecular association between immune infiltration and RIF and propose promising treatment options for RIF patients.
Materials and methods
Microarray datasets
Figure 1 displays this research flowchart. The comprehensive profiles of mRNA expression of GSE58144 (Koot et al., 2016), GSE103465 (Guo et al., 2018) and GSE111974 (Bastu et al., 2019) were collected from the GEO (Gene Expression Omnibus) repository (https://www.ncbi.nlm.nih.gov/geo/). All three gene chip profiles were obtained from human mid-luteal phase endometrial biopsies. Dataset GSE58144 was performed according to the platform GPL15789 (A-UMCU-HS44K-2.0), which includes 43 repeated implantation failure (RIF) samples and 72 healthy controls. Series GSE103465 was carried out by GPL16043 (GeneChip® PrimeView™ Human Gene Expression Array) and included three RIF samples and three normal control samples. GSE111974, according to the GPL17077 platform (Agilent-039494 SurePrint G3 Human GE v2 8x60K Microarray 039381), included 24 RIF as well as 24 healthy fertile control samples. Supplementary Table S1 provides detailed information about these three datasets.
Data pre-processing and DEG screening
The raw expression matrix of three datasets was first performed quantile normalization to ensure the columns have the same distribution utilizing the “preprocessCore” package in R tool program (R Foundation for Statistical Computing version 4.1.2). We performed log2-transformation and background correction on GSE58144, GSE103465, and GSE111974 expression profiles utilizing the R package “linear models for microarray data” (limma). Using the annotation file as a basis, probe IDs were converted to gene symbols. For numerous probes mapping to a single gene, the mean expression value of each probe was utilized. The adjusted p-value was utilized to regulate the false discovery rate induced by repeated testing. The “limma” program screened DEGs between RIF and fertile control samples. Different datasets have different situations, so it is not feasible to use |log2 fold-change (FC)| > 1 uniformly, which will miss many useful genes. As we use three datasets, we use adjusted p-value < 0.05 and |log2 fold-change (FC)|>0 to filter genes and then take the intersection. Moreover, the DEGs were shown as a volcano plot and heatmap using the R packages “ggplot2” as well as “pheatmap.”
Functional enrichment analysis
Further, DEGs functional enrichment analysis was conducted, which were upregulated or downregulated in at least two datasets by the “VennDiagram” and “RColorBrewer” packages in R. The Gene Ontology-Biological Process (GO-BP) and Kyoto Encyclopedia of Genes and Genomes (KEGG) pathways were then investigated using the “clusterProfiler” and “enrichplot” packages to find potential biological roles.
WGCNA to identify RIF-related key gene modules
Weighted gene co-expression network analysis (WGCNA) is an algorithm for constructing a co-expression network, which reveals the correlation patterns across genes and provides the biologically functional interpretations of network modules (Yuan et al., 2020). As mentioned before, the intersections of gene lists in GSE58144 were selected to build a co-expression network employing “WGCNA” package (version 1.60). After assessing the existence of apparent outliers through cluster analysis, the one-step network building tool was utilized for co-expression network construction as well as the detection of main modules. Furthermore, to determine the relevance of every module, the module eigengene (ME) was summed according to the initial major element of module expression, and based on the relationship between MEs and clinical features, module-trait associations were evaluated. Then, the correlation strength was assessed using module significance (MS), which refers to the mean absolute gene significance (GS) of each gene inside a module. Relevantly, the GS value was derived by log10-transforming the p-value from the linear regression between expression and clinical characteristics. Generally, the modules with the highest MS values were deemed to be the key modules.
Enrichment analysis of genes in key modules
Using the “clusterprofiler” and “enrichplot” packages, we executed KEGG pathway enrichment analysis to comprehend the biological significance of genes in key modules (Top 20). Moreover, to illustrate the gene-corresponding relationship between the terms, a sub-cluster of terms (the Top 5 in KEGG) was chosen and presented as a network plot (with a similarity of >0.3). Every node depicted a term that has been enriched and colored based on its cluster-ID.
PPI network construction and hub genes identification
In WGCNA, key genes were determined from key modules with |module membership (MM)|≥ 0.8 and |GS|≥ 0.2. Following overlapping the DEGs and key genes from WGCNA, we inserted these genes into the STRING (http://string-db.org) database to gather target proteins interactions with a medium confidence score of >0.4 [12] and built a PPI network via Cytoscape program (v3.7.2).
Moreover, Hub genes were determined as the central genes for our study employing the Cytoscape plug-in program “cytoHubba” depending on “Closeness,” “Radiality,” as well as “Stress” character calculation.
Diagnostic effectiveness of hub genes
The functional correlation analysis was conducted among the above hub genes from GSE58144. Moreover, the differentially expressed hub genes were shown regarding adjusted p-value and logFC by the heatmap from GSE58144, GSE103465, and GSE111974.
Notably, ROC analysis was conducted to anticipate the biomarkers diagnostic effectiveness. The area under the ROC curve (AUC) value was used to measure the diagnostic effectiveness of GSE58144 dataset in distinguishing RIF from control samples.
Immune infiltration analysis
The “CIBERSORT” tool in R from GSE58144 was employed to assess the level of immune infiltration in RIF. We investigated the connection between hub genes and immune cell infiltration utilizing correlation analysis as well as the “ggplot2” tool in R.
Functional correlation and enrichment analyses by GSEA against the Reactome
Applying GSE58144, a functional correlation study was conducted between hub genes and other mRNAs in RIF, and the Pearson correlation coefficient was determined. The top 50 most positively correlated genes with hub genes were chosen for enrichment analysis to indicate the possible function of hub genes. GSEA was conducted on the Reactome pathways utilizing “gsePathway” R tool of the “clusterProfiler.”
qRT-PCR verification
15 RIF women and 15 healthy fertile controls were recruited between October 2020 and June 2021 in the Yantai Yuhuangding Hospital, a Qingdao University Affiliate. Their mid-luteal phase endometrial tissues were collected to snap-frozen and stored at −80°C until use in the qRT-PCR experiments. Briefly, Trizol (TaKaRa, Dalian, China) was used to isolate total RNA from tissues above the endometrium. The RNA purity was evaluated utilizing a NanoDrop 2000 (Thermo Scientific-USA). cDNA synthesis was performed using the HiScript III RT SuperMix (Vazyme Biotech Co., Ltd., Nangjing, China). The qRT-PCR was conducted with ChamQ universal SYBR qPCR master mix (Vazyme Biotech Co., Ltd., Nangjing, China). Supplementary Table S2 displays the primers (Sangon Biotechnology Company, Shanghai, China) utilized in this work. The 2−ΔΔCt method assessed the relative gene expressions using GAPDH as the reference gene. The complete experimental process was performed for each sample in triplicate. All patients provided informed permission in writing. Ethical approval for the study was granted by the ethical committee of Yantai Yuhuangding Hospital (20221208).
Statistical analysis
The RT-qPCR results were analyzed by using SPSS (version 24.0, Chicago, IL) and GraphPad Prism (version 8, San Diego, CA) software. Continuous variables were expressed as mean ± standard deviation (SD), and differences between the two groups were compared using Student’s t-test for normally distributed variables. Nonparametric tests were applied for non-normal distribution data. The difference was deemed statistically significant if the p-value < 0.05. All microarray data analysis and visualization in this work were conducted with R software (version 4.1.2; https://www.r-project.org/) with the proper packages which are listed in detail in “Materials and methods” individually. All the related raw data and R code are provided in the “Supplementary Material”.
Results
DEGs identification in patients with RIF and fertile controls
Three raw microarray datasets, including a total of 99 RIF and 70 normal endometrial tissues, were chosen for the research. Figure 2 displays the data before (A, C, E) and after (B, D, F) quantile normalization, indicating that the normalizing of raw datasets was successful. Following performing data pre-processing on the GSE58144, GSE103465, and GSE111974 datasets, we screened DEGs using the cutoff criteria of adjusted p-value <0.05 and |log2 FC| ≥ 0. In GSE58144, we detected 704 upregulated and 1085 downregulated genes. The top 21 DEGs are shown in Figures 3A, B by the volcanic diagram and the expression heatmap. Similarly, a sum of 4807 overexpressed genes and 5174 downregulated genes were identified in GSE111974 (Figures 3C, D). In GSE103465, 690 genes were overexpressed, whereas 740 were downregulated (Figures 3E, F).
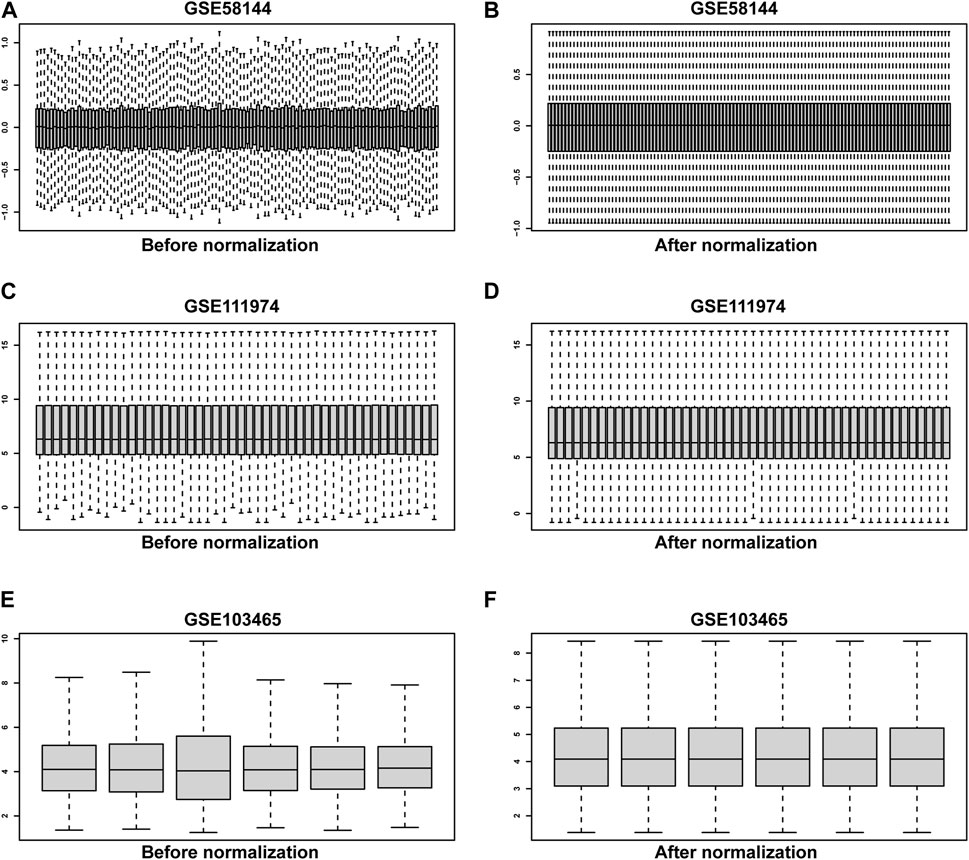
Figure 2. The quantile normalization. Before normalization for GSE58144, GSE111974 and GSE103465 datasets (A, C, E), respectively. After normalization for GSE58144, GSE111974 and GSE103465 datasets (B, D, F), respectively.
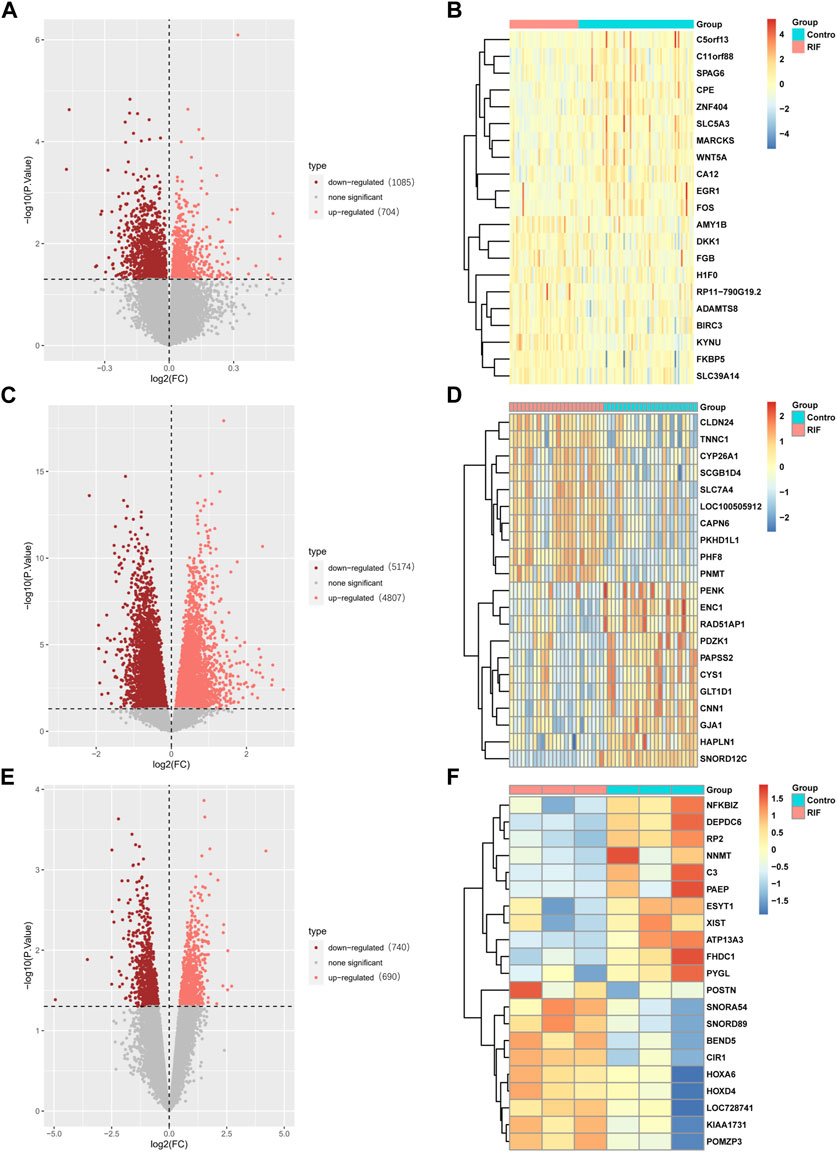
Figure 3. Detection of differentially expressed genes (DEGs) in the GSE58144, GSE111974 and GSE103465 datasets. The expression volcano plots in the GSE58144 (A), GSE111974 (C) and GSE103465 (E) datasets, respectively. The heatmap of the top 21 DEGs corresponding to the GSE58144 (B), GSE111974 (D) and GSE103465 (F) datasets.
Finally, 8 upregulated DEGs (Figure 4A) and 20 downregulated DEGs (Figure 4B) were shared among the three datasets, which were displayed through Venn diagram analyses (Figure 4).
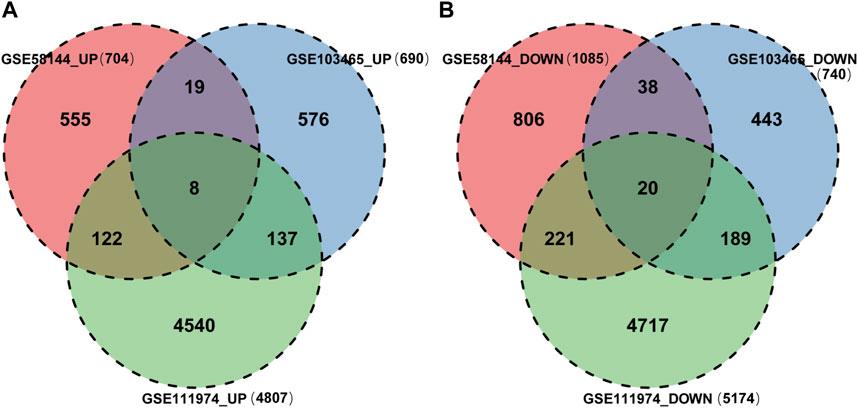
Figure 4. Identification of shared DEGs. (A) DEGs upregulated among the GSE58144, GSE111974 and GSE103465 datasets. (B) DEGs downregulated among the GSE58144, GSE111974 and GSE103465 datasets.
Pathway enrichment analyses
Functional enrichment on the above DEG, which was upregulated or downregulated in at least two datasets, was conducted to discover potential biological roles. GO-BP analysis indicated that RIF was mostly associated with negative control of transport and regulation of mitotic cell cycle phase transition, intracellular transport regulation, post−translational protein modification, nucleocytoplasmic transport, and nuclear transport (Figure 5A). Such DEGs were also enriched in KEGG pathways, including Herpes simplex virus 1 infection, Amyotrophic lateral sclerosis, Huntington’s disease, Salmonella infection, Hippo signaling pathway, and Tight junction, which seemed to be associated with RIF (Figure 5B). Supplementary Table S3 demonstrated a list of detailed genes from the datasets that contribute to the identified pathways.
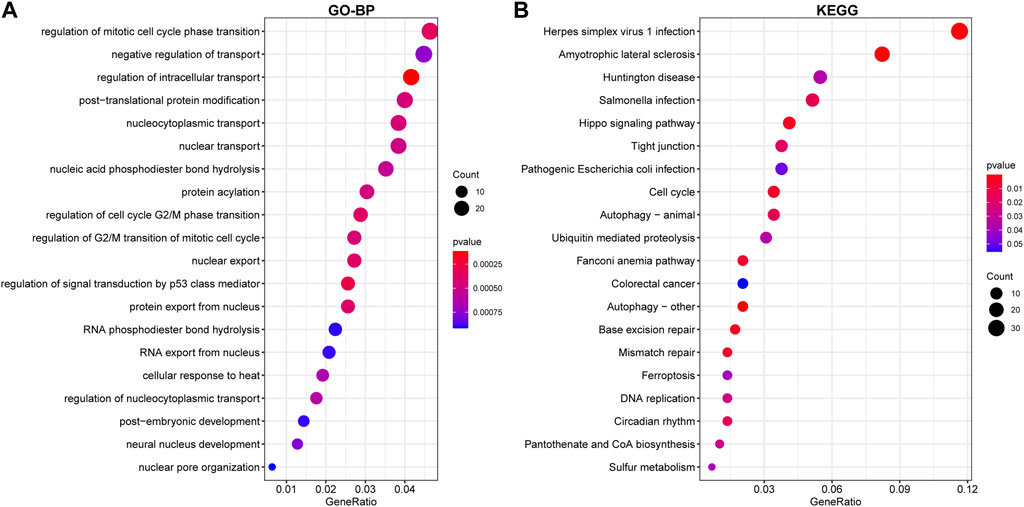
Figure 5. Enrichment of DEGs using Gene Ontology (GO)-BP (A) and Kyoto Encyclopedia of Genes and Genomes (KEGG) (B) analysis. The larger the circle in the figure, the more genes it contains; lower p values are indicated with a stronger red color.
WGCNA and key modules identification
To study the relation between the aforementioned DEGs and RIF clinical characteristics, we performed a molecular clustering analysis on the GSE58144 dataset using the WGCNA algorithm. On the basis of a dynamic hybrid cut and a scale-free network with topological overlaps, a hierarchical clustering tree was constructed (Figure 6A). The GSE58144 series comprised seven kinds of clinical characteristics: control, RIF, previous implantation, smoking, embryo implantations, age, and BMI. Based on an initial assessment of the data, we determined that a power value of 8 (scale-free R2 = 0.82) was an adequate soft threshold for continued investigation (Figure 6B). As a consequence, we acquired four connected modules colored differently: blue (191 genes), brown (405 genes), green (81), and grey (59) (Figure 6C).
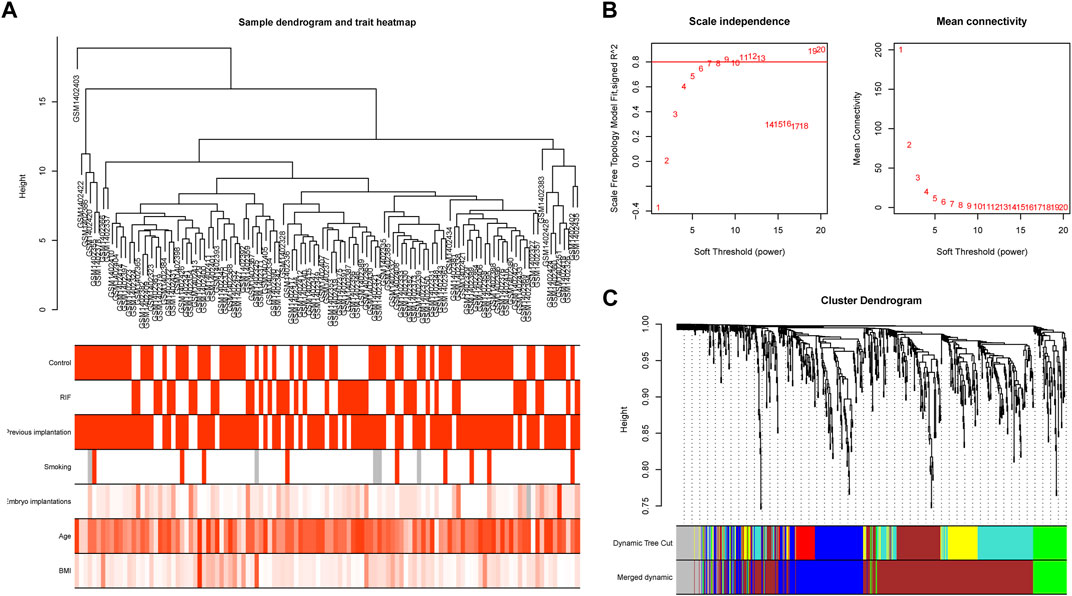
Figure 6. Sample clustering and network construction of the weighted gene co-expression network analysis. (A) Clustering dendrogram of 43 RIF and 72 control samples. The color intensity was proportional to disease status (control or RIF) or clinical traits (previous implantation, smoking, embryo implantations, age and BMI). (B) Analysis of the scale-free fit index and the mean connectivity for various soft-thresholding powers. The soft-thresholding power of 8 was selected based on the scale-free topology criterion. (C) Dendrogram clustered based on a dissimilarity measure (1-TOM). Gene expression similarity is assessed by a pair-wise weighted correlation metric and clustered based on a topological overlap metric into modules. Each color below represents one co-expression module, and every branch stands for one gene.
We investigated the link between the aforementioned four modules as well as clinical parameters to assess the clinical significance of modules. Figure 6 displays that none of the modules had a statistically significant association with prior implantation, smoking, embryo implantations, age, or BMI (p > 0.01). In contrast, the brown and grey modules had a high positive association with the control group, but both modules demonstrated a significant negative correlation with RIF (Figure 7). Therefore, subsequent analyses were carried out on genes from the brown and grey modules.
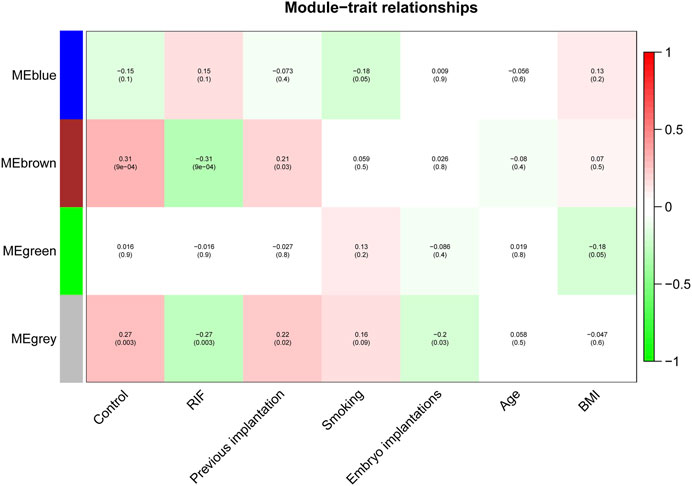
Figure 7. The identification of key modules via weighted gene co-expression network analysis. Heatmap of the correlation between module eigengenes and the clinical traits. The corresponding correlation coefficient along with p-value is given in each cell, and each cell is color-coded by correlation according to the color (legend at right).
Enrichment analysis of key modules
We utilized KEGG analysis for a better understanding of the potential biological role of two key modules related to RIF. Figure 8A displays that KEGG pathway analysis (top 20) indicates that Herpes simplex virus 1 infection and Amyotrophic lateral sclerosis were the highest enriched pathways, followed by Huntington’s disease, Hippo signaling pathway, nucleocytoplasmic transport, as well as Fanconi anemia pathway.
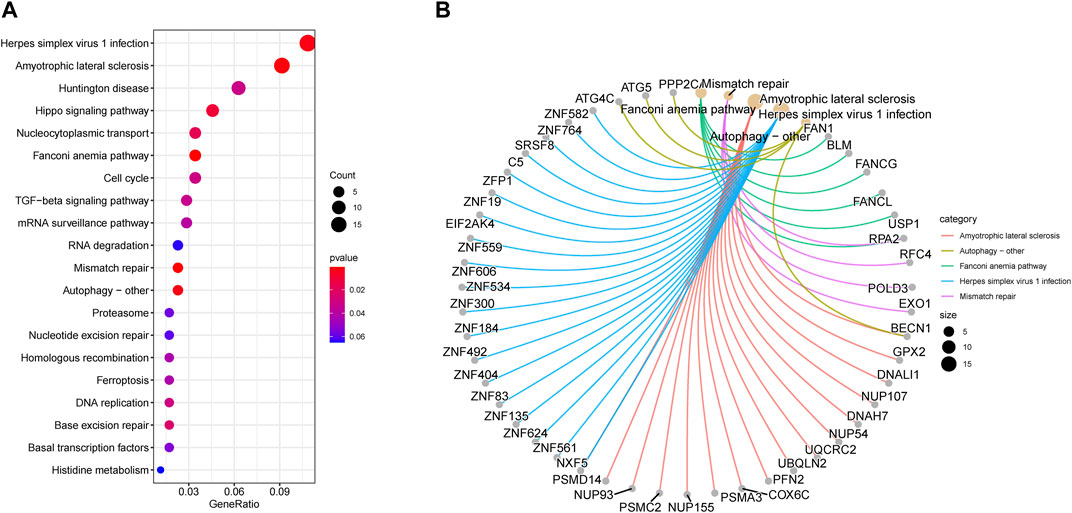
Figure 8. Enrichment analysis of key modules. (A) Kyoto Encyclopedia of Genes and Genomes pathway enrichment analysis in brown and grey modules (top 20). The significance of enrichment gradually increases from blue to red, and the size of the dots indicates the number of genes contained in the corresponding pathway. (B) Gene-Concept Network: Gene corresponding relationship analysis on the top 5 pathways.
Besides that, we executed gene corresponding relationship analysis between the genes and top 5 pathways (Amyotrophic lateral sclerosis, autophagy–other, Fanconi anemia pathway, Herpes simplex virus 1 infection, and Mismatch repair) enriched by KEGG in key modules (Figure 8B).
PPI network Construction and hub gene identification in the key modules
To study in further depth the interaction between genes in the aforementioned brown and grey modules, a PPI network was formed using the STRING database tool, and 464 nodes were displayed using the Cytoscape program (Figure 9A). Further, we used the Cytoscape plug-in “cytoHubba” to screen the hub genes based on their degree of connectivity scores using three algorithms, “Closeness,” “Radiality,” and “Stress” (Figures 9B–D), respectively. After overlapping the above three algorithms by integrating an intersection of genes (top 12), a sum of 9 hub genes were screened out (Figure 10A), which include: actin like 6A (ACTL6A), beclin1 (BECN1), small nuclear ribonucleoprotein D1 polypeptide (SNRPD1), RNA polymerase I subunit B (POLR1B), glycogen synthase kinase 3 beta (GSK3B), protein phosphatase 2 catalytic subunit alpha (PPP2CA), RB binding protein 7 (RBBP7), polo-like kinase 4 (PLK4) and replication factor C subunit 4 (RFC4). We showed the detailed results of all the above hub genes in Table 1.
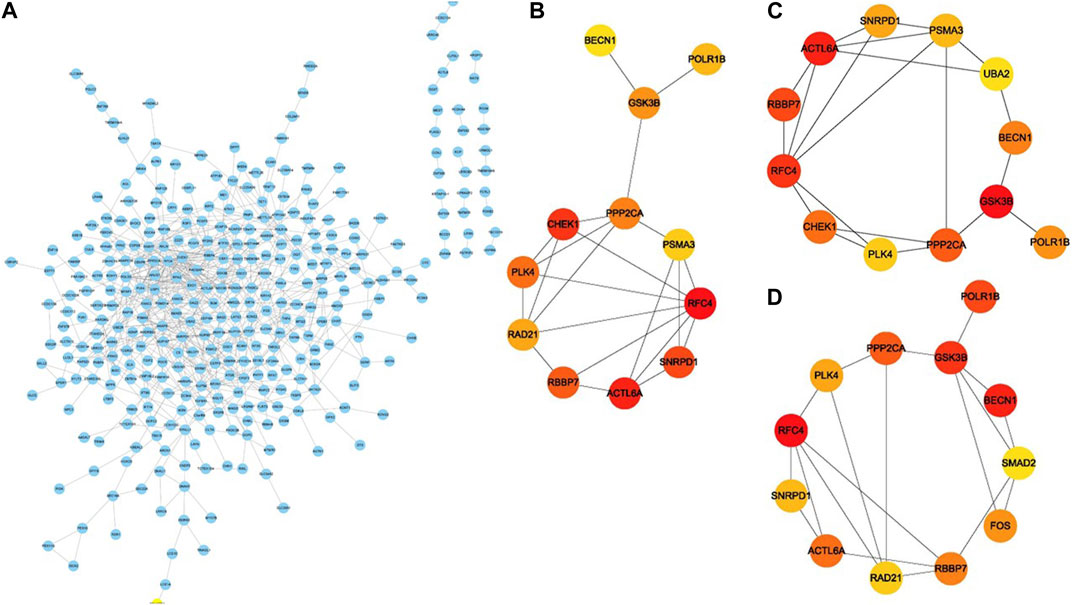
Figure 9. PPI network construction and identification of hub genes. (A) The protein–protein interaction network of the overlapped genes. Key genes identified by “cytoHubba” according to three algorithms “Closeness,” “Radiality” and “Stress” (B–D), respectively. The significance of key genes (top 12) gradually increases from yellow to red.
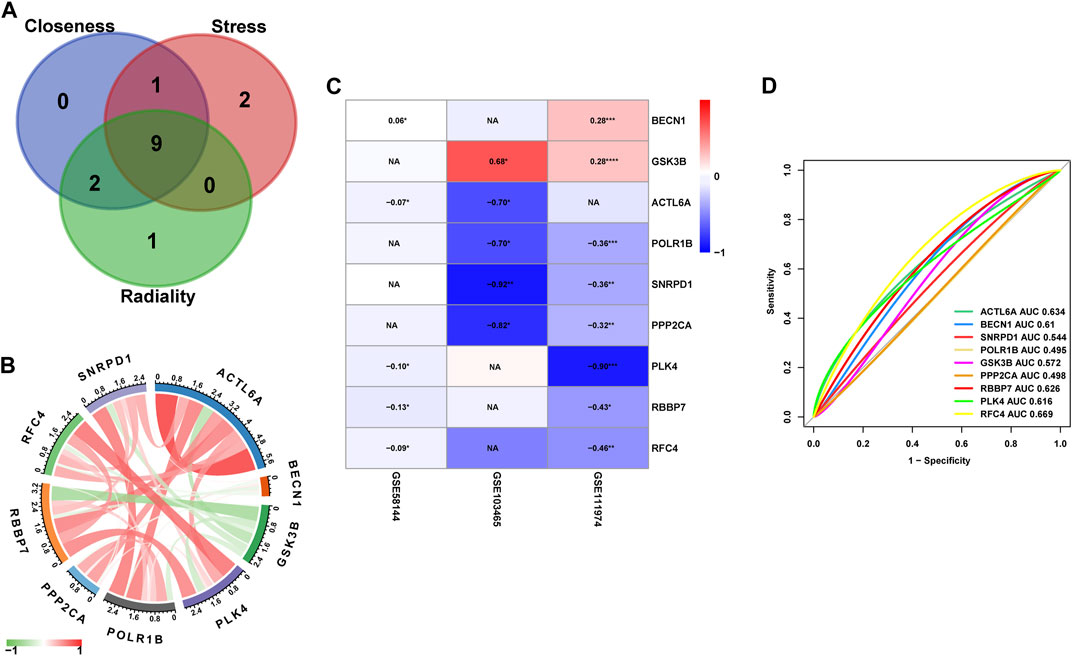
Figure 10. (A) The Venn diagram of Hub genes based on three-character calculations from the key modules. (B) Correlation analysis between the nine core target genes from GSE58144, where red represents positive correlation, green represents negative correlation, the darker the color, the higher the correlation. (C) Heatmap of the nine Hub genes that are differentially expressed in the GSE58144, GSE103465 and GSE111974 datasets. (D) The GSE58144 dataset was used to validate the diagnostic effectiveness of the Hub genes for RIF by ROC analysis.
Correlation and expression of hub genes
Correlation analysis of the nine core genes in GSE58144 indicated that: ACTL6A expression is positively correlated with SNRPD1, POLR1B, PPP2CA, RBBP7, PLK4, and RFC4, and negatively correlated with GSK3B. Notably, the gene expression of GSK3B exhibited a significant negative correlation to all other genes (Figure 10B).
Moreover, the expression levels of ACTL6A, BECN1, SNRPD1, POLR1B, GSK3B, PPP2CA, RBBP7, PLK4, and RFC4 were presented in the heatmap from all three datasets (Figure 10C). Specifically, GSK3B and BECN1 had significantly enhanced expression in RIF in dataset GSE111974 (p < 0.05). However, the levels of expression of POLR1B, SNRPD1, PPP2CA, PLK4, RBBP7, and RFC4 were substantially lower in RIF (p < 0.05). Compatible with the above findings, the expression of GSK3B was also markedly elevated in RIF versus control in dataset GSE103465, but SNRPD1 and PPP2CA had lower expression in RIF (p < 0.05). BECN1 exhibited higher gene expression in GSE58144 (p < 0.05), while ACTL6A, PLK4, RBBP7, and RFC4 displayed lower expression levels in the RIF group.
We further confirmed the expression of nine hub genes via qRT-PCR in our mid-luteal phase endometrial samples. The results were in accordance with the above-described heatmap, i.e., BECN1 and GSK3B were overexpressed in RIF compared to normal endometrial tissues. Meanwhile, ACTL6A, POLR1B, SNRPD1, PPP2CA, PLK4, RBBP7, and RFC4 were significantly lower expressed (Figure 11).
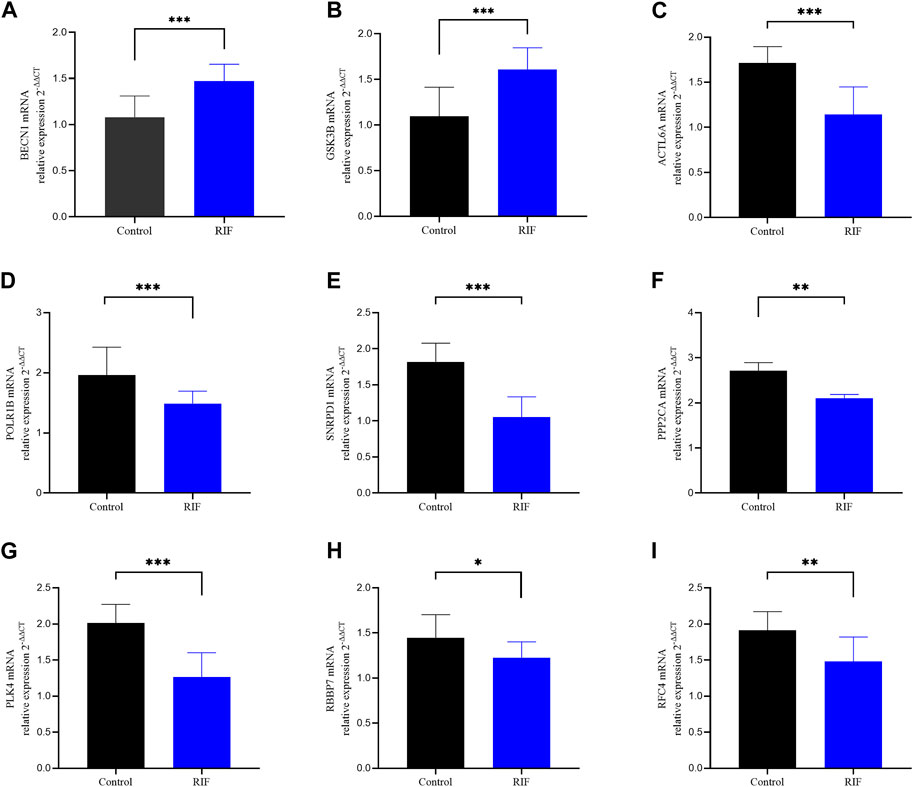
Figure 11. qRT-PCR analysis of the 9 hub genes expression in the indicated groups. Validation of the expression of these 9 hub genes in our study sample. (A) BECN1, (B) GSK3B, (C) ACTL6A, (D) POLR1B, (E) SNRPD1, (F) PPP2CA (G), PLK4 (H) RBBP7, (I) RFC4.
Diagnostic value of hub genes
ROC analysis was employed for the validation of diagnostic effectiveness of the hub genes for RIF using the GSE58144 dataset. Figure 10D depicts the AUC values for RFC4, ACTL6A, and RBBP7 were 0.669 (95% CI 0.564–0.763), 0.634 (95% CI 0.529–0.733), and 0.626 (95% CI 0.518–0.729), respectively. Consequently, it appears that these key genes from the PPI subgroups do not perform well in the diagnosis of RIF (Figure 10D).
Infiltration of immune cells in RIF
Using “cibersoft” package in R, we identified the infiltration landscape of 22 immune cell subpopulations in RIF by evaluating the GSE58144 dataset (72 RIF VS 43 Control). Figure 12A presents that every sample composed of 22 types of immune cells was presented in a histogram. In the histogram, the color indicates the proportion of various immune cells in all samples, and the sum equals 1. Results indicated that T cells CD4 memory resting, T cells CD4 memory activated, NK cells stimulated, macrophages M1, macrophages M2, dendritic cells stimulated, and mast cells resting were the predominant infiltrating immune cells, suggesting that memory CD4 T cells and NK cells were the two most abundant immune cells in the RIF group.
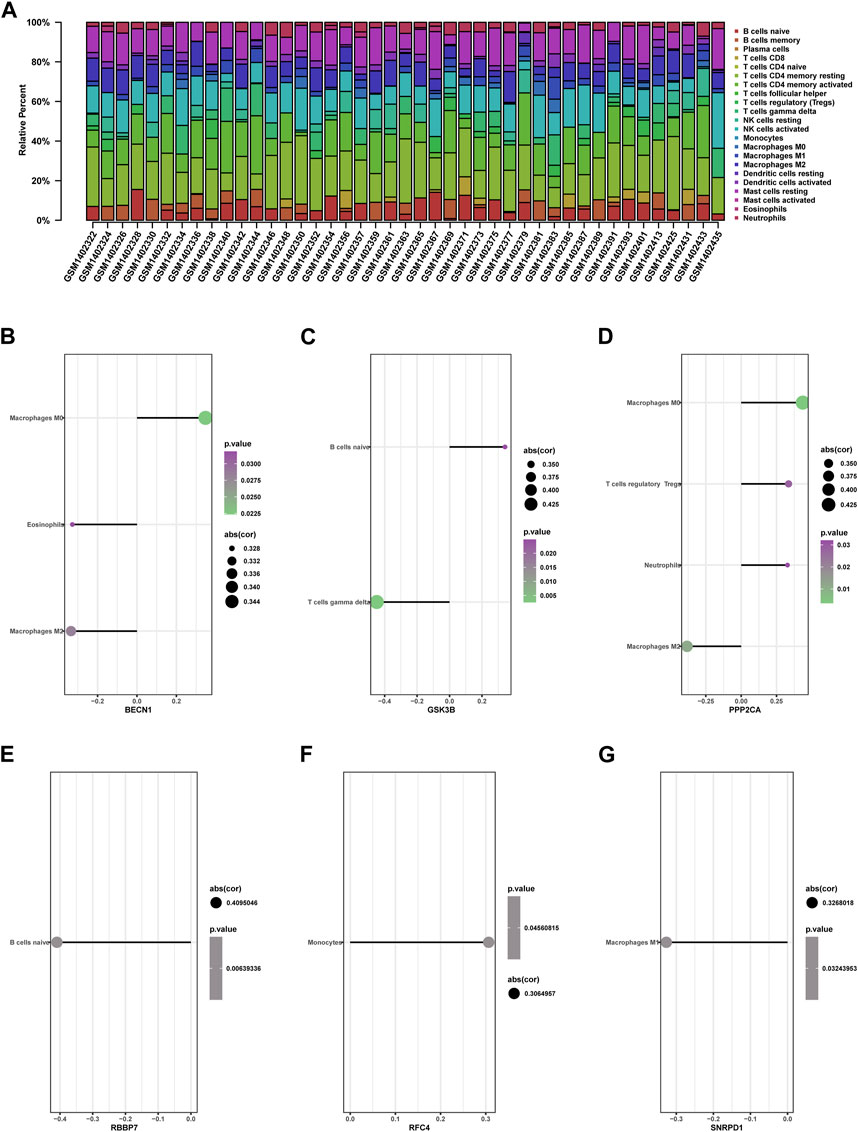
Figure 12. Immune cell infiltration in RIF and Control tissues. (A) The composition of 22 kinds of immune cells in each sample was showed in a histogram. (B–G) Correlation of the expression of 9 hub genes with the infiltration of immune cells from GSE58144.
Hub genes Association with differential immune cells
We further investigated the relationship between nine hub genes and immune cell infiltration. Correlation analysis results are presented in the following (Figure 12).
BECN1 showed positive correlation with macrophages M0 (cor = 0.347636451, p = 0.022), but adversely with eosinophils (cor = −0.327840586, p = 0.032) and macrophages M2 (cor = −0.334793114, p = 0.028) (Figure 12B). GSK3B was positively associated with B cells naive (cor = 0.341707789, p = 0.025) and negatively associated with gamma delta T cells (cor = −0.448673168, p = 0.003) (Figure 12C). PPP2CA presented a positive correlation with macrophages M0 (cor = 0.435657589, p = 0.003) and T cells regulatory Tregs (cor = 0.334876257, p = 0.028) but adversely with macrophages M2 (cor = −0.382965871, p = 0.011) (Figure 12D). RBBP7 presented a negative association with B cells naive (cor = −0.409504551, p = 0.006) (Figure 12E). RFC4 presented a positive association with monocytes (cor = 0.306495744, p = 0.046) (Figure 12F). SNRPD1 was adversely associated with macrophage M1 (cor = −0.326801828, p = 0.032) (Figure 12G).
The function of nine hub genes in RIF
To further elucidate the nine hub genes function, correlation analysis between hub genes and all other genes was performed in RIF using the GSE58144 database. As shown in the heatmap (Figures 13A–I), the top 50 most positively associated genes with ACTL6A, BECN1, GSK3B, PLK4, POLR1B, PPP2CA, RBBP7, RFC4, and SNRPD1, respectively, were selected for subsequent enrichment analysis.
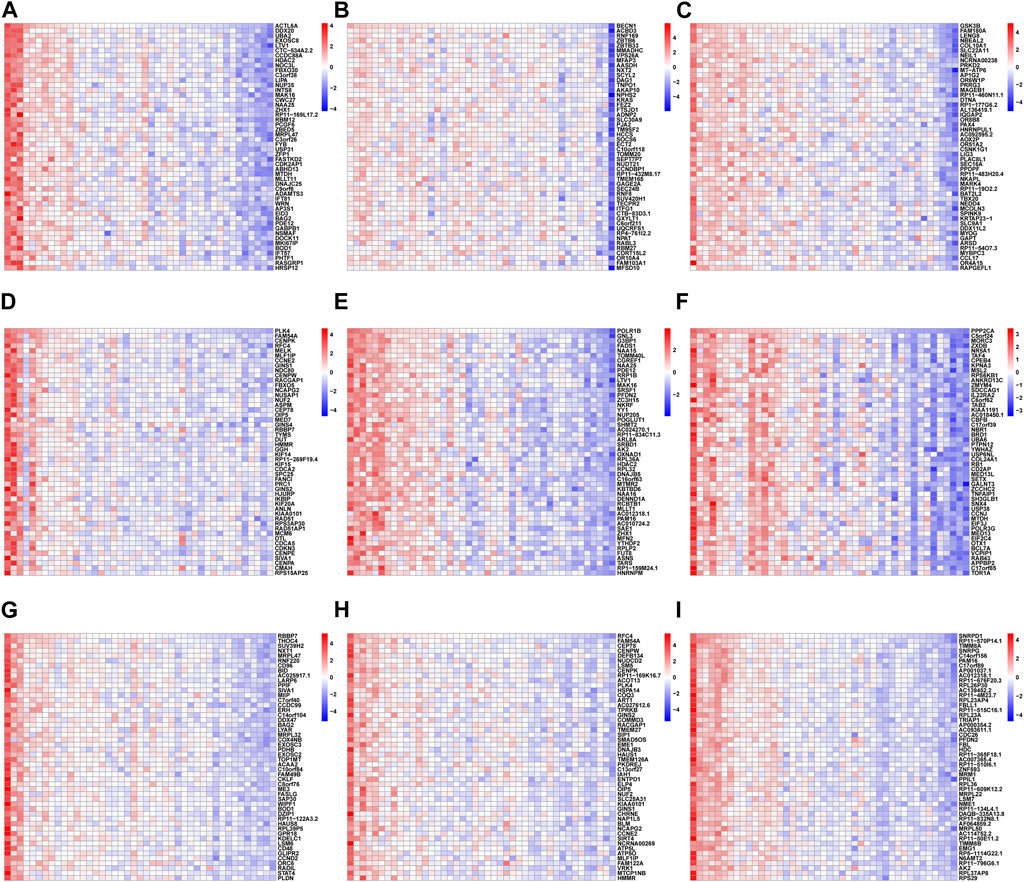
Figure 13. The heatmap of the correlation analysis between the 9 hub genes and other all genes in RIF tissues from GSE58144, where red represents positive correlation, blue represents negative correlation; the deeper the color, the stronger the correlation. Each column of the heatmap represents one sample and each row represents one gene. The heatmaps showed the top 50 most positively associated significant genes with (A) ACTL6A, (B) BECN1, (C) GSK3B, (D) PLK4, (E) POLR1B, (F) PPP2CA, (G) RBBP7, (H) RFC4 and (I) SNRPD1, respectively.
In accordance with the above correlation analysis findings, the functional pathways enrichment analysis of nine hub genes was conducted using GSEA against the Reactome database. Ridge plot (Figures 14A–I) indicated that the processing of rRNA in the nucleus and cytosol, Influenza Viral RNA transcription and replication, and processing of rRNA were significantly enriched in ACTL6A. The situation of other genes can be easily identified in the ridge plot, which is not shown in detail here.
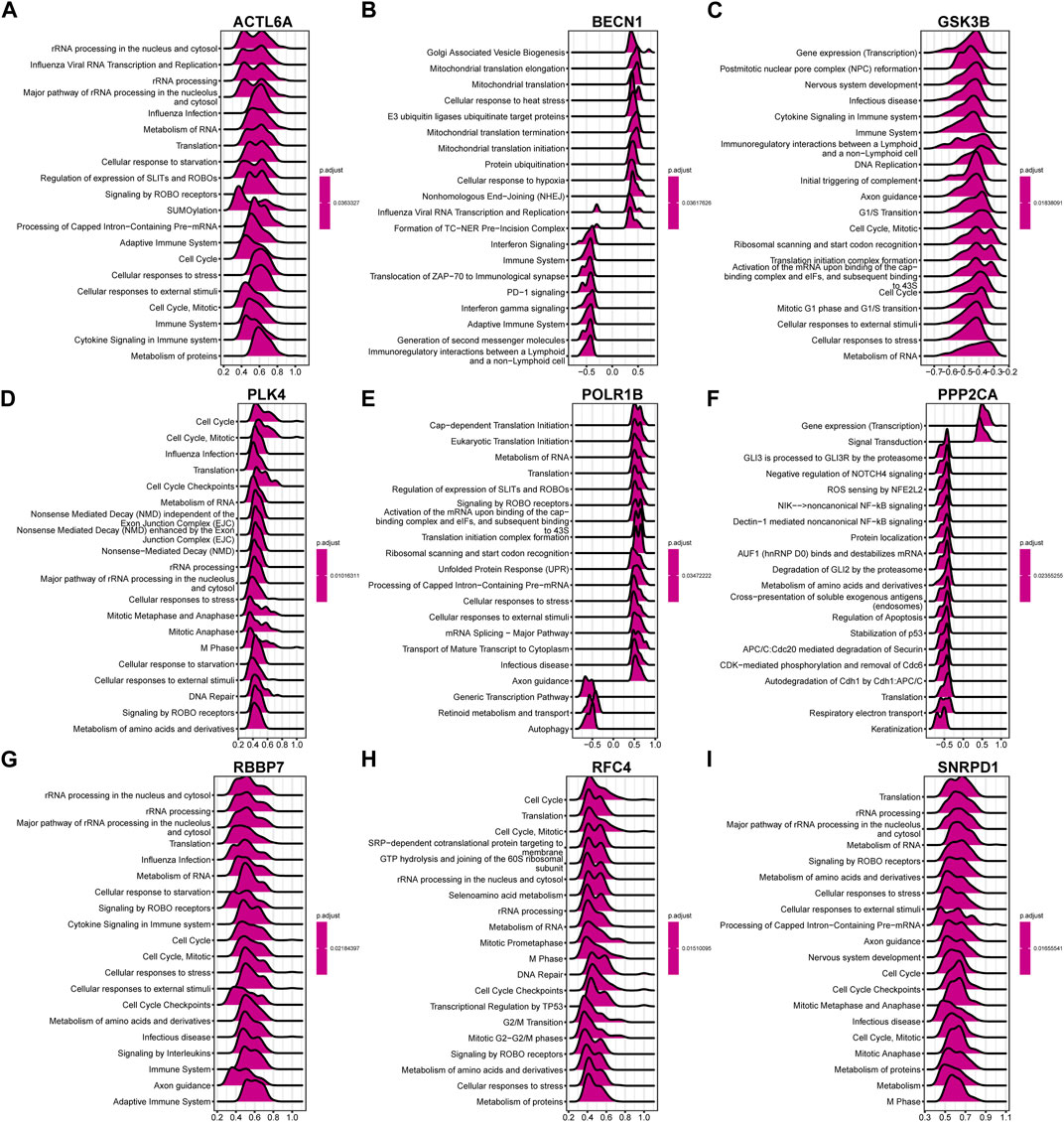
Figure 14. The ridge plot of gene set enrichment analysis (GSEA) against the Reactome pathways (top 20) for the 9 hub genes. Significant GSEA results of the top 50 genes most positively or negatively associated with (A) ACTL6A, (B) BECN1, (C) GSK3B, (D) PLK4, (E) POLR1B, (F) PPP2CA, (G) RBBP7, (H) RFC4 and (I) SNRPD1, respectively.
Discussion
Up to now, RIF is still not well understood and is an unsolved issue in the field of assisted reproduction, causing significant mental stress, economic strain for families, also several societal issues. Thus, it is vital to examine the potential genetic basis of aberrant endometrial gene expression profiles in patients with RIF to identify better and more accurate biomarkers for diagnosis and treatment.
First, we identified DEGs in the endometrium during the implantation period between RIF and healthy fertile control, which yielded 8 upregulated and 20 downregulated overlapping genes with stable differences in the three-chip datasets. In the further functional exploration of these DEGs, the GO-BP analysis revealed that mitotic cell cycle phase transition, negative control of transport, control of intracellular transport, post−translational protein modification, nucleocytoplasmic transport, and nuclear transport were significantly enriched, which are closely related to the maintenance of body homeostasis. Maintaining cellular homeostasis in the endometrium under oxidative stress is thought to be essential for pregnancy (Qin et al., 2016). A recent study found that downregulation of Sirtuin1 (SIRT1) disrupted the intracellular reactive oxygen species (ROS) homeostasis during the decidualization of human endometrial stromal cells (ESCs) in RIF patients (Li et al., 2021). Although there is no report on the relationship between the above functions and RIF, any imbalance in cellular and molecular endometrial homeostasis may lead to reproductive diseases (Kolanska et al., 2021), raising the possibility that they may cause embryo implantation failure in RIF. This warrants clarification with further studies.
Moreover, the enrichment of KEGG pathways for prevalent DEGs demonstrates that Herpes simplex virus 1 infection and Salmonella infection could contribute significantly to RIF. Throughout the human body, microbial communities assemble into distinguishing and stable ecological systems, usually referred to as the “another human genome,” which participates in the internal environment and homeostasis of human body. The female reproductive tract contains different bacterial communities, forming a continuous microbial population from the vagina to the ovary (Chen et al., 2017). It has been shown previously that the abundance of Lactobacillus in the vagina has been positively associated with pregnancy outcomes. Compared to individuals who attained clinical pregnancy during the first frozen embryo transfer cycle, patients with unexplained RIF exhibited significantly reduced Lactobacillus spp in vaginal canals, and the α-diversity of microbial communities from unexplained RIF was higher than control samples. Moreover, altered Lactobacillus-dominated endometrial microbiota has been reported to cause endometritis (Moreno et al., 2016), and some studies suggested an association of chronic endometritis (CE) with miscarriage and implantation failure (Cicinelli et al., 2015). A prospective cohort study (n = 241) reported that the prevalence of CE in the RIF population was up to 33.7% (Kitaya et al., 2017). In combination with the findings of present investigation, we conclude that an imbalance of Lactobacillus-dominated endometrial microbiota can contribute to implantation failure.
Traditional DEG-based screening methods have the disadvantage of locally exploring the dataset, which is most likely to lack master molecules. Encouragingly, WGCNA can effectively detect co-expression modules and genes in the biological system as a whole, which has been widely utilized to discover potential biomarkers or treatment targets. The current result revealed that infection with Herpes simplex virus 1, Amyotrophic lateral sclerosis, and the Hippo signaling pathway were significantly associated with genes in the brown and grey modules, while the genes in the two above modules were a significant negative association with RIF. The Hippo signaling pathway is a signaling mechanism with high conservation that regulates endometrial physiology in a substantial way (Zhu et al., 2017). Previous research uncovered that the upregulation of LATS1 and MOB kinase activator 1A is implicated in RIF patients’ Hippo signaling pathway (Bastu et al., 2019). However, there is no research on the genes related to amyotrophic lateral sclerosis. We hypothesized that dysregulated genes contribute to the progression of RIF via these potential pathways.
According to genes that exhibited a strong association with RIF recognized via WGCNA, the intersected genes with the previous DEGs were obtained with both variance and association. Notably, the nine hub genes listed below were ultimately chosen: ACTL6A, BECN1, SNRPD1, POLR1B, GSK3B, PPP2CA, RBBP7, PLK4, and RFC4, based on the PPI network and three algorithms (“Closeness,” “Radiality,” and “Stress”). The levels of expression of the nine hub genes were significantly varied between RIF versus control tissues. Particularly, the expressions of BECN1 and GSK3B were significantly elevated, whereas ACTL6A, POLR1B, SNRPD1, PPP2CA, PLK4, RBBP7, and RFC4 exhibited remarkably decreased expression levels in RIF tissues, which were also verified by RT-qPCR in our independent samples containing RIF patients and control. Nevertheless, the diagnostic effectiveness of aforementioned genes for RIF was low. Based on ROC analysis, suggesting on this hand that RIF is a clinical difficulty with heterogeneous etiologic factors and complicated pathogenesis. On the other hand, its diagnosis mainly depends on the special clinical manifestations, and it is currently hard to predict by genetic testing.
BECN1 and LC3B expressions are widely used as autophagic markers. A previous study found that autophagy is drastically enhanced in RIF endometrial tissues and could be implicated in the pathogenesis of RIF(Zhu et al., 2022). Similarly, our result showed that endometrial BECN1 is upregulated in the RIF patient compared to normal endometrium. Mehdinejadiani, S. et al. discovered that the GSK3B abnormal expression was negatively impacted in the endometrium of women stimulated by clomiphene citrate in comparison with letrozole in polycystic ovary syndrome, primarily liable for the thin endometrium(Mehdinejadiani et al., 2019). This result was consistent with our study, which demonstrated that GSK3B had considerably enhanced expression in RIF. Besides, SNRPD1, one of the key genes encoding core spliceosome constituents, and its elevated protein expression in somatic cells was associated with kidney injury and pulmonary hypertension in systemic lupus erythematosus (SLE) patients (Hu et al., 2017). It has been reported that SNRPD1 could be an oncogene that affects the development of hepatocellular carcinoma by controlling the mTOR pathway and autophagy (Wang et al., 2022). Moreover, PLK4, an essential member of the polo-like serine-threonine kinase family, is required for centriole duplication regulation. The aberrant expression of PLK4 resulted in tripolar mitosis and aneuploidy in human preimplantation embryos [25], which led to RIF. ACTL6A is an ATP-dependent SWI/SNF regulatory complex protein with chromatin-remodeling components (Mani et al., 2017). Recent studies revealed that follicle-stimulating hormone (FSH)-stimulated glycolysis in ovarian malignancy comprised a higher level of the poor prognostic factor ACTL6A, which forecasted metastasis and prognosis of ovarian cancer patients (Chen et al., 2023). POLR1B, which encodes DNA-directed RNA polymerase I subunit RPA2, has been linked to Treacher Collins and may be involved in cluster headaches (Harder et al., 2021). PP2A catalytic subunit (PP2Aca) was encoded by the PPP2CA gene. A recent investigation revealed that PPP2CA variant alleles are significantly correlated with susceptibility to SLE (Zhang et al., 2018). RBBP7 is a key element of several complexes for chromatin remodeling and histone modification, that is upregulated in numerous types of cancer and plays contradictory roles in tumors development (Wang et al., 2022). The RFC4 gene, which encodes the fourth biggest subunit of RFC complex, has been found to be dysregulated in a variety of cancers, including head-and-neck squamous cell, hepatocellular, colonic, prostate, and cervical carcinomas (van Dam et al., 2018). However, little relevant studies have been performed to decipher the role of above core genes in endometrial implantation. Hypothetically speaking, key genes mentioned above might be involved in the RIF through impairing autophagy and disturbing proliferation of endometrium, which needs to be further explored.
Embryo quality, embryo endometrium interaction, and endometrial receptivity are essential for successful implantation, and about two-thirds of implantation failures are attributable to insufficient endometrial receptivity (Koler et al., 2009). In particular, well-balanced immune status in the endometrium has been documented as an important determinant of endometrial receptivity. Benefiting from the edge of CIBERSORT to conduct a thorough evaluation of immune cell infiltration, the current study revealed that CD4+ T cells and NK cells were the significantly altered cell types in the RIF endometrium. Around the period of embryo implantation, the maternal immune system undergoes significant immunological changes, including enrichment in the different immune cells in peripheral circulation and the uterine microenvironment. These modifications provide an immunologically tolerant environment protecting embryos expressing paternal antigen from maternal antigen-specific T cells and promote successful implantation (Ledee et al., 2016). During embryo implantation, the maternal immune system is comprised of a unique imbalance of subtypes of T lymphocytes (CD4+ T cells and killer T cells) which are emerging as a prevalent cause of infertility (Lee et al., 2011). An increasing number of studies showed that in RIF, dysregulation of involved immune cells, including uterine NK (uNK), regulatory T (Tregs), and T-helper cells, have been identified [35-37]. While they interacted during the maternal hemodynamic response to embryo implantation, the changed presence of T cells is also related to lower uNK effectiveness in decidual vessel remodeling (Kieckbusch et al., 2014). Throughout trophoblast invasion, abnormal uNK activity may result in unfavorable effects, including vascular remodeling, local ischemia, and oxidative stress, which are damaging to implantation (Donoghue et al., 2019). Moreover, one report suggested that RIF patients with CE exhibited a substantial overexpression of uterine CD68(+) macrophages, CD83(+) mature dendritic cells, CD8(+) T cells, and Foxp3(+) regulatory T cells, which may contribute to decreased endometrial receptivity and repeated pregnancy failures (Li et al., 2020). Compared to healthy controls, the fraction of CD56+ uNK was significantly higher in individuals with RIF, indicating that the intrauterine immunological state of patients with RIF has altered (Von Woon et al., 2022). Consequently, the current findings are in line with earlier reports and demonstrate the significance of these immune cells in RIF pathogenesis, whereas the accurate molecular mechanism needs further research.
Considering the significance of immune infiltration cells and hub genes in RIF, more research reveals that the role of six hub genes (BECN1, GSK3B, PPP2CA, RBBP7, RFC4, and SNRPD1) was considerably associated with immune cells. For example, both BECN1 and PPP2CA exhibited a positive correlation with macrophage M0 and a negative correlation with macrophage M2. The BECN1 can promote the autophagy process of macrophages and regulate the immune response of macrophages. A recent study has reported that PPP2CA downregulation improved NF-κB signaling and stimulated macrophage expression of IL-1b, IL-6, and TNF-α (Guo et al., 2020). The outcomes of correlation analysis revealed that hub genes were primarily enhanced in macrophages, eosinophils, B cells, monocytes, and T cells regulatory Tregs, suggesting that the above hub genes may have a function in the incidence and progression of RIF through controlling corresponding immune cells; this hypothesis should be validated in future studies. Last but not least, according to our understanding, this work describes a previously unreported approach through which the ridge plot can display a clear and crucial association between hub genes and other genes and key pathways. This may be a direction for the next study of RIF mechanism.
Compared to previous studies (Wang and Liu, 2020; Dong et al., 2023; Lai et al., 2023), we first used three comprehensive microarray datasets GSE58144 (Koot et al., 2016), GSE103465 (Guo et al., 2018), and GSE111974 (Bastu et al., 2019) comprising 99 RIF and 70 normal endometrial tissues, and both enrichment analysis and WGCNA were conducted to distinguish hub genes associated with RIF. The WGCNA has made the greatest contribution to finding association patterns among genes across samples and interpreting the direct biological role of gene network modules, which makes our results more effective and reliable. In particular, we have validated this study’s results through RT-qPCR in our 30 independent samples. However, some limitations should be acknowledged in the current study. First, since the GEO datasets were exploited retrospectively, no additional clinical information about the patients is available, which could cause some deviations in the analysis of our results. Second, the three datasets come from multiple, heterogeneous studies with different populations, these discrepancies might be related to ethnic differences. Third, although the RNA expression patterns of nine key genes were validated in the present study through laboratory experiments, we did not evaluate their expression profiles on the protein level. Last but not least, exploring the expression profiles of the hub genes in previously published single-cell endometrial datasets could unveil specific cell types or state that play a role in the pathogenesis of RIF. We will collect more endometrial samples and single-cell endometrial datasets for more in-depth study in the future.
Conclusion
In conclusion, we first utilized WGCNA to identify the most potential hub genes (ACTL6A, BECN1, SNRPD1, POLR1B, GSK3B, PPP2CA, RBBP7, PLK4, and RFC4), which might be associated with RIF. Meanwhile, this study offers insights into the landscape of immune infiltration status to reveal the underlying immune pathogenesis of RIF. This may be a direction for the next study of RIF etiology. Further studies would be required to investigate the involved mechanisms.
Data availability statement
The datasets presented in this study can be found in online repositories. The names of the repository/repositories and accession number(s) can be found in the article/Supplementary Material.
Ethics statement
The studies involving humans were approved by The Ethical Committee of Yantai Yuhuangding Hospital. The studies were conducted in accordance with the local legislation and institutional requirements. The participants provided their written informed consent to participate in this study. Written informed consent was obtained from the individual(s) for the publication of any potentially identifiable images or data included in this article.
Author contributions
ZL: Conceptualization, Data curation, Writing–original draft, Writing–review and editing. SL: Data curation, Validation, Writing–original draft, Writing–review and editing. QQ: Conceptualization, Formal Analysis, Writing–original draft, Writing–review and editing. XL: Funding acquisition, Methodology, Writing–review and editing. WZ: Formal Analysis, Writing–original draft. DZ: Data curation, Writing–review and editing. SH: Validation, Writing–review and editing. YS: Investigation, Project administration, Supervision, Writing–review and editing. HB: Investigation, Project administration, Supervision, Writing–original draft, Writing–review and editing.
Funding
The author(s) declare that financial support was received for the research, authorship, and/or publication of this article. This work was supported by Natural Science Foundation of Shandong Province (ZR2021MH012) and Yantai Science and Technology Planning Project (2023YD014).
Acknowledgments
We would like to extend our deepest thanks to the editors and all reviewers for their hard work.
Conflict of interest
The authors declare that the research was conducted in the absence of any commercial or financial relationships that could be construed as a potential conflict of interest.
Publisher’s note
All claims expressed in this article are solely those of the authors and do not necessarily represent those of their affiliated organizations, or those of the publisher, the editors and the reviewers. Any product that may be evaluated in this article, or claim that may be made by its manufacturer, is not guaranteed or endorsed by the publisher.
Supplementary material
The Supplementary Material for this article can be found online at: https://www.frontiersin.org/articles/10.3389/fgene.2024.1292757/full#supplementary-material
References
Ahmadi, M., Pashangzadeh, S., Moraghebi, M., Sabetian, S., Shekari, M., Eini, F., et al. (2022). Construction of circRNA-miRNA-mRNA network in the pathogenesis of recurrent implantation failure using integrated bioinformatics study. J. Cell Mol. Med. 26 (6), 1853–1864. doi:10.1111/jcmm.16586
Bastu, E., Demiral, I., Gunel, T., Ulgen, E., Gumusoglu, E., Hosseini, M. K., et al. (2019). Potential marker pathways in the endometrium that may cause recurrent implantation failure. Reprod. Sci. 26 (7), 879–890. doi:10.1177/1933719118792104
Busnelli, A., Reschini, M., Cardellicchio, L., Vegetti, W., Somigliana, E., and Vercellini, P. (2020). How common is real repeated implantation failure? An indirect estimate of the prevalence. Reprod. Biomed. Online 40 (1), 91–97. doi:10.1016/j.rbmo.2019.10.014
Chen, C., Song, X., Wei, W., Zhong, H., Dai, J., Lan, Z., et al. (2017). The microbiota continuum along the female reproductive tract and its relation to uterine-related diseases. Nat. Commun. 8 (1), 875. doi:10.1038/s41467-017-00901-0
Chen, P. M., Wong, C. N., Wong, C. N., and Chu, P. Y. (2023). Actin-like protein 6A expression correlates with cancer stem cell-like features and poor prognosis in ovarian cancer. Int. J. Mol. Sci. 24 (3), 2016. doi:10.3390/ijms24032016
Cicinelli, E., Matteo, M., Tinelli, R., Lepera, A., Alfonso, R., Indraccolo, U., et al. (2015). Prevalence of chronic endometritis in repeated unexplained implantation failure and the IVF success rate after antibiotic therapy. Hum. Reprod. 30 (2), 323–330. doi:10.1093/humrep/deu292
Coughlan, C., Ledger, W., Wang, Q., Liu, F., Demirol, A., Gurgan, T., et al. (2014). Recurrent implantation failure: definition and management. Reprod. Biomed. Online 28 (1), 14–38. doi:10.1016/j.rbmo.2013.08.011
Dong, X., Zhou, M., Li, X., Huang, H., and Sun, Y. (2023). Gene profiling reveals the role of inflammation, abnormal uterine muscle contraction and vascularity in recurrent implantation failure. Front. Genet. 14, 1108805. doi:10.3389/fgene.2023.1108805
Donoghue, J. F., Paiva, P., Teh, W. T., Cann, L. M., Nowell, C., Rees, H., et al. (2019). Endometrial uNK cell counts do not predict successful implantation in an IVF population. Hum. Reprod. 34 (12), 2456–2466. doi:10.1093/humrep/dez194
Feng, C., Shen, J. M., Lv, P. P., Jin, M., Wang, L. Q., Rao, J. P., et al. (2018). Construction of implantation failure related lncRNA-mRNA network and identification of lncRNA biomarkers for predicting endometrial receptivity. Int. J. Biol. Sci. 14 (10), 1361–1377. doi:10.7150/ijbs.25081
Guo, F., Si, C., Zhou, M., Wang, J., Zhang, D., Leung, P. C. K., et al. (2018). Decreased PECAM1-mediated TGF-β1 expression in the mid-secretory endometrium in women with recurrent implantation failure. Hum. Reprod. 33 (5), 832–843. doi:10.1093/humrep/dey022
Guo, J., Duan, Z., Zhang, C., Wang, W., He, H., Liu, Y., et al. (2020). Mouse 4T1 breast cancer cell-derived exosomes induce proinflammatory cytokine production in macrophages via miR-183. J. Immunol. 205 (10), 2916–2925. doi:10.4049/jimmunol.1901104
Harder, A. V. E., Winsvold, B. S., Noordam, R., Vijfhuizen, L. S., Borte, S., Kogelman, L. J. A., et al. (2021). Genetic susceptibility loci in genomewide association study of cluster headache. Ann. Neurol. 90 (2), 203–216. doi:10.1002/ana.26146
Hu, C., Li, M., Liu, J., Qian, J., Xu, D., Zhang, S., et al. (2017). Anti-SmD1 antibodies are associated with renal disorder, seizures, and pulmonary arterial hypertension in Chinese patients with active SLE. Sci. Rep. 7 (1), 7617. doi:10.1038/s41598-017-08099-3
Huang, C., Jiang, Y., Zhou, J., Yan, Q., Jiang, R., Cheng, X., et al. (2017). Increased Kruppel-like factor 12 in recurrent implantation failure impairs endometrial decidualization by repressing Nur77 expression. Reprod. Biol. Endocrinol. 15 (1), 25. doi:10.1186/s12958-017-0243-8
Kieckbusch, J., Gaynor, L. M., Moffett, A., and Colucci, F. (2014). MHC-dependent inhibition of uterine NK cells impedes fetal growth and decidual vascular remodelling. Nat. Commun. 5, 3359. doi:10.1038/ncomms4359
Kitaya, K., Matsubayashi, H., Takaya, Y., Nishiyama, R., Yamaguchi, K., Takeuchi, T., et al. (2017). Live birth rate following oral antibiotic treatment for chronic endometritis in infertile women with repeated implantation failure. Am. J. Reprod. Immunol. 78 (5), 12719. doi:10.1111/aji.12719
Kolanska, K., Bendifallah, S., Canlorbe, G., Mekinian, A., Touboul, C., Aractingi, S., et al. (2021). Role of miRNAs in normal endometrium and in endometrial disorders: comprehensive review. J. Clin. Med. 10 (16), 3457. doi:10.3390/jcm10163457
Koler, M., Achache, H., Tsafrir, A., Smith, Y., Revel, A., and Reich, R. (2009). Disrupted gene pattern in patients with repeated in vitro fertilization (IVF) failure. Hum. Reprod. 24 (10), 2541–2548. doi:10.1093/humrep/dep193
Koot, Y. E., van Hooff, S. R., Boomsma, C. M., van Leenen, D., Groot Koerkamp, M. J., Goddijn, M., et al. (2016). An endometrial gene expression signature accurately predicts recurrent implantation failure after IVF. Sci. Rep. 6, 19411. doi:10.1038/srep19411
Lai, Z. Z., Zhang, J., Zhou, W. J., Shi, J. W., Yang, H. L., Yang, S. L., et al. (2023). Identification of potential biomarkers and immune infiltration characteristics in recurrent implantation failure using bioinformatics analysis. Front. Immunol. 14, 992765. doi:10.3389/fimmu.2023.992765
Langfelder, P., and Horvath, S. (2008). WGCNA: an R package for weighted correlation network analysis. BMC Bioinforma. 9, 559. doi:10.1186/1471-2105-9-559
Ledee, N., Petitbarat, M., Chevrier, L., Vitoux, D., Vezmar, K., Rahmati, M., et al. (2016). The uterine immune profile may help women with repeated unexplained embryo implantation failure after in vitro fertilization. Am. J. Reprod. Immunol. 75 (3), 388–401. doi:10.1111/aji.12483
Lee, S. K., Kim, J. Y., Hur, S. E., Kim, C. J., Na, B. J., Lee, M., et al. (2011). An imbalance in interleukin-17-producing T and Foxp3⁺ regulatory T cells in women with idiopathic recurrent pregnancy loss. Hum. Reprod. 26 (11), 2964–2971. doi:10.1093/humrep/der301
Li, J., Qi, J., Yao, G., Zhu, Q., Li, X., Xu, R., et al. (2021). Deficiency of Sirtuin 1 impedes endometrial decidualization in recurrent implantation failure patients. Front. Cell Dev. Biol. 9, 598364. doi:10.3389/fcell.2021.598364
Li, Y., Yu, S., Huang, C., Lian, R., Chen, C., Liu, S., et al. (2020). Evaluation of peripheral and uterine immune status of chronic endometritis in patients with recurrent reproductive failure. Fertil. Steril. 113 (1), 187–196. doi:10.1016/j.fertnstert.2019.09.001
Mani, U., S, A. S., Goutham, R. N. A., and Mohan, S. S. (2017). SWI/SNF Infobase-An exclusive information portal for SWI/SNF remodeling complex subunits. PLoS One 12 (9), e0184445. doi:10.1371/journal.pone.0184445
Mehdinejadiani, S., Amidi, F., Mehdizadeh, M., Barati, M., Pazhohan, A., Alyasin, A., et al. (2019). Effects of letrozole and clomiphene citrate on Wnt signaling pathway in endometrium of polycystic ovarian syndrome and healthy women†. Biol. Reprod. 100 (3), 641–648. doi:10.1093/biolre/ioy187
Moreno, I., Codoner, F. M., Vilella, F., Valbuena, D., Martinez-Blanch, J. F., Jimenez-Almazan, J., et al. (2016). Evidence that the endometrial microbiota has an effect on implantation success or failure. Am. J. Obstet. Gynecol. 215 (6), 684–703. doi:10.1016/j.ajog.2016.09.075
Qin, A., Qin, J., Jin, Y., Xie, W., Fan, L., Jiang, L., et al. (2016). DHEA improves the antioxidant capacity of endometrial stromal cells and improves endometrium receptivity via androgen receptor. Eur. J. Obstet. Gynecol. Reprod. Biol. 198, 120–126. doi:10.1016/j.ejogrb.2016.01.016
van Dam, P. A., Rolfo, C., Ruiz, R., Pauwels, P., Van Berckelaer, C., Trinh, X. B., et al. (2018). Potential new biomarkers for squamous carcinoma of the uterine cervix. ESMO Open 3 (4), e000352. doi:10.1136/esmoopen-2018-000352
Von Woon, E., Greer, O., Shah, N., Nikolaou, D., Johnson, M., and Male, V. (2022). Number and function of uterine natural killer cells in recurrent miscarriage and implantation failure: a systematic review and meta-analysis. Hum. Reprod. Update 28 (4), 548–582. doi:10.1093/humupd/dmac006
Wang, F., and Liu, Y. (2020). Identification of key genes, regulatory factors, and drug target genes of recurrent implantation failure (RIF). Gynecol. Endocrinol. 36 (5), 448–455. doi:10.1080/09513590.2019.1680622
Wang, H., Xu, F., Lu, L., Yang, F., Huang, X., Lv, L., et al. (2022a). The diagnostic and prognostic significance of small nuclear ribonucleoprotein Sm D1 aberrantly high expression in hepatocellular carcinoma. J. Cancer 13 (1), 184–201. doi:10.7150/jca.65225
Wang, R., Huang, Z., Lin, Z., Chen, B., Pang, X., Du, C., et al. (2022b). Hypoxia-induced RBBP7 promotes esophagus cancer progression by inducing CDK4 expression. Acta Biochim. Biophys. Sin. (Shanghai) 54 (2), 179–186. doi:10.3724/abbs.2021027
Yuan, Y., Chen, J., Wang, J., Xu, M., Zhang, Y., Sun, P., et al. (2020). Identification hub genes in colorectal cancer by integrating weighted gene Co-expression network analysis and clinical validation in vivo and vitro. Front. Oncol. 10, 638. doi:10.3389/fonc.2020.00638
Zhang, J., Meng, Y., Wu, H., Wu, Y., Yang, B., and Wang, L. (2018). Association between PPP2CA polymorphisms and clinical features in southwest Chinese systemic lupus erythematosus patients. Med. Baltim. 97 (27), e11451. doi:10.1097/MD.0000000000011451
Zhou, Q., Yan, G., Ding, L., Liu, J., Yu, X., Kong, S., et al. (2019). EHD1 impairs decidualization by regulating the Wnt4/β-catenin signaling pathway in recurrent implantation failure. EBioMedicine 50, 343–354. doi:10.1016/j.ebiom.2019.10.018
Zhu, H. Y., Ge, T. X., Pan, Y. B., and Zhang, S. Y. (2017). Advanced role of Hippo signaling in endometrial fibrosis: implications for intrauterine adhesion. Chin. Med. J. Engl. 130 (22), 2732–2737. doi:10.4103/0366-6999.218013
Keywords: recurrent implantation failure, hub genes, immune cell infiltration, bioinformatics, infertility
Citation: Liu Z, Lai S, Qu Q, Liu X, Zhang W, Zhao D, He S, Sun Y and Bao H (2024) Analysis of weighted gene co-expression networks and clinical validation identify hub genes and immune cell infiltration in the endometrial cells of patients with recurrent implantation failure. Front. Genet. 15:1292757. doi: 10.3389/fgene.2024.1292757
Received: 14 September 2023; Accepted: 22 March 2024;
Published: 05 April 2024.
Edited by:
Petar Ozretić, Rudjer Boskovic Institute, CroatiaReviewed by:
Zhen-Gao Sun, Affiliated Hospital of Shandong University of Traditional Chinese Medicine, ChinaTanvi Potluri, Northwestern Medicine, United States
Copyright © 2024 Liu, Lai, Qu, Liu, Zhang, Zhao, He, Sun and Bao. This is an open-access article distributed under the terms of the Creative Commons Attribution License (CC BY). The use, distribution or reproduction in other forums is permitted, provided the original author(s) and the copyright owner(s) are credited and that the original publication in this journal is cited, in accordance with accepted academic practice. No use, distribution or reproduction is permitted which does not comply with these terms.
*Correspondence: Yuxia Sun, 1092147497@qq.com; Hongchu Bao, hongchubao@outlook.com
†These authors have contributed equally to this work and share first authorship